Inferring Patient Zero on Temporal Networks via Graph Neural Networks
AAAI 2023(2023)
Abstract
The world is currently seeing frequent local outbreaks of epidemics, such as COVID-19 and Monkeypox. Preventing further propagation of the outbreak requires prompt implementation of control measures, and a critical step is to quickly infer patient zero. This backtracking task is challenging for two reasons. First, due to the sudden emergence of local epidemics, information recording the spreading process is limited. Second, the spreading process has strong randomness. To address these challenges, we tailor a gnn-based model to establish the inverse statistical association between the current and initial state implicitly. This model uses contact topology and the current state of the local population to determine the possibility that each individual could be patient zero. We benchmark our model on data from important epidemiological models on five real temporal networks, showing performance significantly superior to previous methods. We also demonstrate that our method is robust to missing information about contact structure or current state. Further, we find the individuals assigned higher inferred possibility by model are closer to patient zero in terms of core number and the activity sequence recording the times at which the individual had contact with other nodes.
MoreTranslated text
Key words
ML: Applications,APP: Healthcare, Medicine & Wellness,APP: Humanities & Computational Social Science,APP: Social Networks,ML: Classification and Regression,ML: Graph-based Machine Learning
AI Read Science
Must-Reading Tree
Example
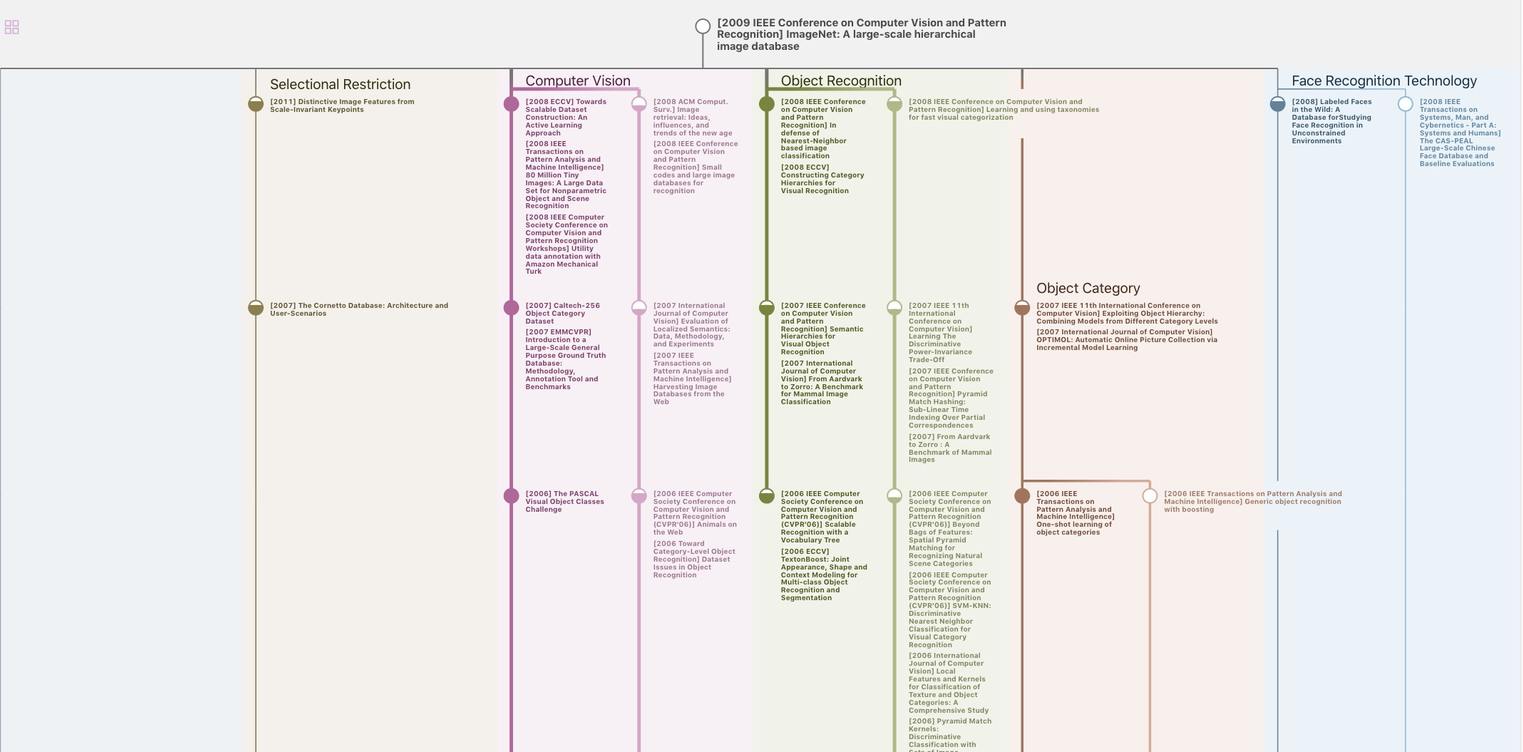
Generate MRT to find the research sequence of this paper
Chat Paper
Summary is being generated by the instructions you defined