A generalized AI method for pathology cancer diagnosis and prognosis prediction based on transfer learning and hierarchical split.
Physics in medicine and biology(2023)
摘要
Objective.This study aims to propose a generalized AI method for pathology cancer diagnosis and prognosis prediction based on transfer learning and hierarchical split.Approach.We present a neural network framework for cancer diagnosis and prognosis prediction in pathological images. To enhance the network's depth and width, we employ a hierarchical split block (HS-Block) to create an AI-aided diagnosis system suitable for semi-supervised clinical settings with limited labeled samples and cross-domain tasks. By incorporating a lightweight convolution unit based on the HS-Block, we improve the feature information extraction capabilities of a regular network (RegNet). Additionally, we integrate a Convolutional Block Attention Module into the first and last convolutions to optimize the extraction of global features and local details. To address limited sample labels, we employ a dual-transfer learning (DTL) mechanism named DTL-HS-Regnet, enabling semi-supervised learning in clinical settings.Main results.Our proposed DTL-HS-Regnet model outperforms other advanced deep-learning models in three different types of cancer diagnosis tasks. It demonstrates superior feature extraction ability, achieving an average sensitivity, specificity, accuracy, and F1 score of 0.9987, 1.0000, 1.0000 and 0.9992, respectively. Furthermore, we evaluate the model's capability to directly extract prognosis prediction information from pathological images by constructing patient cohorts. The results show that the correlation between DTL-HS-Regnet predictions and the presence of cancer-associated fibroblasts is comparable to that of pathologists.Significance.Our proposed AI method offers a generalized approach for cancer diagnosis and prognosis prediction in pathology. The outstanding performance of the DTL-HS-Regnet model demonstrates its potential for improving current practices in image digital pathology, expanding the boundaries of cancer treatment in two critical areas.
更多查看译文
关键词
transfer learning,generalized ai method,prognosis prediction,pathology cancer diagnosis,hierarchical split
AI 理解论文
溯源树
样例
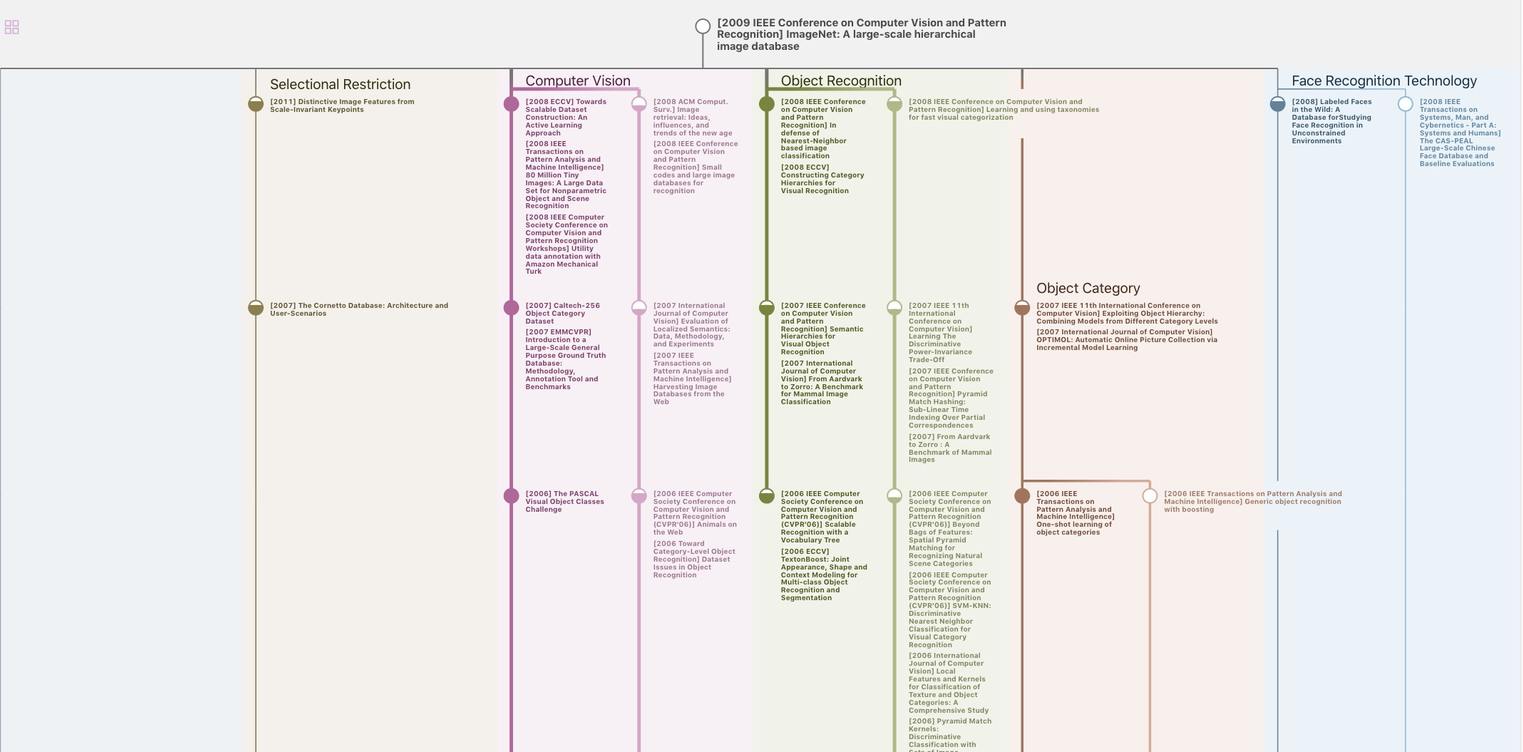
生成溯源树,研究论文发展脉络
Chat Paper
正在生成论文摘要