Application of network embedding and transcriptome data in supervised drug repositioning
International Journal of Information Technology(2023)
Abstract
Computational drug repositioning gains more attention due to offering alternative treatments with lower development costs. This study proposes a machine learning-based drug repositioning approach by integrating various biological data. Transcriptome profiles of drug treatments and cancer patients are represented as gene expression profiles, which have been integrated into protein-protein interaction networks. The aim is to summarize molecular interaction data covered in biological networks, therefore individual networks were analyzed by attributed biased random walk (ABRW) to get low-dimensional representations of disease and drug networks. One of novel contributions this study is usage of ABRW as an embedding method in biological domain. It provides vector embeddings for drug and disease networks that have been later combined to build cancer specific drug repositioning models. We employed these supervised models to predict whether a given drug might be suggested as an alternative treatment for lung cancer or breast cancer. The experiments on new drug samples revealed more than 20 drugs as potential treatment candidates for two cancers. Comparison with another drug repositioning approach showed common predictions which have been used in some clinical trials. The application of an attributed network embedding algorithm for biological networks and its usage in the feature extraction step of a drug repositioning approach are two novel contributions of this study.
MoreTranslated text
Key words
Network embedding, Drug repositioning, Supervised learning, Breast cancer, Lung cancer
AI Read Science
Must-Reading Tree
Example
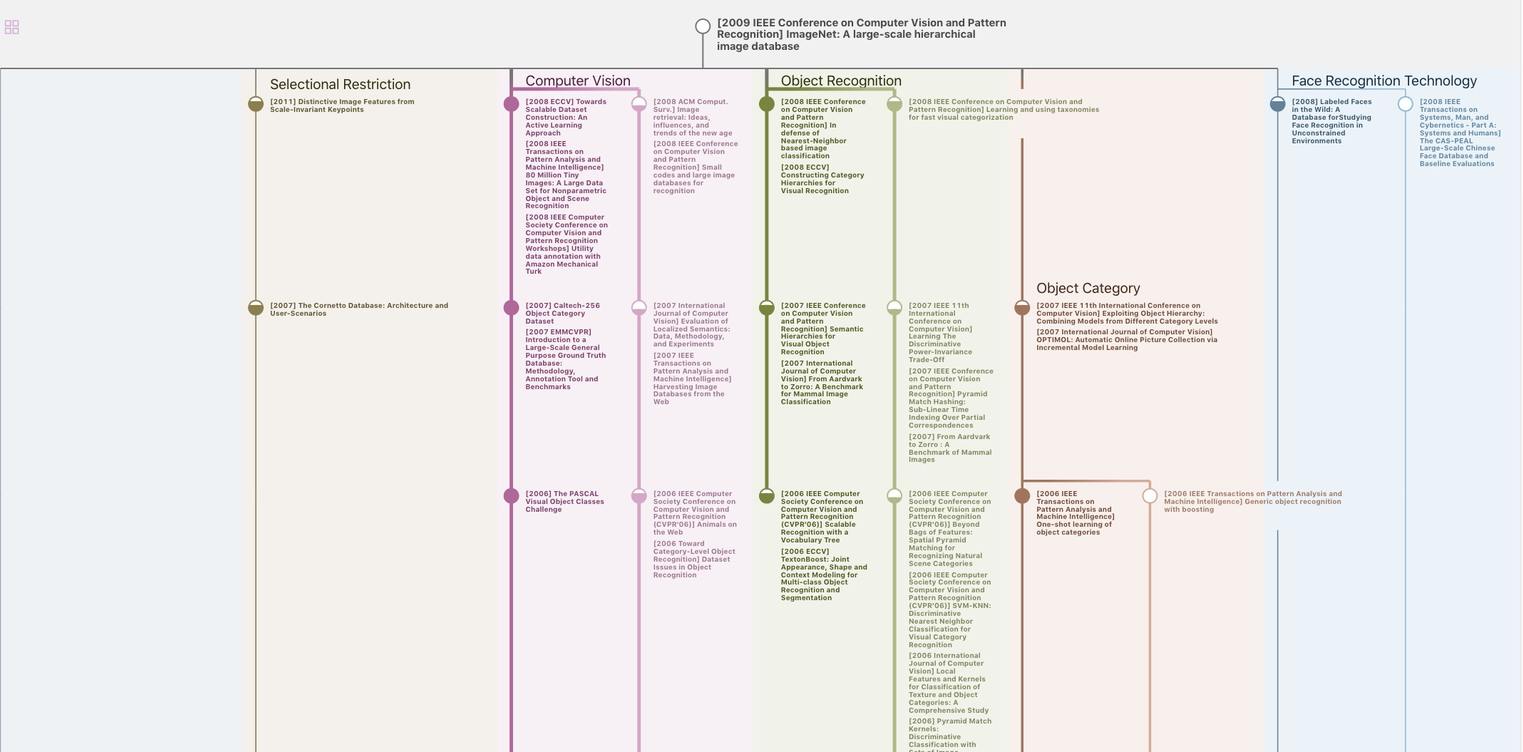
Generate MRT to find the research sequence of this paper
Chat Paper
Summary is being generated by the instructions you defined