Delete: Deep Lead Optimization Enveloped in Protein Pocket through Unified Deleting Strategies and a Structure-aware Network
arXiv (Cornell University)(2023)
摘要
Drug discovery is a highly complicated process, and it is unfeasible to fully commit it to the recently developed molecular generation methods. Deep learning-based lead optimization takes expert knowledge as a starting point, learning from numerous historical cases about how to modify the structure for better drug-forming properties. However, compared with the more established de novo generation schemes, lead optimization is still an area that requires further exploration. Previously developed models are often limited to resolving one (or few) certain subtask(s) of lead optimization, and most of them can only generate the two-dimensional structures of molecules while disregarding the vital protein-ligand interactions based on the three-dimensional binding poses. To address these challenges, we present a novel tool for lead optimization, named Delete (Deep lead optimization enveloped in protein pocket). Our model can handle all subtasks of lead optimization involving fragment growing, linking, and replacement through a unified deleting (masking) strategy, and is aware of the intricate pocket-ligand interactions through the geometric design of networks. Statistical evaluations and case studies conducted on individual subtasks demonstrate that Delete has a significant ability to produce molecules with superior binding affinities to protein targets and reasonable drug-likeness from given fragments or atoms. This feature may assist medicinal chemists in developing not only me-too/me-better products from existing drugs but also hit-to-lead for first-in-class drugs in a highly efficient manner.
更多查看译文
关键词
deep lead optimization,protein pocket,unified deleting strategies,structure-aware
AI 理解论文
溯源树
样例
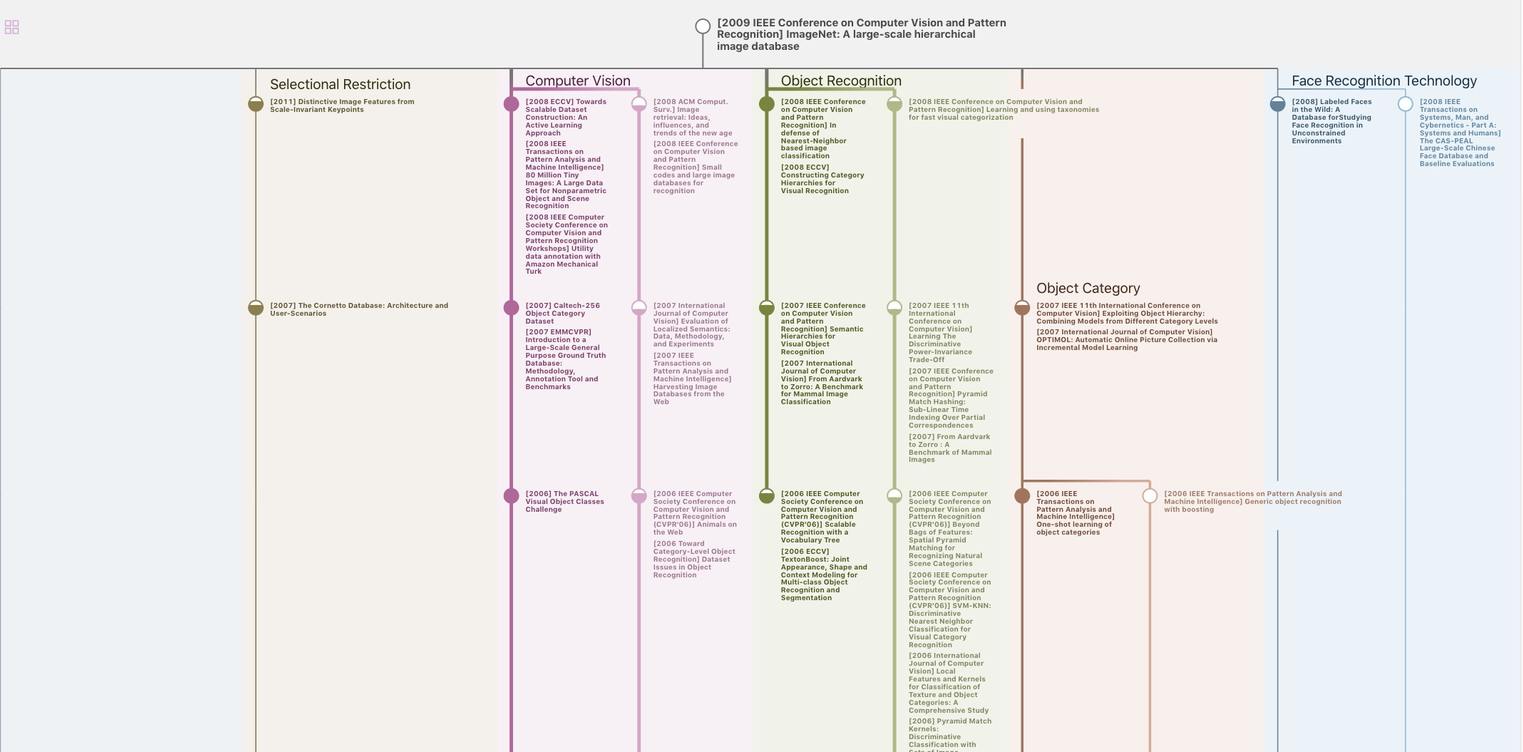
生成溯源树,研究论文发展脉络
Chat Paper
正在生成论文摘要