Latent Constraints on Unsupervised Text-Graph Alignment with Information Asymmetry.
AAAI(2023)
摘要
Unsupervised text-graph alignment (UTGA) is a fundamental task that bidirectionally generates texts and graphs without parallel data. Most available models of UTGA suffer from information asymmetry, a common phenomenon that texts and graphs include additional information invisible to each other. On the one hand, these models fail to supplement asymmetric information effectively due to the lack of ground truths. On the other hand, it is challenging to indicate asymmetric information with explicit indicators because it cannot be decoupled from the data directly. To address the challenge posed by information asymmetry, we propose the assumption that asymmetric information is encoded in unobservable latent variables and only affects the one-way generation processes. These latent variables corresponding to asymmetric information should obey prior distributions recovered approximately from original data. Therefore, we first propose a taxonomy of the latent variable that classifies the latent variable into transferrable (TV) and non-transferable (NTV) variables and further distinguish NTV as the dependent variable (DV) and the independent variable (IV). Next, we propose three latent VAE-based regularizations on TV, DV, and IV to constrain their distributions to well-designed prior distributions to introduce asymmetric information into models and enhance the preservation of shared contents. Finally, we impose the three proposed constraints on a cycle-consistent learning framework, back-translation (BT), named ConstrainedBT. Experiments on three UTGA tasks demonstrate the effectiveness of ConstrainedBT on the information-asymmetric challenge.
更多查看译文
关键词
constraints,text-graph
AI 理解论文
溯源树
样例
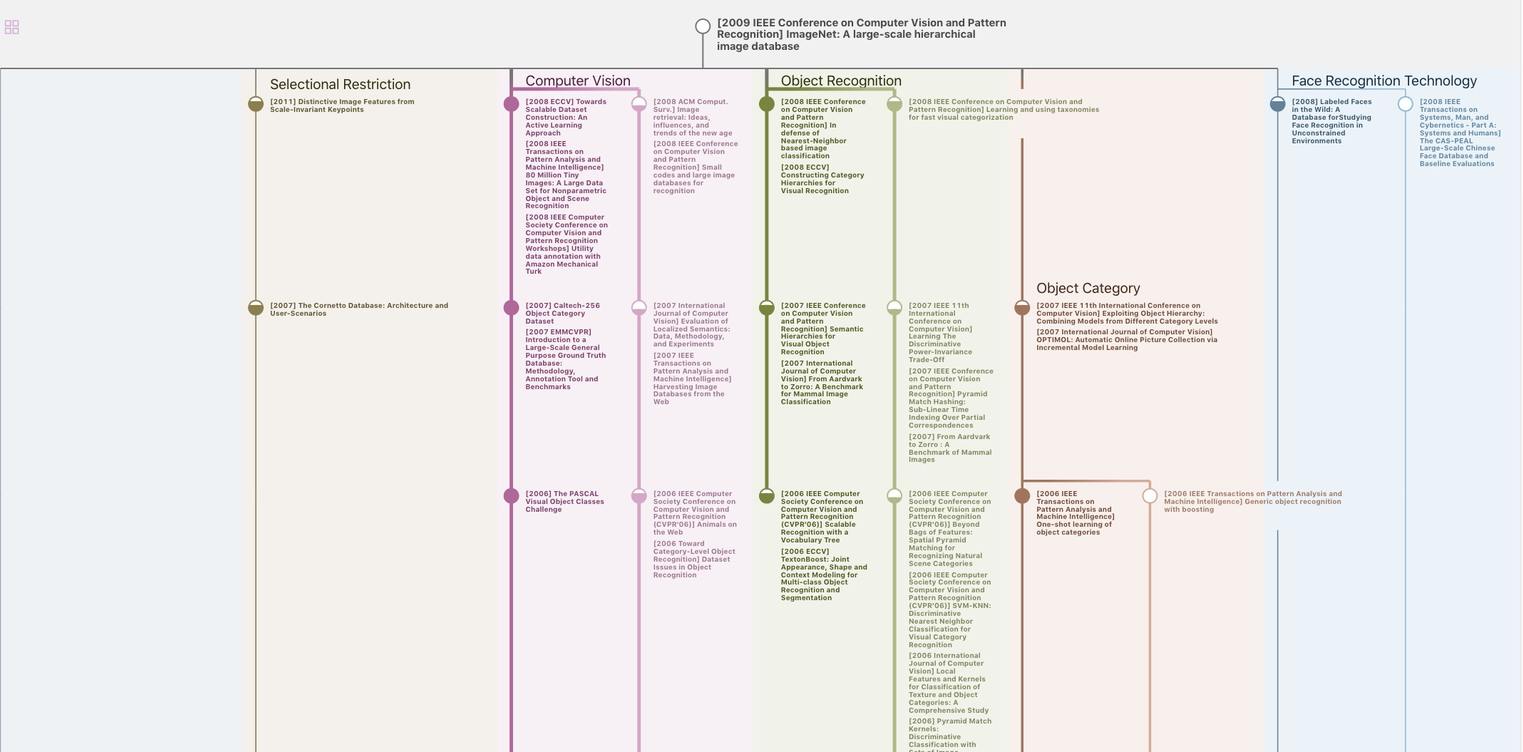
生成溯源树,研究论文发展脉络
Chat Paper
正在生成论文摘要