Preference-Controlled Multi-Objective Reinforcement Learning for Conditional Text Generation.
AAAI(2023)
摘要
Conditional text generation is to generate text sequences conditioning on linguistic or non-linguistic data. The main line of existing work proposed deterministic models to improve the fidelity of the generated text but often ignored the diversity. Another line relied on conditional variational autoencoders (CVAEs), which increased the diversity over their deterministic backbones. However, CVAEs regard diversity as an implicit objective and may not be optimal. In this paper, we raise two questions: i) Can diversity be further improved with an explicit objective? ii) Since fidelity and diversity are two conflicting objectives, how can we obtain different multi-objective optimal solutions according to user preferences? To answer question i), we propose a multi-objective reinforcement learning (MORL) method which explicitly takes CIDEr and Self-CIDEr scores as the fidelity-oriented and diversity-oriented rewards respectively. To answer question ii), we propose a preference-controlled MORL method, which can obtain infinite multi-objective optimal solutions by tuning the preference variable. We conduct extensive experiments on paraphrasing and image captioning tasks, which show that in the fidelity-diversity trade-off space, our model outperforms both deterministic and CVAE-based baselines.
更多查看译文
关键词
text,generation,reinforcement learning,preference-controlled,multi-objective
AI 理解论文
溯源树
样例
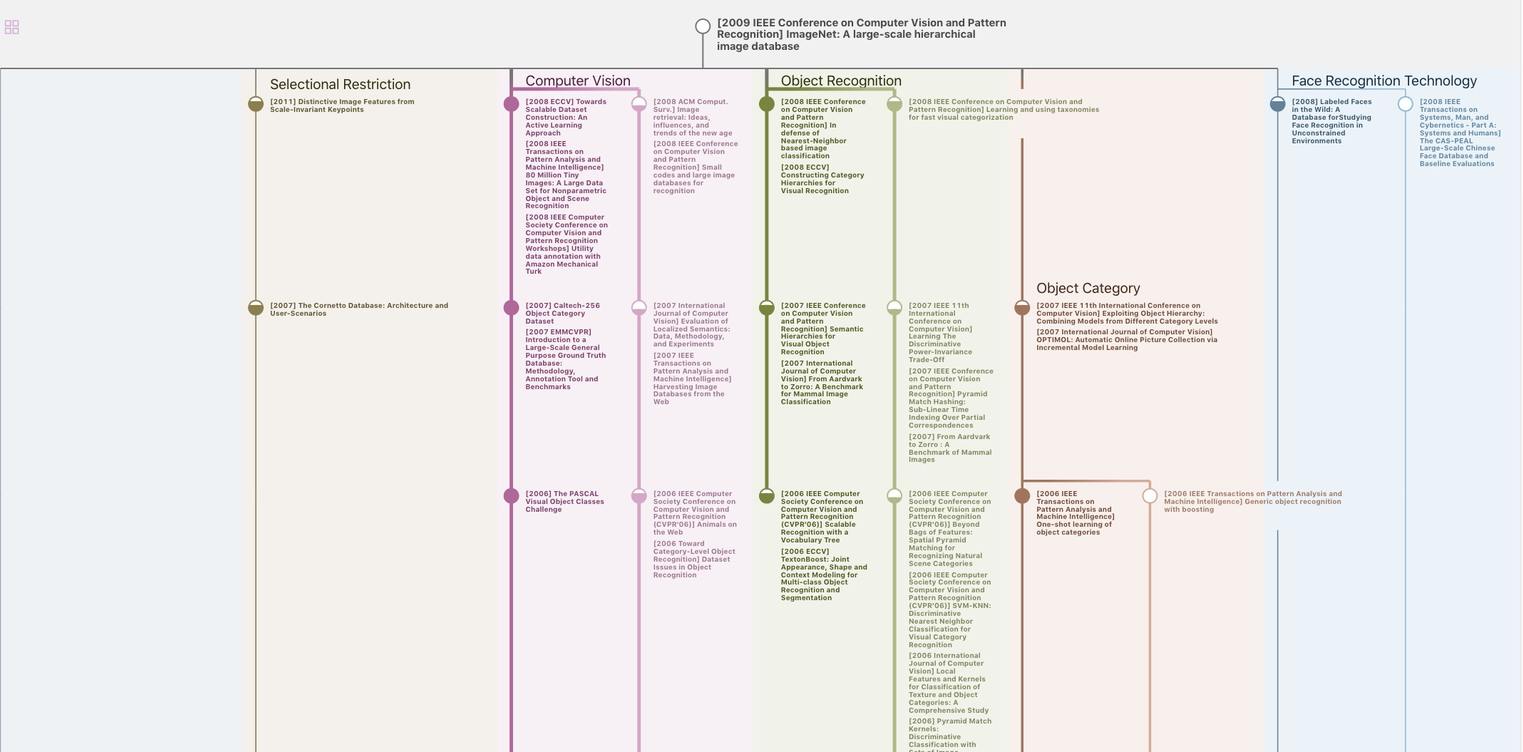
生成溯源树,研究论文发展脉络
Chat Paper
正在生成论文摘要