The Capability of Large Language Models to Measure Psychiatric Functioning
CoRR(2023)
摘要
The current work investigates the capability of Large language models (LLMs) that are explicitly trained on large corpuses of medical knowledge (Med-PaLM 2) to predict psychiatric functioning from patient interviews and clinical descriptions without being trained to do so. To assess this, n = 145 depression and n =115 PTSD assessments and n = 46 clinical case studies across high prevalence/high comorbidity disorders (Depressive, Anxiety, Psychotic, trauma and stress, Addictive disorders) were analyzed using prompts to extract estimated clinical scores and diagnoses. Results demonstrate that Med-PaLM 2 is capable of assessing psychiatric functioning across a range of psychiatric conditions with the strongest performance being the prediction of depression scores based on standardized assessments (Accuracy range= 0.80 - 0.84) which were statistically indistinguishable from human clinical raters t(1,144) = 1.20; p = 0.23. Results show the potential for general clinical language models to flexibly predict psychiatric risk based on free descriptions of functioning from both patients and clinicians.
更多查看译文
关键词
large language models,psychiatric
AI 理解论文
溯源树
样例
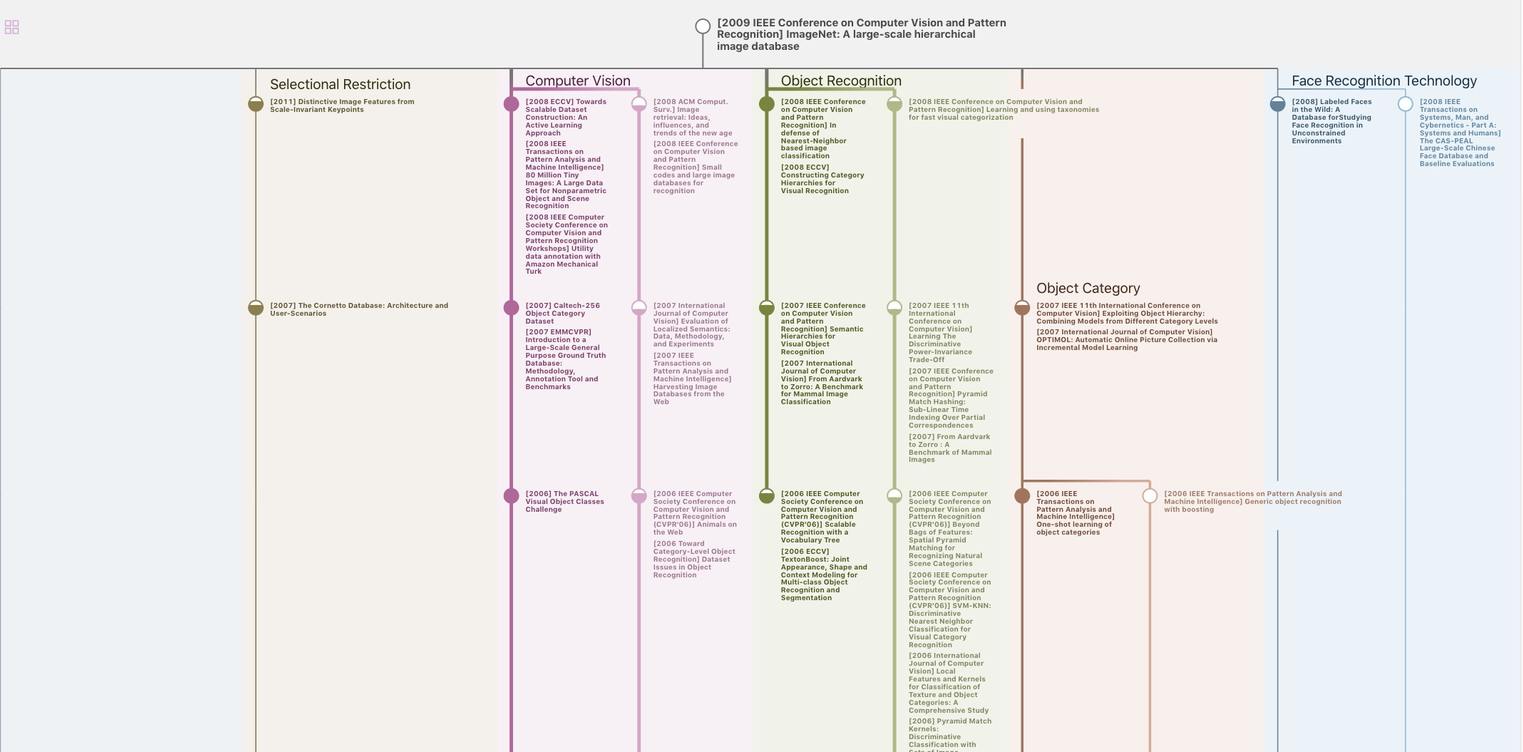
生成溯源树,研究论文发展脉络
Chat Paper
正在生成论文摘要