Compressed Decentralized Learning of Conditional Mean Embedding Operators in Reproducing Kernel Hilbert Spaces
AAAI 2023(2023)
摘要
Conditional mean embedding (CME) operators encode conditional probability densities within Reproducing Kernel Hilbert Space (RKHS). In this paper, we present a decentralized algorithm for a collection of agents to cooperatively approximate CME over a network. Communication constraints limit the agents from sending all data to their neighbors; we only allow sparse representations of covariance operators to be exchanged among agents, compositions of which defines CME. Using a coherence-based compression scheme, we present a consensus-type algorithm that preserves the average of the approximations of the covariance operators across the network. We theoretically prove that the iterative dynamics in RKHS is stable. We then empirically study our algorithm to estimate CMEs to learn spectra of Koopman operators for Markovian dynamical systems and to execute approximate value iteration for Markov decision processes (MDPs).
更多查看译文
关键词
ML: Kernel Methods,MAS: Multiagent Learning
AI 理解论文
溯源树
样例
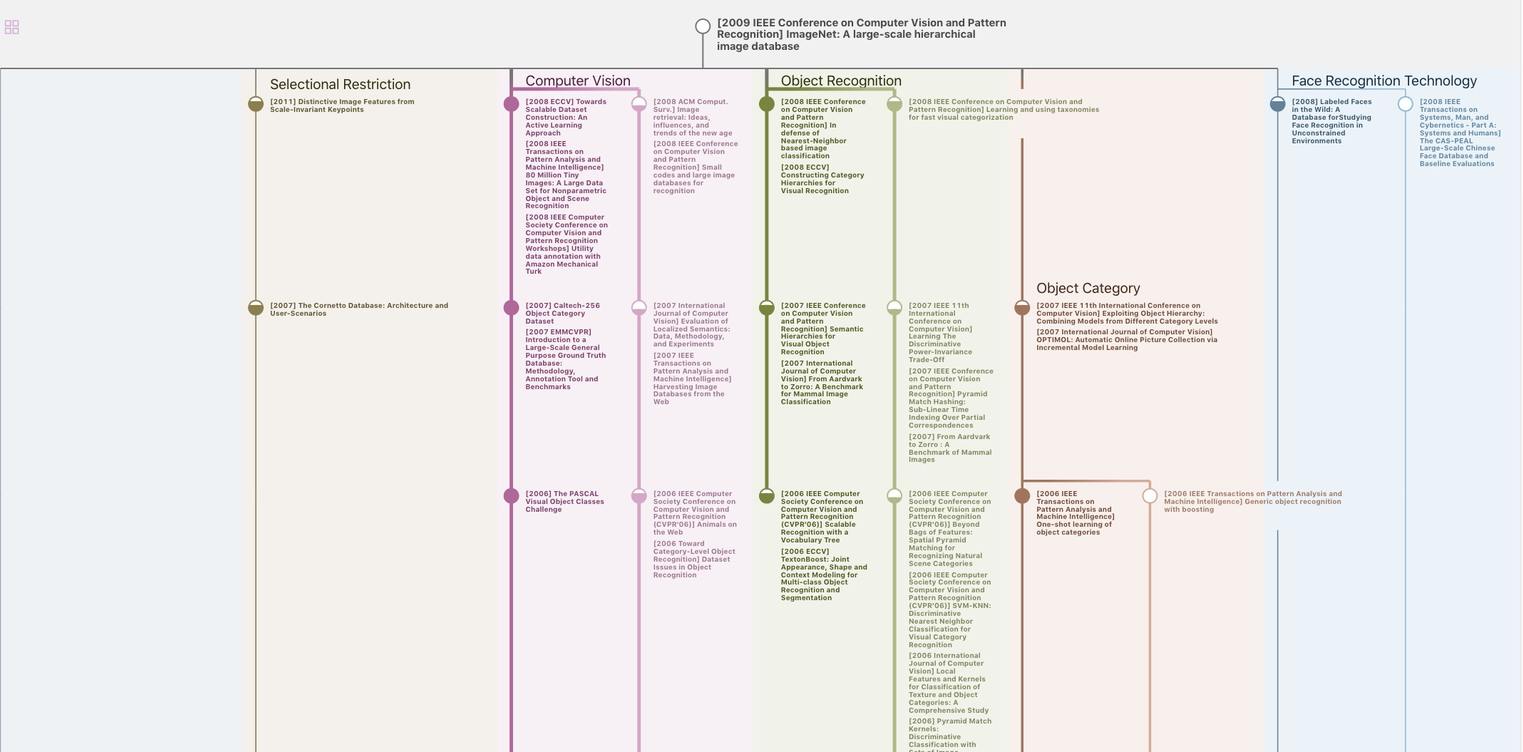
生成溯源树,研究论文发展脉络
Chat Paper
正在生成论文摘要