Feature-Embedding Triplet Networks with a Separately Constrained Loss Function.
ISCAS(2023)
摘要
Feature-embedding triplet networks (TNs) with three symmetric subchannels are very promising for similaritymeasuring applications. This paper proposes a novel separately constrained triple loss (SCTL) function that applies to TNs for classification. Through minimizing the intra-class distance and maximizing the inter-class distance, SCTL eliminates possible false solutions and provides insight into the dependency of training based on these two terms. Based on this dependency, the strategy of selecting hyperparameters in SCTL is also analyzed to further improve performance. The effectiveness of the proposed SCTL is evaluated based on TNs with multi-layer perceptrons; the results show that compared to all existing loss functions, the use of SCTL offers the best classification accuracy for the TNs, while incurring in negligible hardware overhead (e.g., only a 0.0002% area overhead of the subnetworks).
更多查看译文
关键词
triplet networks,classification,loss function,multi-layer perceptron,machine learning
AI 理解论文
溯源树
样例
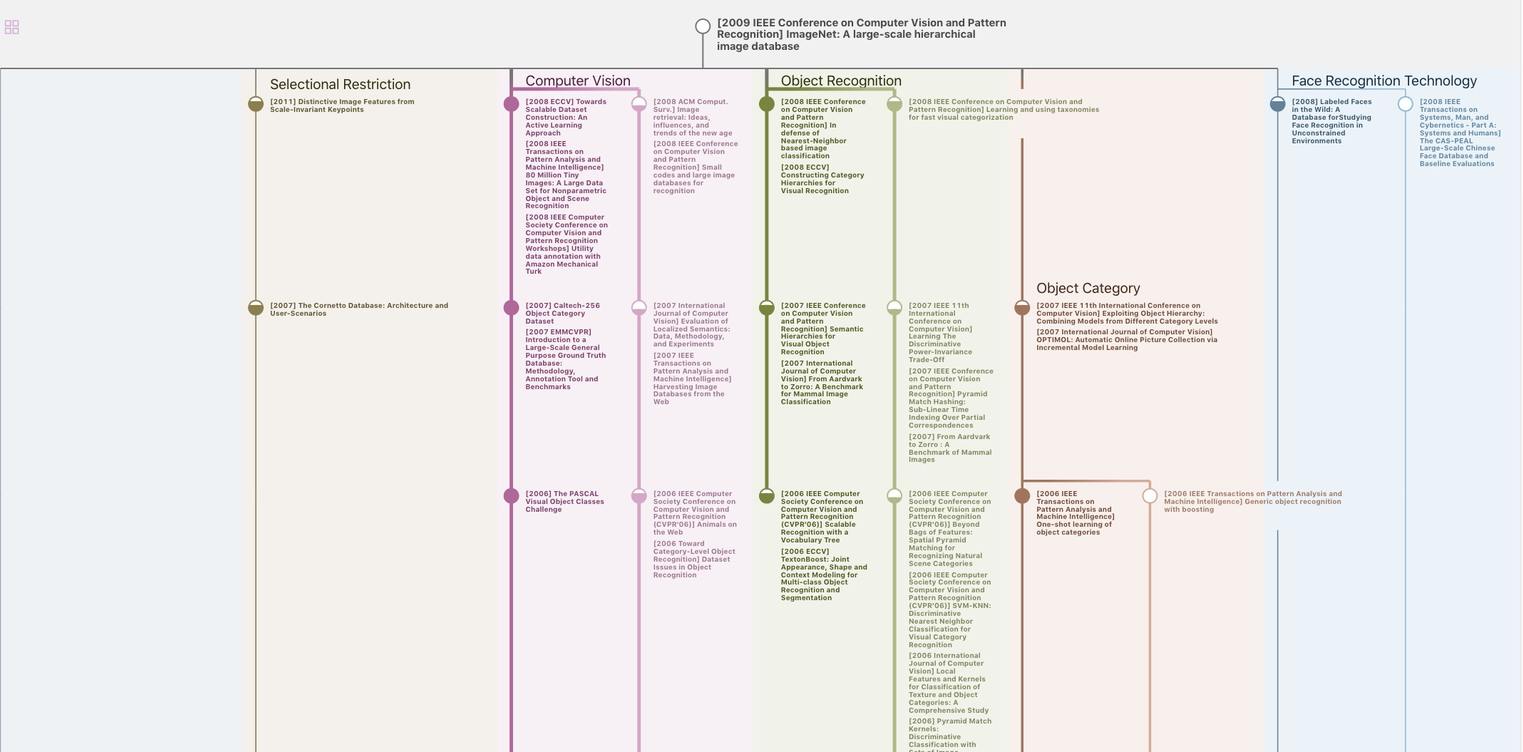
生成溯源树,研究论文发展脉络
Chat Paper
正在生成论文摘要