Transferable Graph Neural Fingerprint Models for Quick Response to Future Bio-Threats
2023 International Conference on Machine Learning and Applications (ICMLA)(2023)
摘要
Fast screening of drug molecules based on the ligand binding affinity is an important step in the drug discovery pipeline. Graph neural fingerprint is a promising method for developing molecular docking surrogates with high throughput and great fidelity. In this study, we built a COVID-19 drug docking dataset of about 300,000 drug candidates on 23 coronavirus protein targets. With this dataset, we trained graph neural fingerprint docking models for high-throughput virtual COVID-19 drug screening. The graph neural fingerprint models yield high prediction accuracy on docking scores with the mean squared error lower than $0.21$ kcal/mol for most of the docking targets, showing significant improvement over conventional circular fingerprint methods. To make the neural fingerprints transferable for unknown targets, we also propose a transferable graph neural fingerprint method trained on multiple targets. With comparable accuracy to target-specific graph neural fingerprint models, the transferable model exhibits superb training and data efficiency. We highlight that the impact of this study extends beyond COVID-19 dataset, as our approach for fast virtual ligand screening can be easily adapted and integrated into a general machine learning-accelerated pipeline to battle future bio-threats.
更多查看译文
关键词
graph,bio-threats
AI 理解论文
溯源树
样例
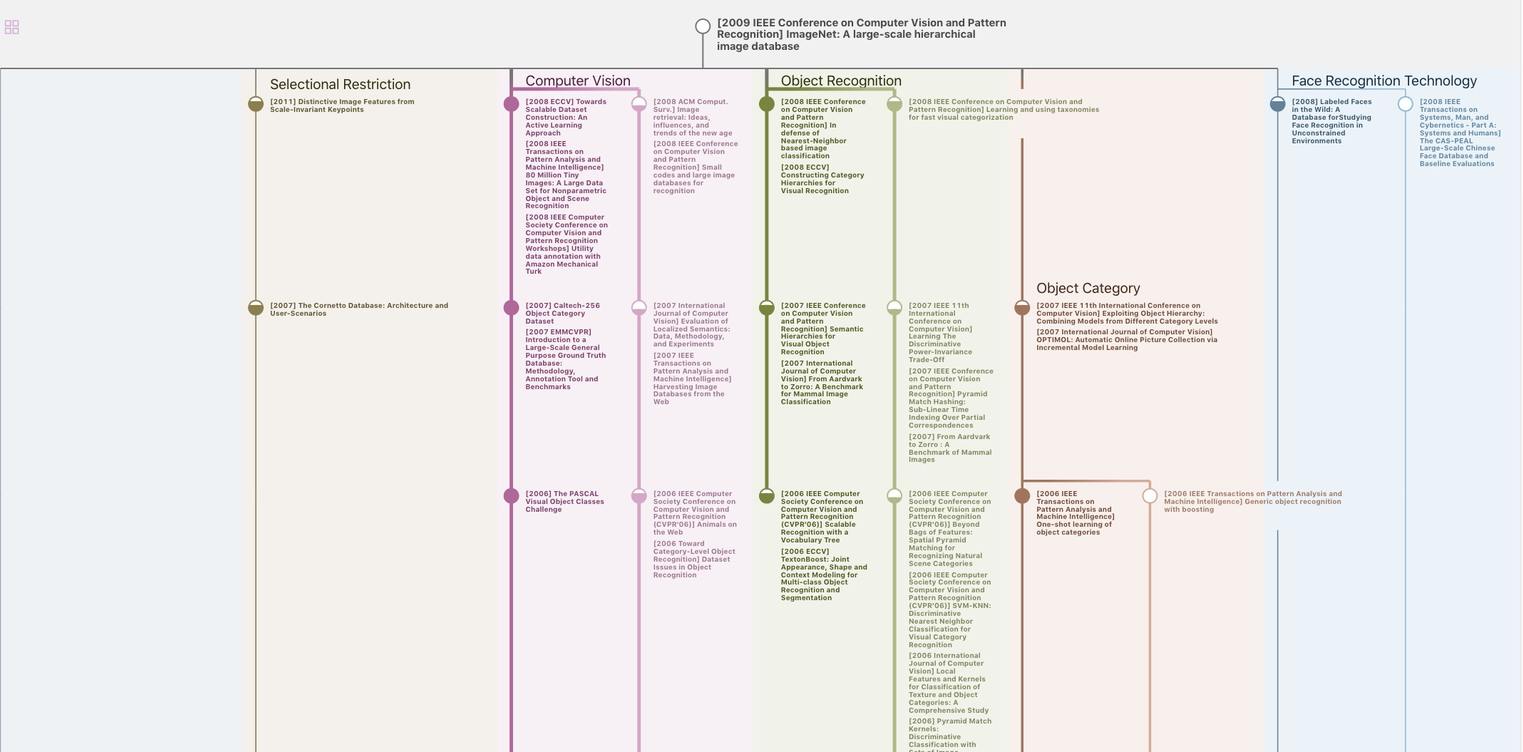
生成溯源树,研究论文发展脉络
Chat Paper
正在生成论文摘要