Enhancing Conversational Model With Deep Reinforcement Learning and Adversarial Learning.
IEEE Access(2023)
摘要
This paper develops a Chatbot conversational model that is aimed to achieve two goals: 1) utilizing contextual information to generate accurate and relevant responses, and 2) implementing strategies to make conversations human-like. We propose a supervised learning approach for model development and make use of a dataset consisting of multi-turn conversations for model training. In particular, we first develop a module based on deep reinforcement learning to maximize the utilization of contextual information serving as insurance for accurate response generation. Then, we incorporate the response generation process into an adversarial learning framework so as to make the generated response more human-like. Using these two phases in combination eventually results in a unified model that generates semantically appropriate responses that are also expressed naturally as human-generated in the conversation. We conducted various experiments and obtained a significant improvement compared to the baseline and other related studies.
更多查看译文
关键词
BERT, chatbot, reinforcement learning, sequence to sequence, generative adversarial nets
AI 理解论文
溯源树
样例
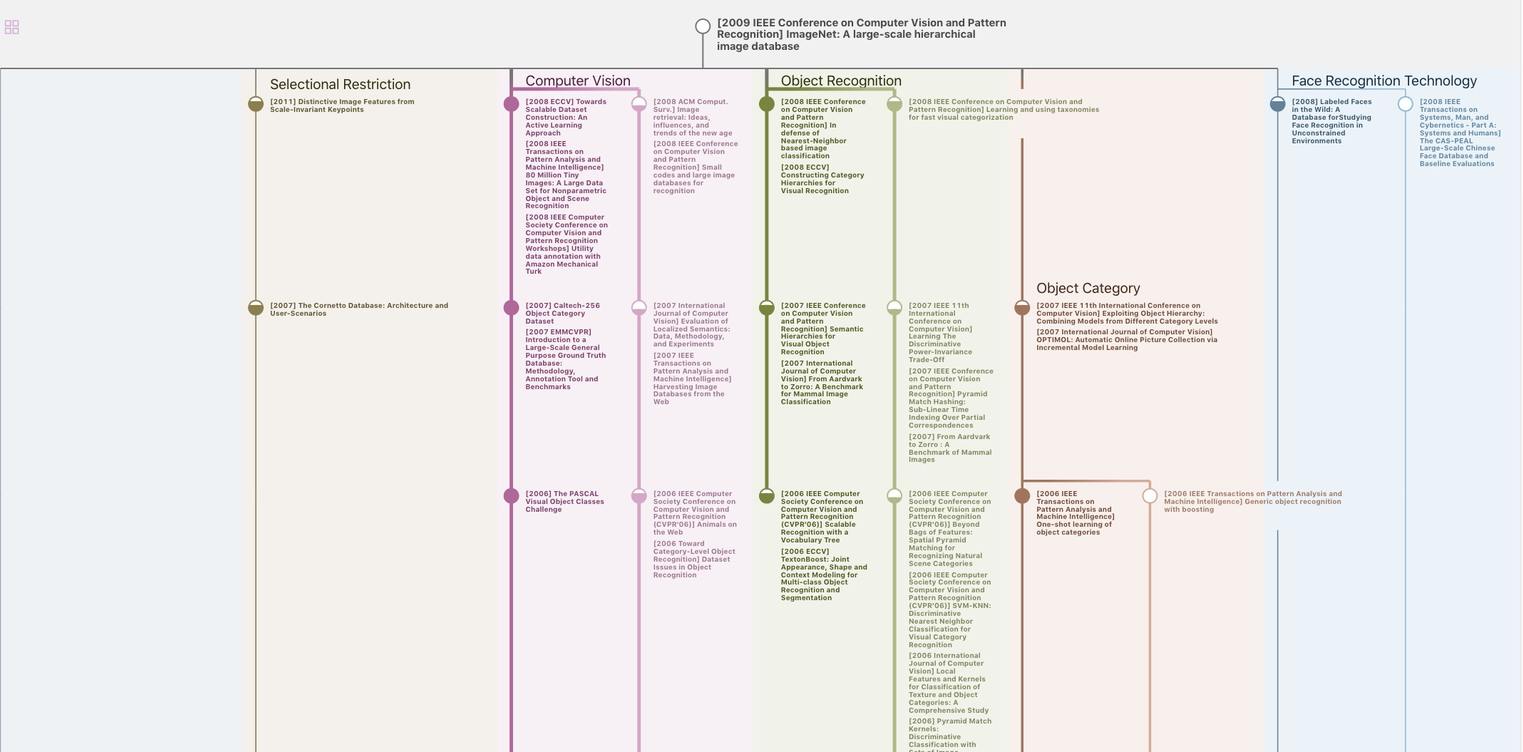
生成溯源树,研究论文发展脉络
Chat Paper
正在生成论文摘要