Gaussian Process for Nonlinear Regression via Memristive Crossbars.
ISCAS(2023)
摘要
Over the last decade, Gaussian processes (GPs) have become popular in the area of machine learning and data analysis for their flexibility and robustness. Despite their attractive formulation, practical use in large-scale problems remains out of reach due to computational complexity. Existing direct computational methods for manipulations involving large-scale nxn covariance matrices require O(n3) calculations. In this work, we present the design and evaluation of a simulated computing platform for exact GP inference, that achieves true model parallelism using memristive crossbars. To achieve a one-shot solution, a linear equation solver and a vector-matrix multiplication solver crossbar configurations are used together, reducing the number of operations from O(n3) to O(n). The transistor level op-amps, ADC models for quantization, circuit and interconnect parasitics, together with the finite memristor precision are incorporated into the system simulation. The analog system resulted in %1.51 mean error and %2.93 average variance error in solving a nonlinear regression problem. The proposed method achieved 9x to 144x better energy efficiency compared to TPU and 7x compared to a custom analog linear regression solver.
更多查看译文
关键词
Analog Computation, Bayesian Optimization, Crossbars, Gaussian Process, Memristor, Nonlinear Regression
AI 理解论文
溯源树
样例
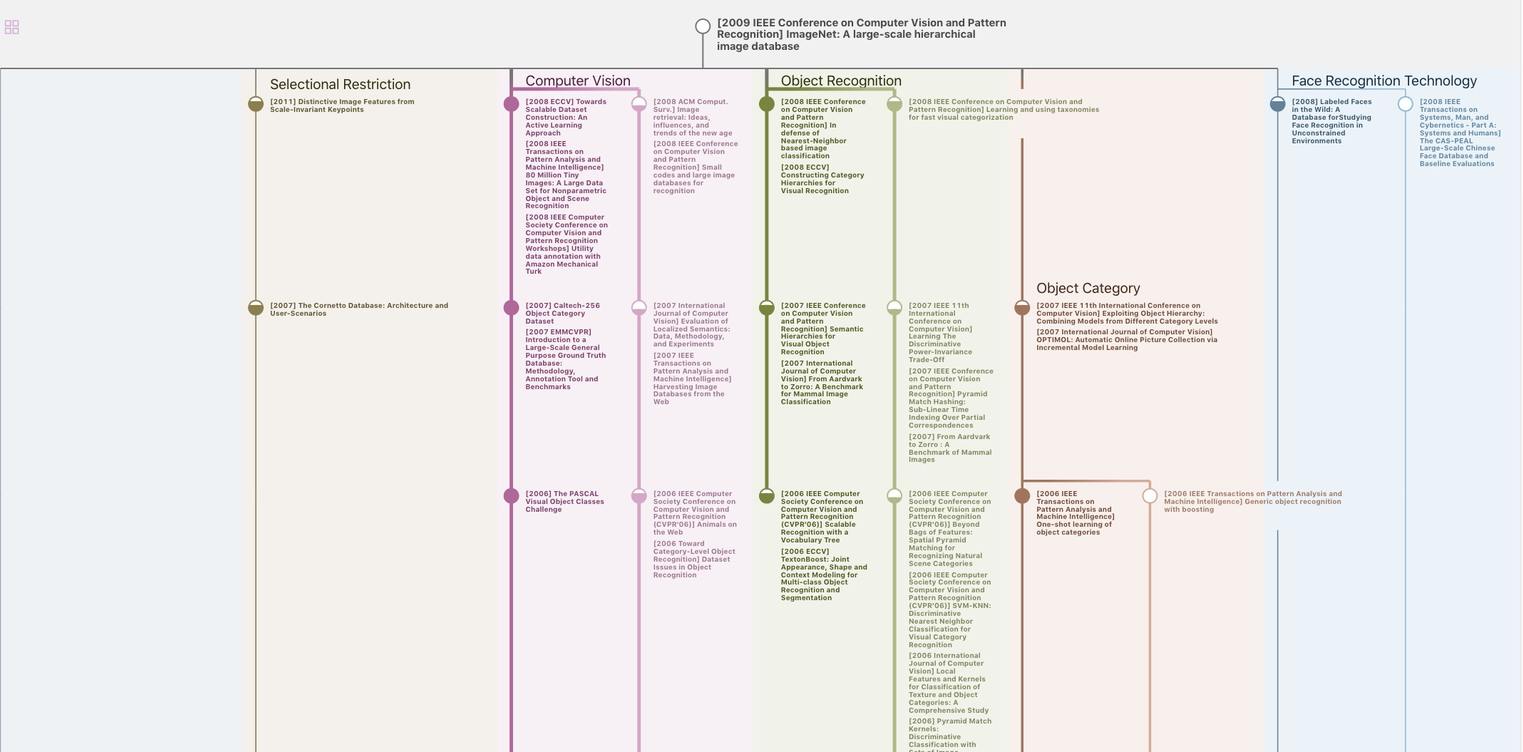
生成溯源树,研究论文发展脉络
Chat Paper
正在生成论文摘要