CLGT: A Graph Transformer for Student Performance Prediction in Collaborative Learning.
AAAI(2023)
摘要
Modeling and predicting the performance of students in collaborative learning paradigms is an important task. Most of the research presented in literature regarding collaborative learning focuses on the discussion forums and social learning networks. There are only a few works that investigate how students interact with each other in team projects and how such interactions affect their academic performance. In order to bridge this gap, we choose a software engineering course as the study subject. The students who participate in a software engineering course are required to team up and complete a software project together. In this work, we construct an interaction graph based on the activities of students grouped in various teams. Based on this student interaction graph, we present an extended graph transformer framework for collaborative learning (CLGT) for evaluating and predicting the performance of students. Moreover, the proposed CLGT contains an interpretation module that explains the prediction results and visualizes the student interaction patterns. The experimental results confirm that the proposed CLGT outperforms the baseline models in terms of performing predictions based on the real-world datasets. Moreover, the proposed CLGT differentiates the students with poor performance in the collaborative learning paradigm and gives teachers early warnings, so that appropriate assistance can be provided.
更多查看译文
关键词
student performance prediction,graph transformer,learning
AI 理解论文
溯源树
样例
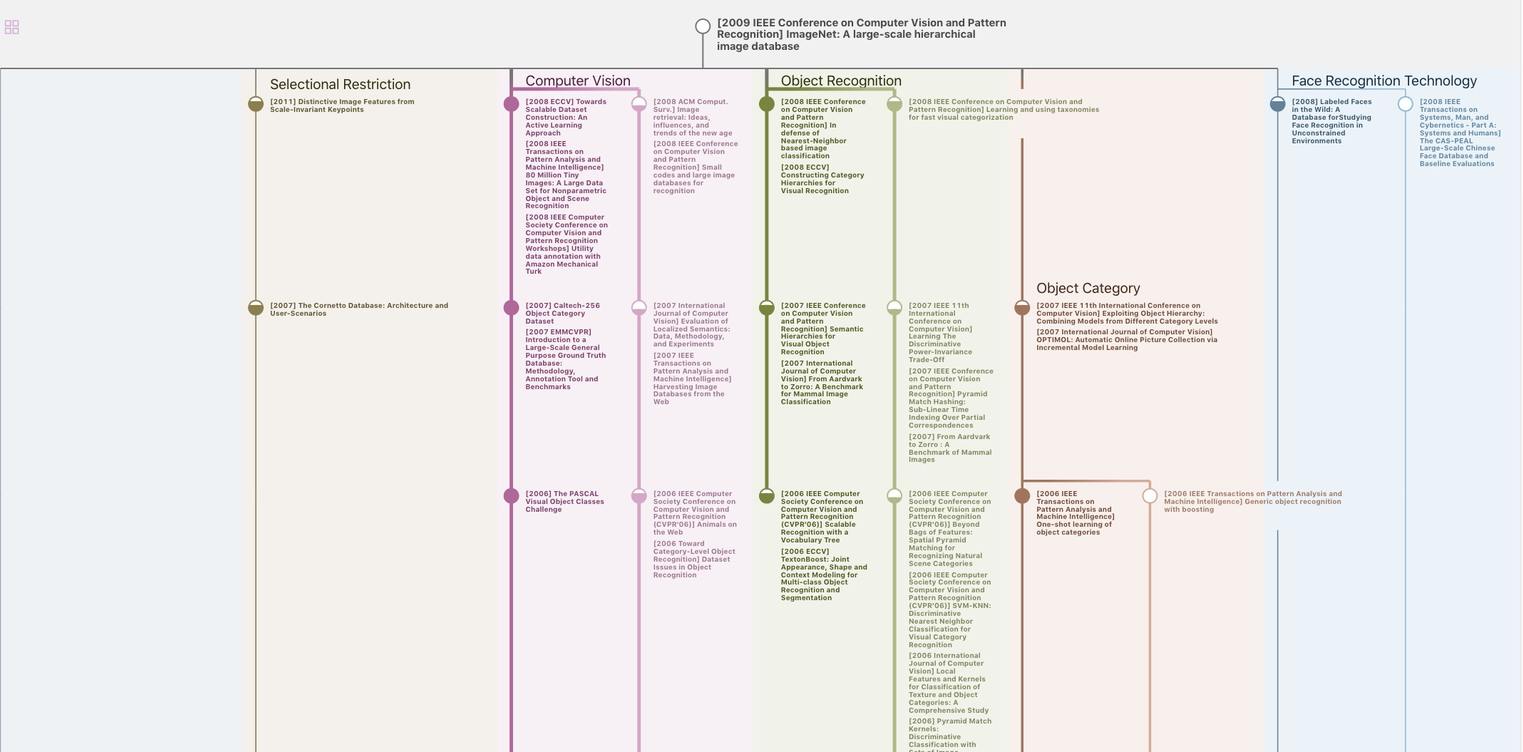
生成溯源树,研究论文发展脉络
Chat Paper
正在生成论文摘要