Graph-Based Scenario-Adaptive Lane-Changing Trajectory Planning for Autonomous Driving
ICRA 2024(2024)
Abstract
Trajectory planning is one of the key challenges to the rapid and large-scale deployment of autonomous driving. The lane-changing trajectory planning algorithm for autonomous driving is typically formulated as a optimization process of a cost function, which can be challenging to manually tune for different traffic scenarios. This paper presents a graph-based scenario-adaptive lane-changing trajectory planning approach that overcomes this challenge. Specifically, the cost function recovery method based on maximum entropy inverse reinforcement learning (IRL) is proposed to recover the cost functions of the all demonstrated lane-changing trajectories, and the cost function database is constructed. Then, the scenario matching model based on spatial-temporal graph convolutional network (ST-GCN) is proposed to match the recovered cost functions with the traffic scenarios, making the lane-changing trajectory planning method scenario-adaptive. Our proposed method is evaluated through simulations on the well-known NGSIM dataset and experiments on two typical lane-changing scenarios on the autonomous driving platform. The results show that our method is capable of learning the lane-changing cost function from demonstration and performing scenario-adaptive lane-changing trajectory planning.
MoreTranslated text
Key words
Autonomous Vehicle Navigation,Motion and Path Planning,Learning from Demonstration
AI Read Science
Must-Reading Tree
Example
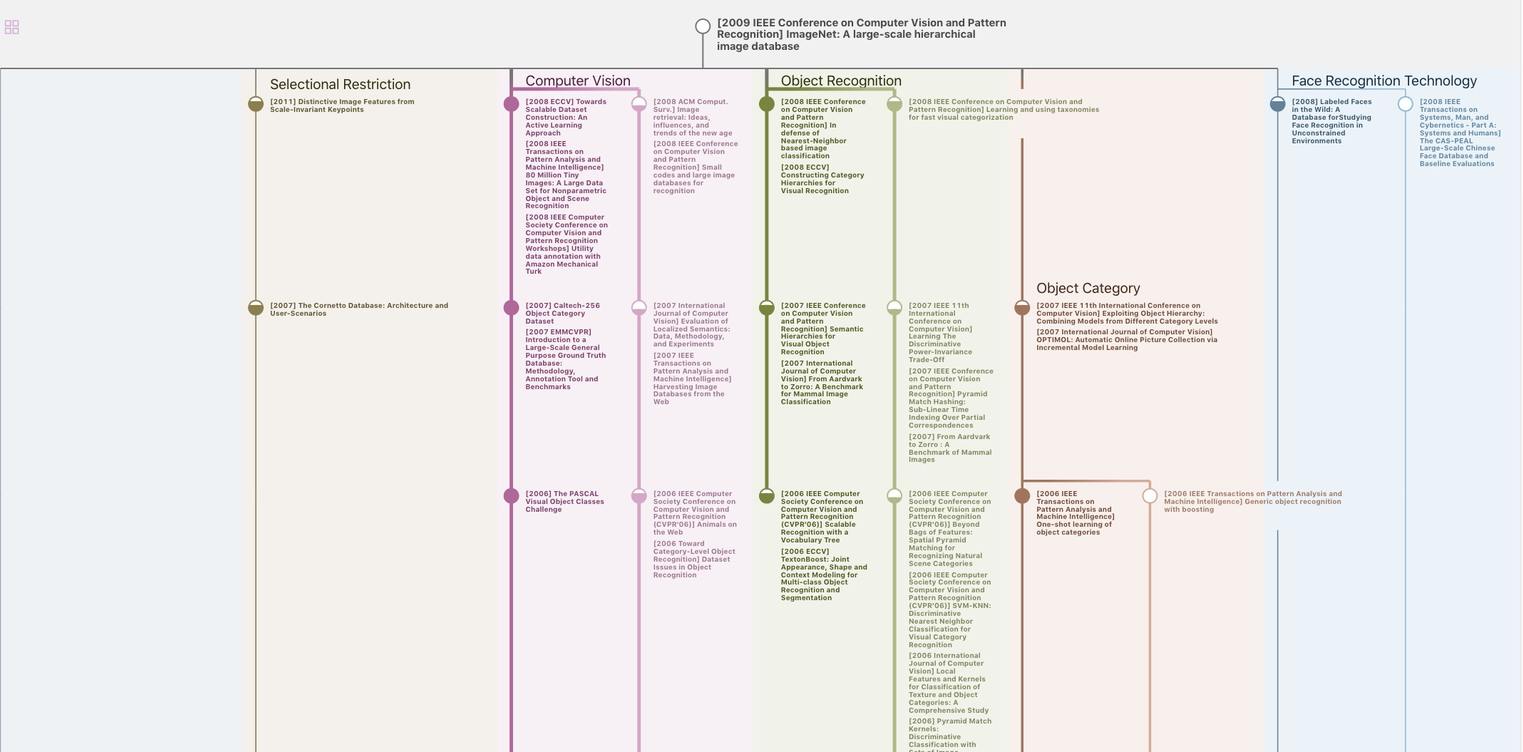
Generate MRT to find the research sequence of this paper
Chat Paper
Summary is being generated by the instructions you defined