Rethinking Label Refurbishment: Model Robustness under Label Noise.
AAAI(2023)
摘要
A family of methods that generate soft labels by mixing the hard labels with a certain distribution, namely label refurbishment , are widely used to train deep neural networks. However, some of these methods are still poorly understood in the presence of label noise. In this paper, we revisit four label refurbishment methods and reveal the strong connection between them. We find that they affect the neural network models in different manners. Two of them smooth the estimated posterior for regularization effects, and the other two force the model to produce high-confidence predictions. We conduct extensive experiments to evaluate related methods and observe that both effects improve the model generalization under label noise. Furthermore, we theoretically show that both effects lead to generalization guarantees on the clean distribution despite being trained with noisy labels.
更多查看译文
关键词
label,model robustness,noise
AI 理解论文
溯源树
样例
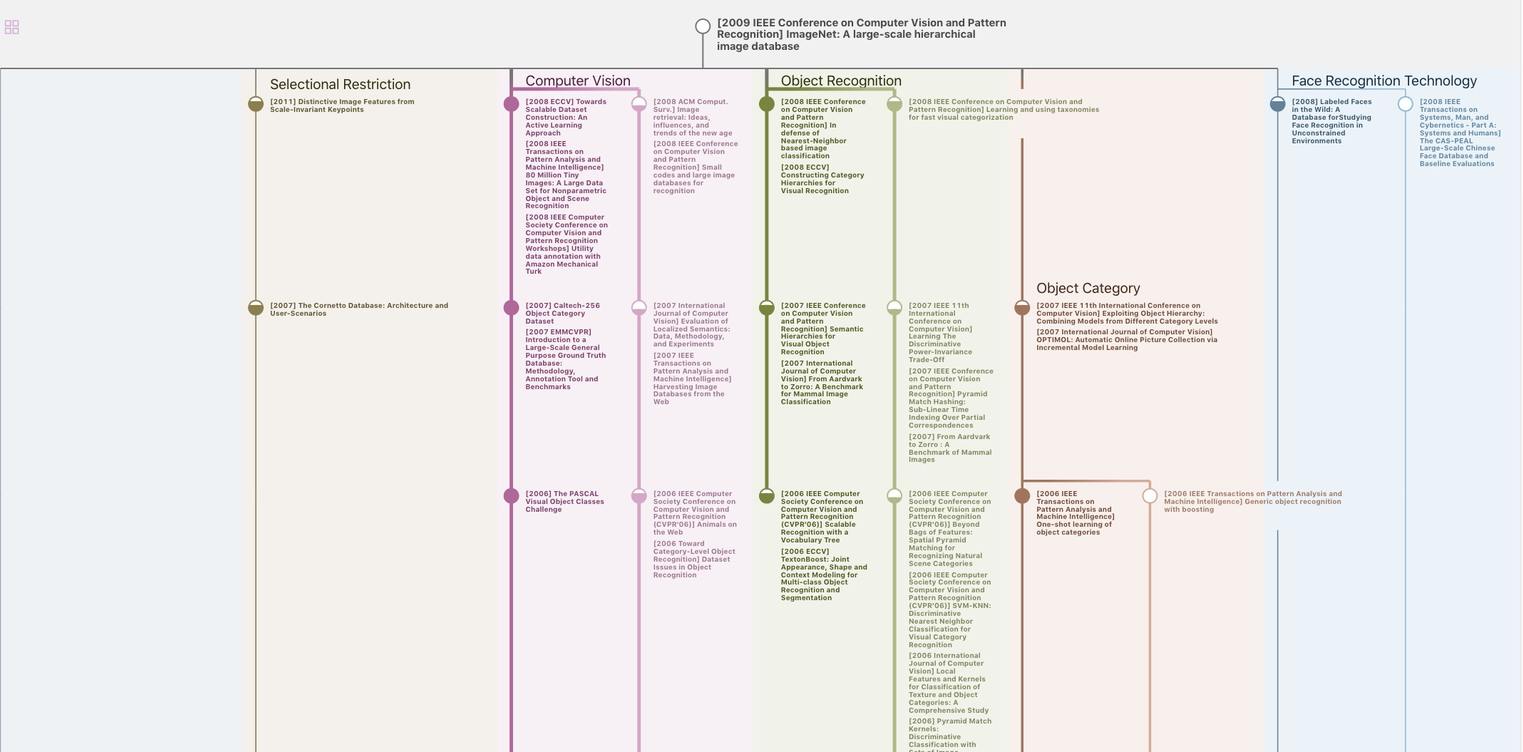
生成溯源树,研究论文发展脉络
Chat Paper
正在生成论文摘要