Solving Large-Scale Pursuit-Evasion Games Using Pre-trained Strategies
AAAI 2023(2023)
Abstract
Pursuit-evasion games on graphs model the coordination of police forces chasing a fleeing felon in real-world urban settings, using the standard framework of imperfect-information extensive-form games (EFGs). In recent years, solving EFGs has been largely dominated by the Policy-Space Response Oracle (PSRO) methods due to their modularity, scalability, and favorable convergence properties. However, even these methods quickly reach their limits when facing large combinatorial strategy spaces of the pursuit-evasion games. To improve their efficiency, we integrate the pre-training and fine-tuning paradigm into the core module of PSRO -- the repeated computation of the best response. First, we pre-train the pursuer's policy base model against many different strategies of the evader. Then we proceed with the PSRO loop and fine-tune the pre-trained policy to attain the pursuer's best responses. The empirical evaluation shows that our approach significantly outperforms the baselines in terms of speed and scalability, and can solve even games on street maps of megalopolises with tens of thousands of crossroads -- a scale beyond the effective reach of previous methods.
MoreTranslated text
Key words
MAS: Multiagent Learning,APP: Energy, Environment & Sustainability,APP: Security,ML: Representation Learning
AI Read Science
Must-Reading Tree
Example
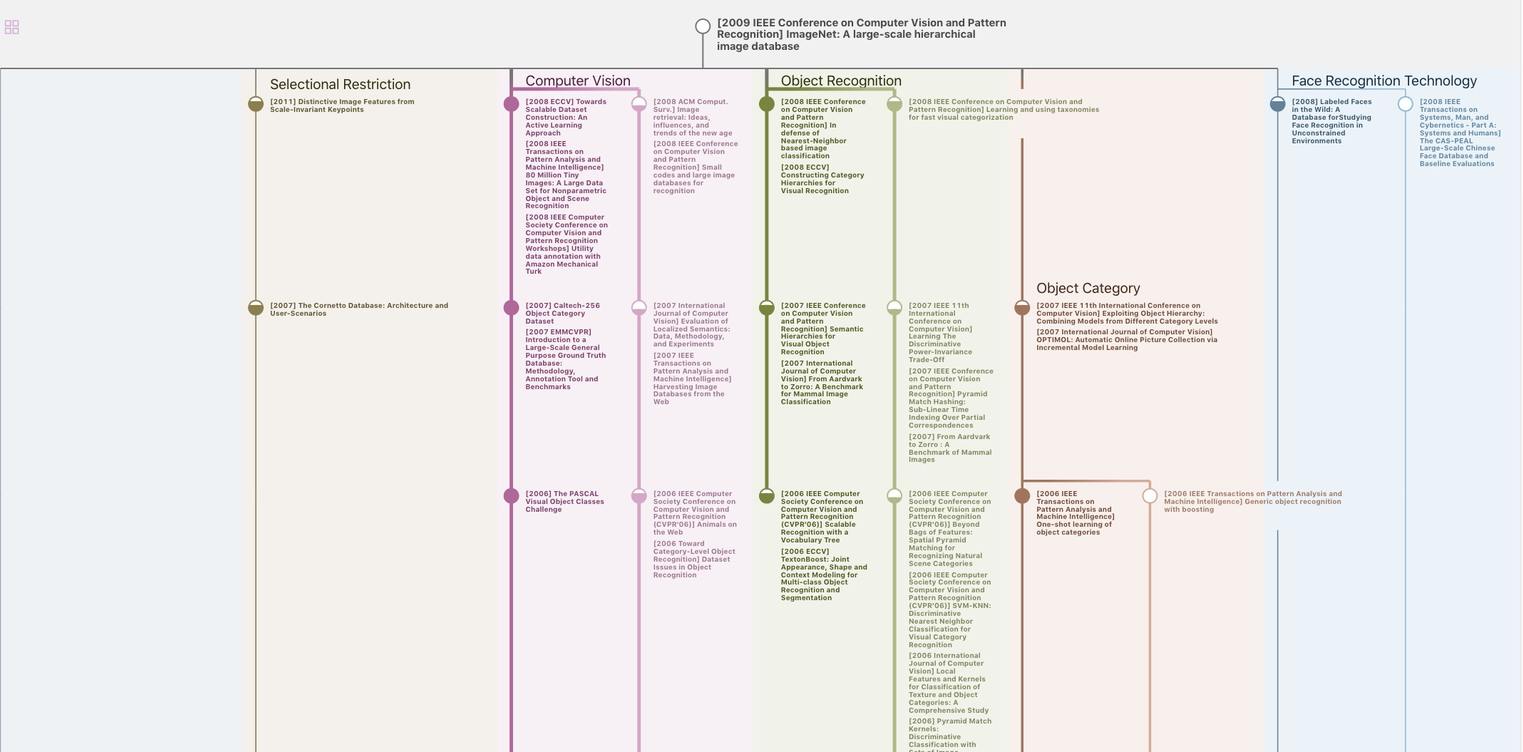
Generate MRT to find the research sequence of this paper
Chat Paper
Summary is being generated by the instructions you defined