Learning Electrical Behavior of Core Interconnects for System-Level Crosstalk Prediction.
ETS(2023)
摘要
Efficient distribution of tasks in an SOC between various components of an embedded system affect rate of data exchange between cores and obviously the number and fanout of interconnecting cores. Data rate and interconnect fanouts depend on post-layout wire characteristics that, in the worst-case situation, must be evaluated for abovementioned system level decisions. In this work we are making provisions for avoiding this large gap between high-level decision making and low-level physical properties. IP-core interconnects can be fully characterized by post layout information of the IP-core, load properties, and the number of destination cores they are driving. This information can be back-annotated into abstract system-level interconnect models to be used by core integrators for design space exploration (DSE). Fanout and/or frequency of operation of an IP-core can be decided by this DSE environment. In this work, we propose a machine-learning based methodology that uses signoff parasitic information and the actual wire data to generate the dataset and train a model. The model was evaluated in fast high-level SystemC environment for two RISC-V based processors in two SoCs. The models were 26 times faster than the low-level simulations with a crosstalk fault coverage of 1.5% error.
更多查看译文
关键词
Crosstalk, interconnect, SoC, machine learning, RISC-V, SystemC
AI 理解论文
溯源树
样例
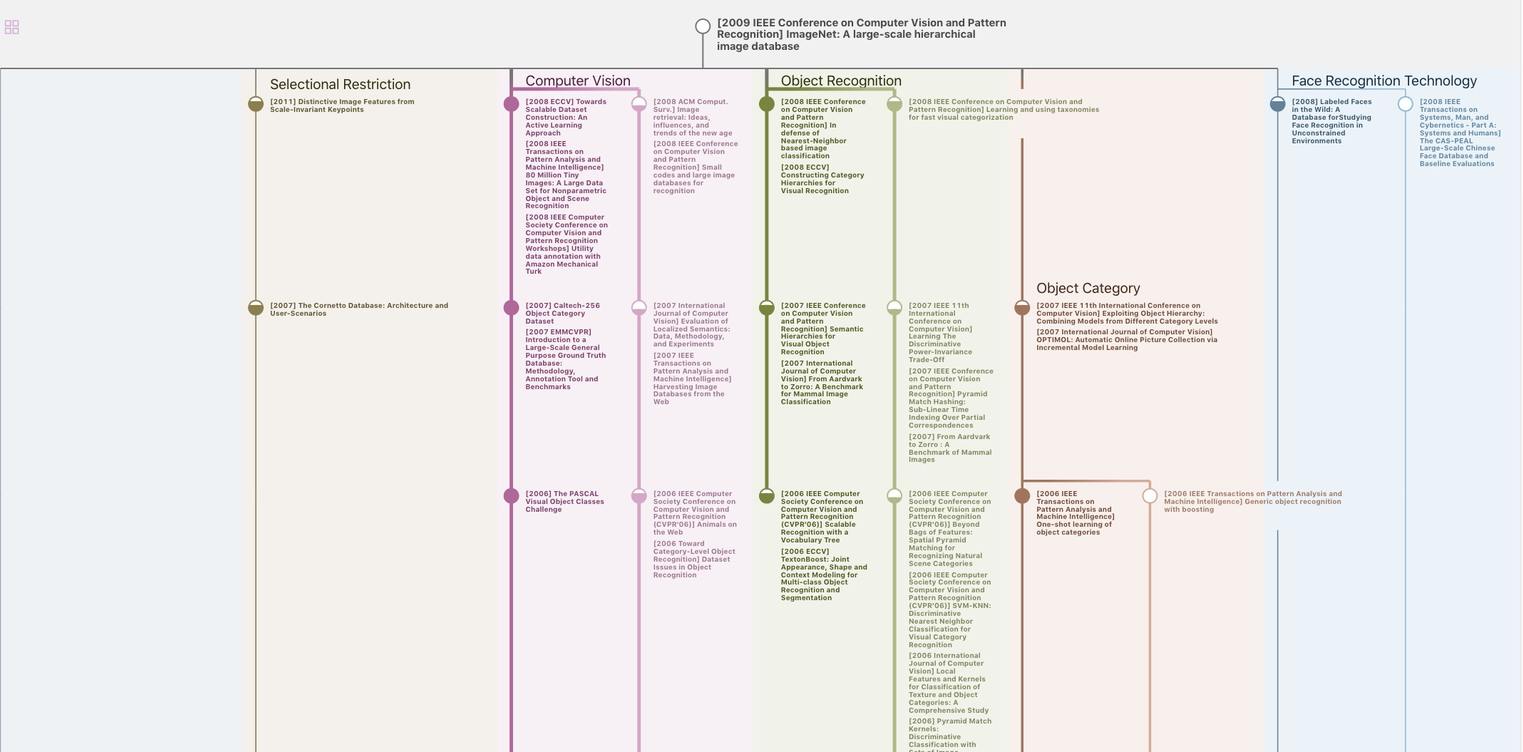
生成溯源树,研究论文发展脉络
Chat Paper
正在生成论文摘要