A Self-organized Maps Ground Extract Method based on Principal Component Analysis
2023 IEEE/ASME INTERNATIONAL CONFERENCE ON ADVANCED INTELLIGENT MECHATRONICS, AIM(2023)
Abstract
The lightweight of point clouds is an essential issue for LiDAR in practical applications. Point clouds collected outdoors often have a large number of ground points, reducing the data processing speed and affecting the classification and identification of targets. The paper develops a ground extraction method based on principal component analysis (PCA) and self-organizing map (SOM). The sufficient information is selected by analyzing the original point cloud features to improve the statistical outlier removal filter to achieve the initial cleaning of the point cloud. The filtered point cloud is reduced dimension by PCA, and overcomes the feature classification difficulty while accelerating the subsequent point cloud processing. Furthermore, SOM achieves unsupervised learning for the practical point cloud, which performs efficient ground extraction at sparse and dense locations while not relying on the size of the dataset. Experiments on SemanticKitti show that the detection accuracy of the proposed method can reach 95%, and it also has the satisfactory real-time performance.
MoreTranslated text
Key words
point cloud,ground separation,filter,principal component analysis,self-organizing map
AI Read Science
Must-Reading Tree
Example
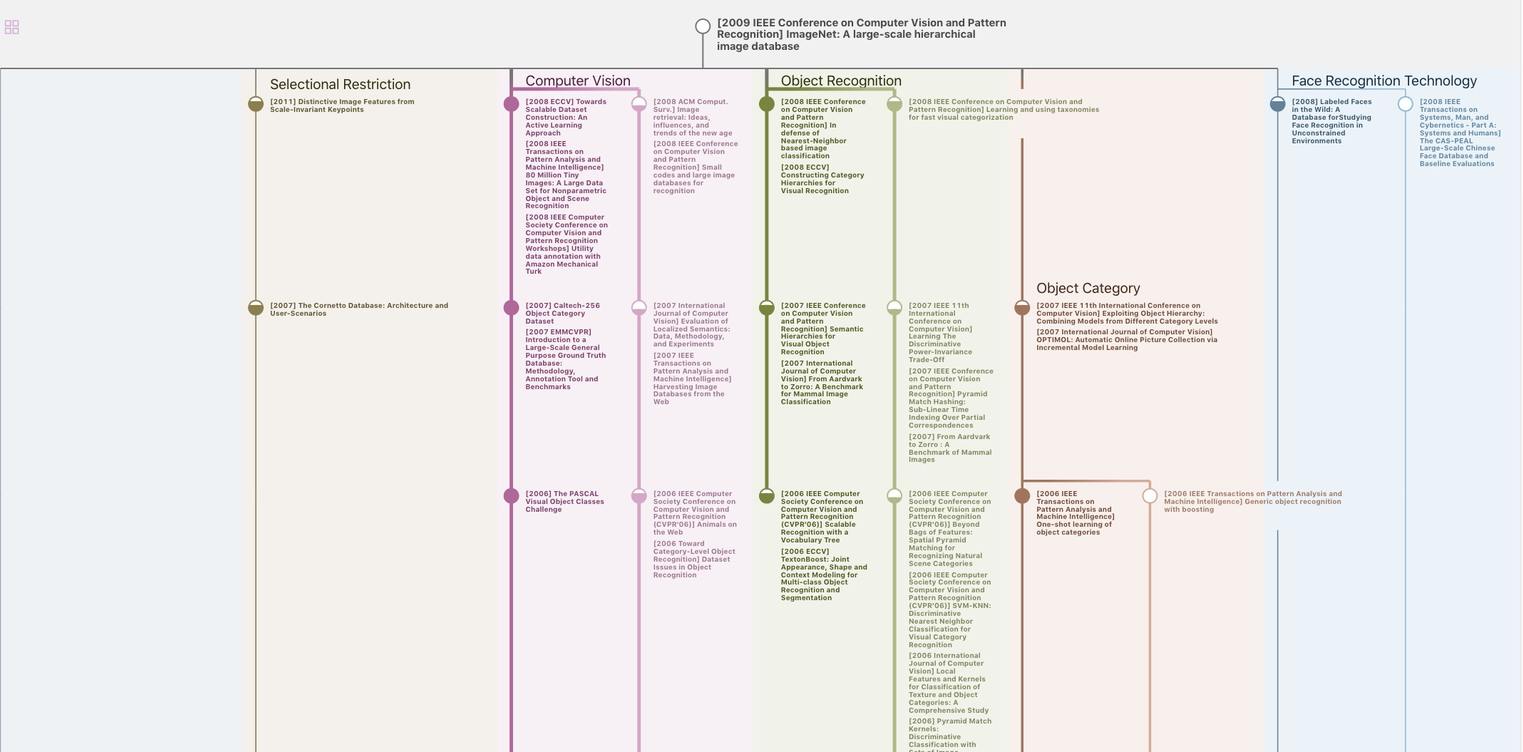
Generate MRT to find the research sequence of this paper
Chat Paper
Summary is being generated by the instructions you defined