Camera Contrast Learning for Unsupervised Person Re-Identification
IEEE Transactions on Circuits and Systems for Video Technology(2023)
摘要
Unsupervised person re-identification (Re-ID) aims at finding the most informative features from unlabeled person datasets. Some recent approaches adopted camera-aware strategies for model training and have thereby achieved highly promising results. However, these methods simultaneously address intra-ID discrepancies of all cameras and require independent learning under each camera, which increases the complexity of algorithm. To resolve this issue, we present a camera contrast learning framework for unsupervised person Re-ID. Our method first proposes a time-based camera contrastive learning module to facilitate model learning. At each iteration, we follow the time contrast principle to select one camera centroid as proxy of each cluster. By enforcing the samples to converge to positive proxies, the correlation between features and cameras can gradually be reduced. Moreover, we design a 3-dimensional attention module to further reduce intra-ID discrepancies caused by background shifts. By re-weighting each feature map element in a spatial-channel order, our module can exactly find identity-invariant semantic cues from regions of interest in person images, no matter how the background change. Experimental results on several popular datasets prove that our work surpasses existing unsupervised person Re-ID approaches to a remarkable extent. The source codes can be found in
https://github.com/HongweiZhang97/CCL
.
更多查看译文
关键词
contrast,person,re-identification
AI 理解论文
溯源树
样例
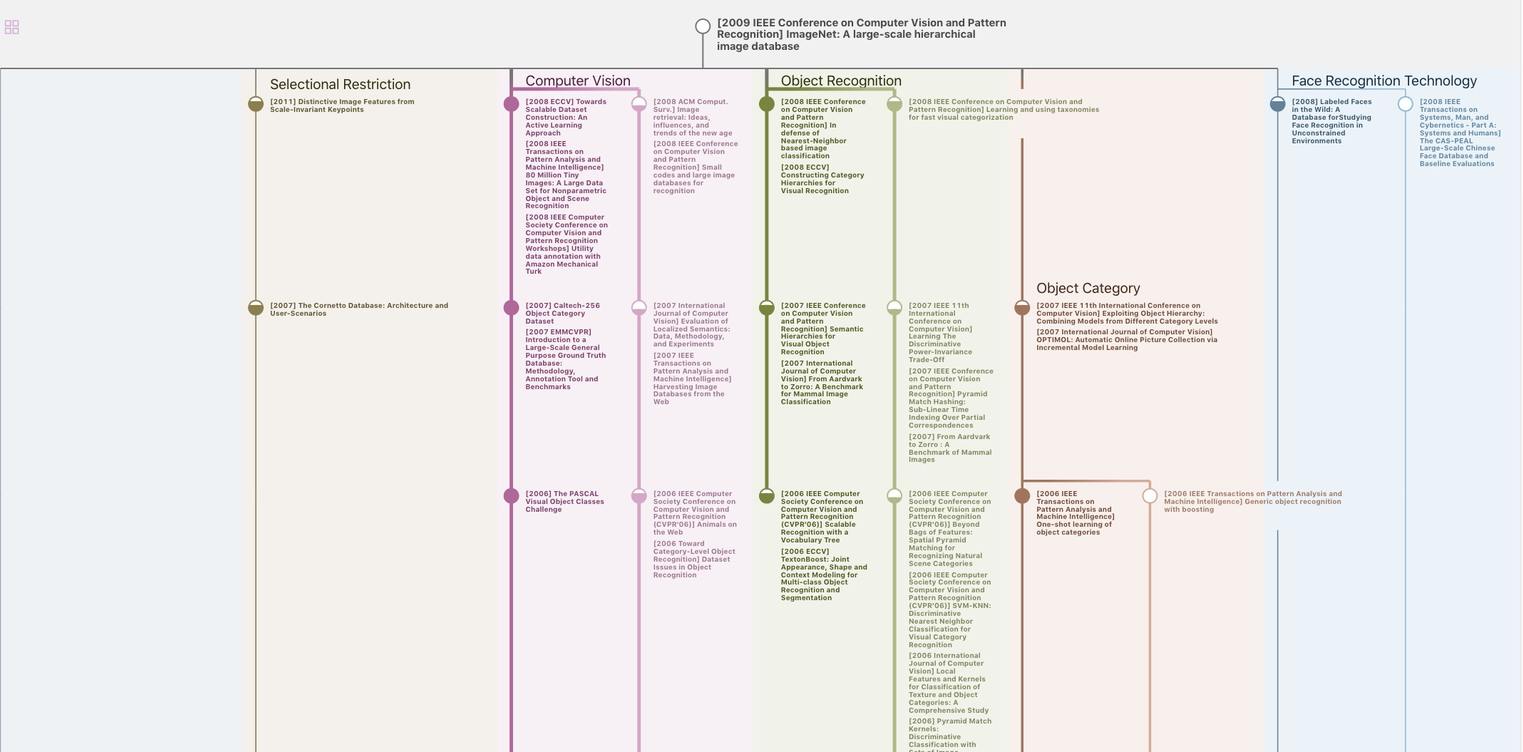
生成溯源树,研究论文发展脉络
Chat Paper
正在生成论文摘要