Enabling Long-Term Robustness in RRAM-based Compute-In-Memory Edge Devices
ISCAS(2023)
Abstract
The states of resistive random-access memory (RRAM) have been shown to drift from read voltage stress. When using RRAM as weight elements in deep neural networks (DNNs), this drift degrades inference accuracy over time. To maintain accuracy, RRAM cells must be frequently reprogrammed back to their initial state. We propose a method for reprogramming RRAM memory arrays without accessing the nominal DNN parameters stored off-chip (e.g., in cloud). The method utilizes techniques in linear algebra to calculate the correct state of the RRAM as needed. Resistance drift of RRAM cells were measured from a 40 nm RRAM compute-in-memory (CIM) macro test chip, and the impact on inference accuracy was simulated. Then, the accuracy of the method was evaluated and the recovery in inference accuracy was recorded. Results suggest the method is capable of correctly calculating RRAM resistance states in the presence of resistance drift and other realistic chip noises. The method was also evaluated at reduced precision analog-to-digital conversion to examine its effectiveness in a practical CIM design.
MoreTranslated text
Key words
analog-to-digital conversion,deep neural networks,nominal DNN parameters,random-access memory,read voltage stress,resistance drift,RRAM cells,RRAM compute-in-memory macro test chip,RRAM memory arrays,RRAM resistance states,RRAM-based compute-in-memory edge devices,size 40.0 nm
AI Read Science
Must-Reading Tree
Example
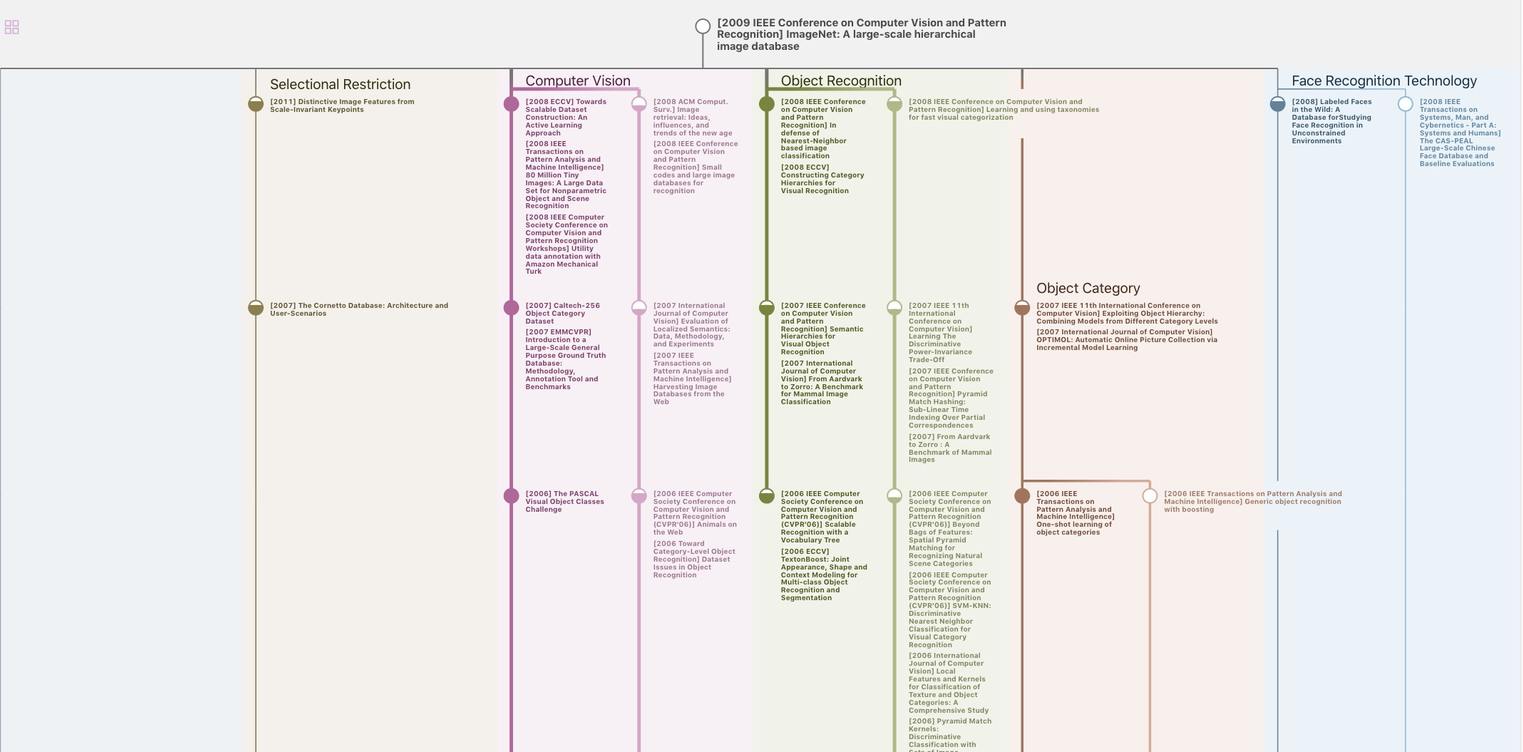
Generate MRT to find the research sequence of this paper
Chat Paper
Summary is being generated by the instructions you defined