FedMDFG: Federated Learning with Multi-Gradient Descent and Fair Guidance.
AAAI(2023)
摘要
Fairness has been considered as a critical problem in federated learning (FL). In this work, we analyze two direct causes of unfairness in FL - an unfair direction and an improper step size when updating the model. To solve these issues, we introduce an effective way to measure fairness of the model through the cosine similarity, and then propose a federated multiple gradient descent algorithm with fair guidance (FedMDFG) to drive the model fairer. We first convert FL into a multi-objective optimization problem (MOP) and design an advanced multiple gradient descent algorithm to calculate a fair descent direction by adding a fair-driven objective to MOP. A low-communication-cost line search strategy is then designed to find a better step size for the model update. We further show the theoretical analysis on how it can enhance fairness and guarantee the convergence. Finally, extensive experiments in several FL scenarios verify that Fed-MDFG is robust and outperforms the SOTA FL algorithms in convergence and fairness.
更多查看译文
关键词
federated learning,fedmdfg,fair guidance,multi-gradient
AI 理解论文
溯源树
样例
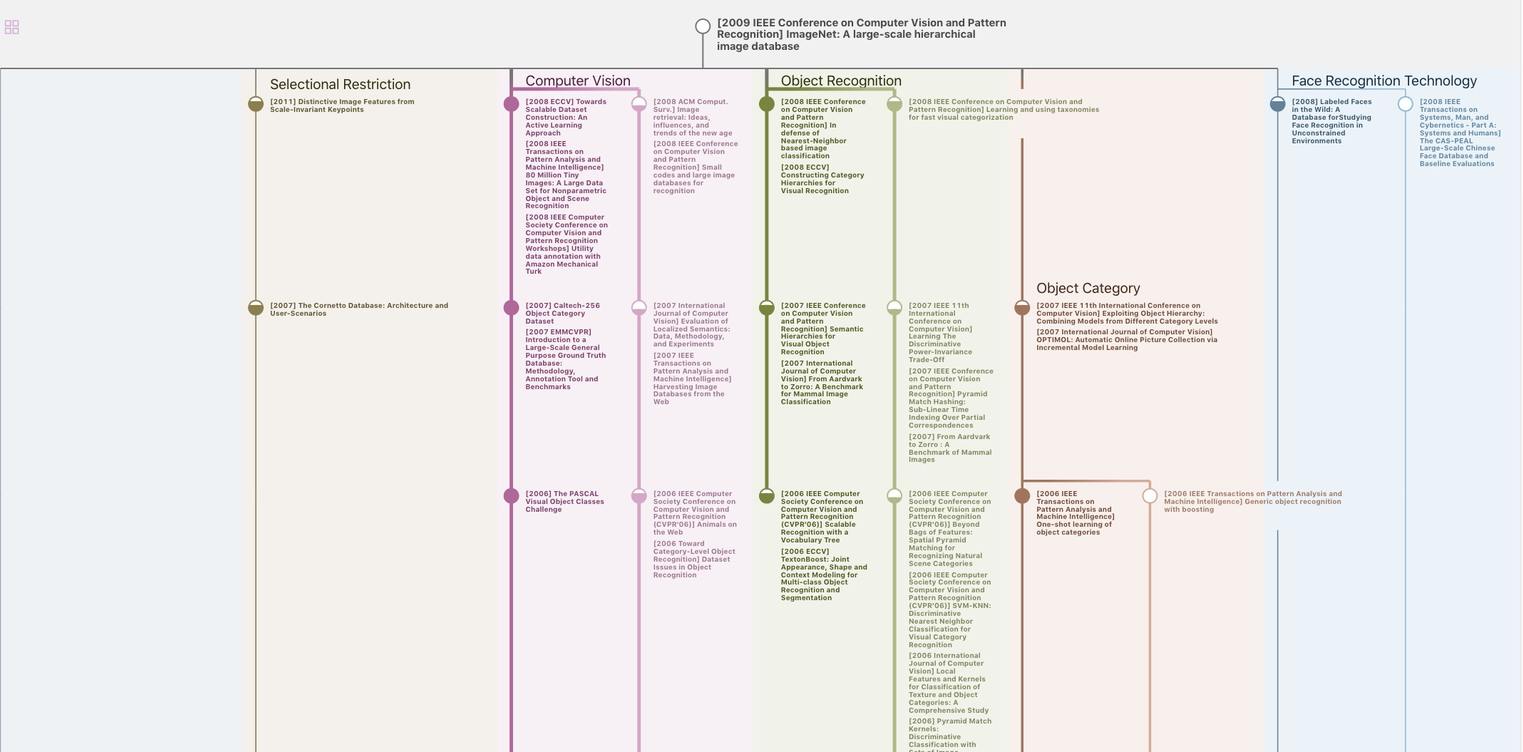
生成溯源树,研究论文发展脉络
Chat Paper
正在生成论文摘要