PRM232 - LONGITUDINAL MATCHING: A METHOD FOR GENERATING COMPARABLE SAMPLES OF TREATED AND TREATMENT-NAÏVE PATIENTS WITH PROGRESSIVE CONDITIONS
VALUE IN HEALTH(2018)
Abstract
Evaluating the long-term effects of treatment for progressive conditions via RCTs may be difficult or even unethical, and careful analysis of real world data using techniques such as matching provides a useful alternative. This study proposes a method - Longitudinal Matching - that uses observational data to approximate an RCT by matching treated patients with treatment-naïve patients at a similar stage of disease progression. Longitudinal Matching can be applied to separate datasets of treated and untreated patients with different (or no) baseline dates, as the algorithm pairs an untreated patient, coupled with an index observation date, to each treated patient at the start of observation. Untreated patient histories are discretized to create “pseudo-patients” with distinct observation windows starting every month from the start of patient data until its end. Each treated patient is matched to the pseudo-patient that minimizes the Mahalanobis distance between the pair at the start of treatment/observation. A single untreated patient may contribute multiple pseudo-patients to the matching. This introduces a trade-off between match quality, on the one hand, and power. Allowing better suited untreated patients to contribute more pseudo-patients improves balance, while reducing power as standard errors in subsequent comparisons of treated and matched patients are clustered at the unique patient level. An application to lipodystrophy, a rare condition with limited patient data, estimates the treatment effect of leptin replacement therapy on mortality using data from a single-arm trial (n=112) and a natural history cohort (n=230) (HR=0.281, p=0.017). Longitudinal matching successfully balanced baseline characteristics between treated and untreated lipodystrophy patients, thereby reducing bias due to differences in observed covariates. This method has potential applications to studies of treatments for other progressive conditions.
MoreTranslated text
Key words
longitudinal matching,patients
AI Read Science
Must-Reading Tree
Example
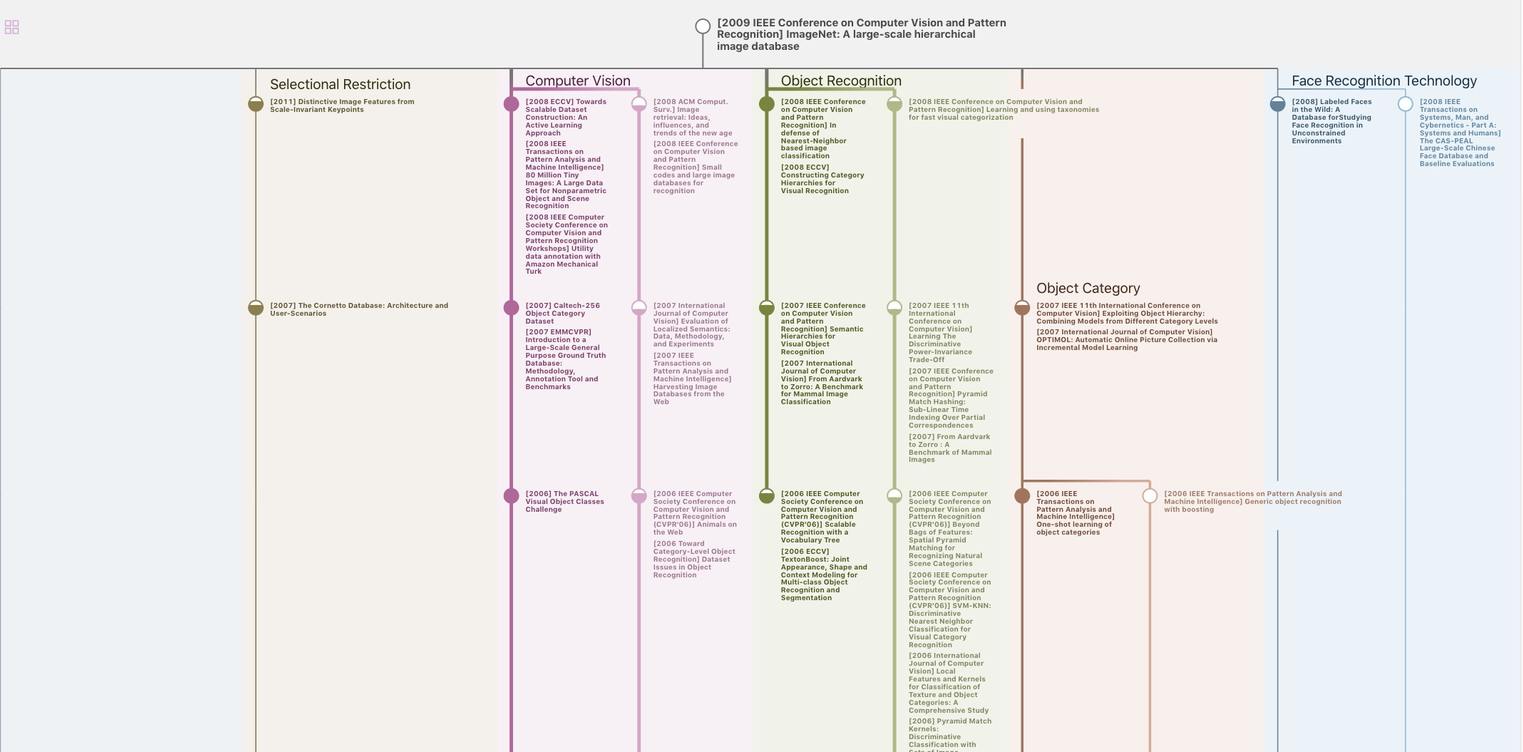
Generate MRT to find the research sequence of this paper
Chat Paper
Summary is being generated by the instructions you defined