A Dataset for Learning University STEM Courses at Scale and Generating Questions at a Human Level.
AAAI(2023)
Abstract
We present a new dataset for learning to solve, explain, and generate university-level STEM questions from 27 courses across a dozen departments in seven universities. We scale up previous approaches to questions from courses in the departments of Mechanical Engineering, Materials Science and Engineering, Chemistry, Electrical Engineering, Computer Science, Physics, Earth Atmospheric and Planetary Sciences, Economics, Mathematics, Biological Engineering, Data Systems, and Society, and Statistics. We visualize similarities and differences between questions across courses. We demonstrate that a large foundation model is able to generate questions that are as appropriate and at the same difficulty level as human-written questions.
MoreTranslated text
AI Read Science
Must-Reading Tree
Example
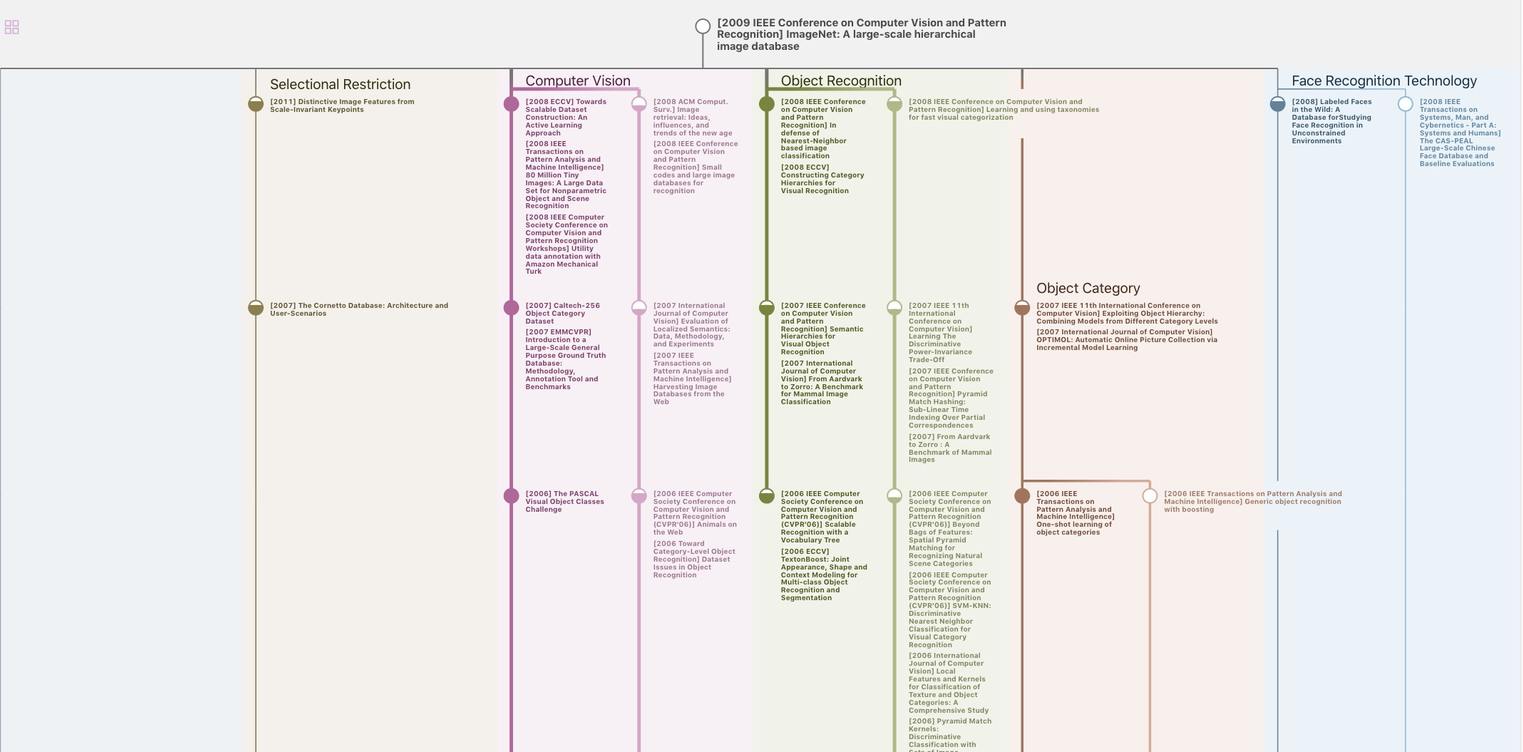
Generate MRT to find the research sequence of this paper
Chat Paper
Summary is being generated by the instructions you defined