A Tale of Two Latent Flows: Learning Latent Space Normalizing Flow with Short-Run Langevin Flow for Approximate Inference.
AAAI(2023)
摘要
We study a normalizing flow in the latent space of a top-down generator model, in which the normalizing flow model plays the role of the informative prior model of the generator. We propose to jointly learn the latent space normalizing flow prior model and the top-down generator model by a Markov chain Monte Carlo (MCMC)-based maximum likelihood algorithm, where a short-run Langevin sampling from the intractable posterior distribution is performed to infer the latent variables for each observed example, so that the parameters of the normalizing flow prior and the generator can be updated with the inferred latent variables. We show that, under the scenario of non-convergent short-run MCMC, the finite step Langevin dynamics is a flow-like approximate inference model and the learning objective actually follows the perturbation of the maximum likelihood estimation (MLE). We further point out that the learning framework seeks to (i) match the latent space normalizing flow and the aggregated posterior produced by the short-run Langevin flow, and (ii) bias the model from MLE such that the short-run Langevin flow inference is close to the true posterior. Empirical results of extensive experiments validate the effectiveness of the proposed latent space normalizing flow model in the tasks of image generation, image reconstruction, anomaly detection, supervised image inpainting and unsupervised image recovery.
更多查看译文
关键词
latent space normalizing flows,two latent flows,short-run
AI 理解论文
溯源树
样例
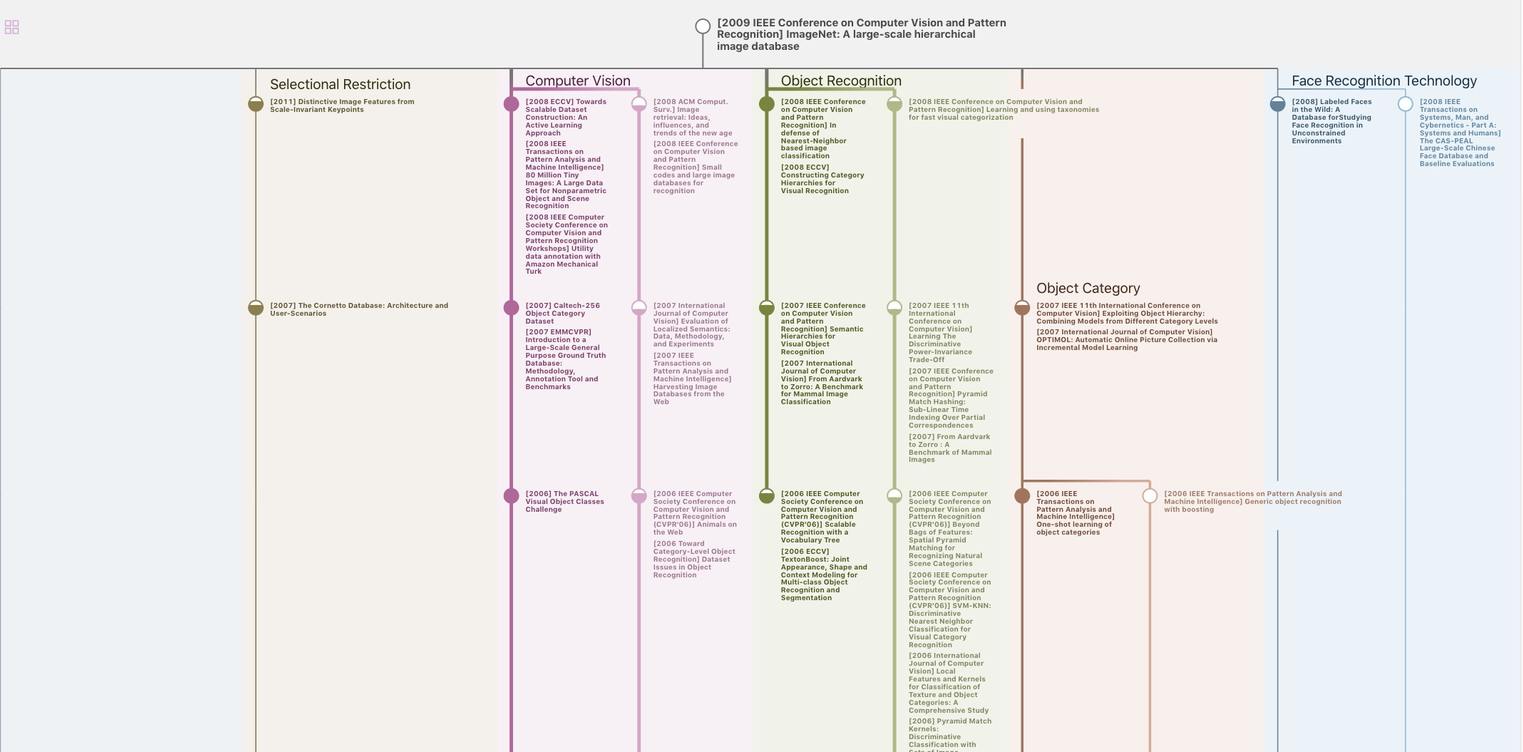
生成溯源树,研究论文发展脉络
Chat Paper
正在生成论文摘要