Prediction of resilient modulus of fine-grained soil for pavement design using KNN, MARS, and random forest techniques
Arabian Journal of Geosciences(2023)
摘要
This study was motivated by the difficulty in determining the resilient modulus of soils using the repeated load triaxial test (RLTT) recommended by the mechanistic-empirical pavement design guide (MEPDG). An alternative means to estimate the resilient modulus of fine-grained soils has been established in the form of three models that were developed using three supervised machine-learning techniques. This includes k -nearest neighbor (KNN), multivariate adaptive regression splines (MARS), and random forest. The data utilized for the development of the models were sourced from the long-term pavement performance (LTPP) database domiciled in the Infopave database in the USA. A total of twelve routine soil properties that have significant influence on the resilient modulus of fine-grained soils were considered in this study. Results obtained from this study revealed that the three developed models (KNN, MARS, and random forest) had high prediction accuracy and high generalization ability. However, the random forest model, based on the statistical indices used to evaluate the models, gave the best prediction accuracy ( R 2 = 0.9312 for the testing dataset) of the three developed model. It was followed closely by the MARS model with an R 2 value of 0.9057. The last model in terms of prediction accuracy was the KNN model with an R 2 value of 0.8748. Furthermore, based on parameter significance assessment using the random forest model, it was revealed that the nominal maximum axial stress and confining pressure are the best predictor variables for the estimation of the resilient modulus of fine-grained soils.
更多查看译文
关键词
Flexible pavement design,Hyperparameters,Mechanistic-empirical pavement design guide,Repeated load triaxial test,Supervised artificial intelligence techniques
AI 理解论文
溯源树
样例
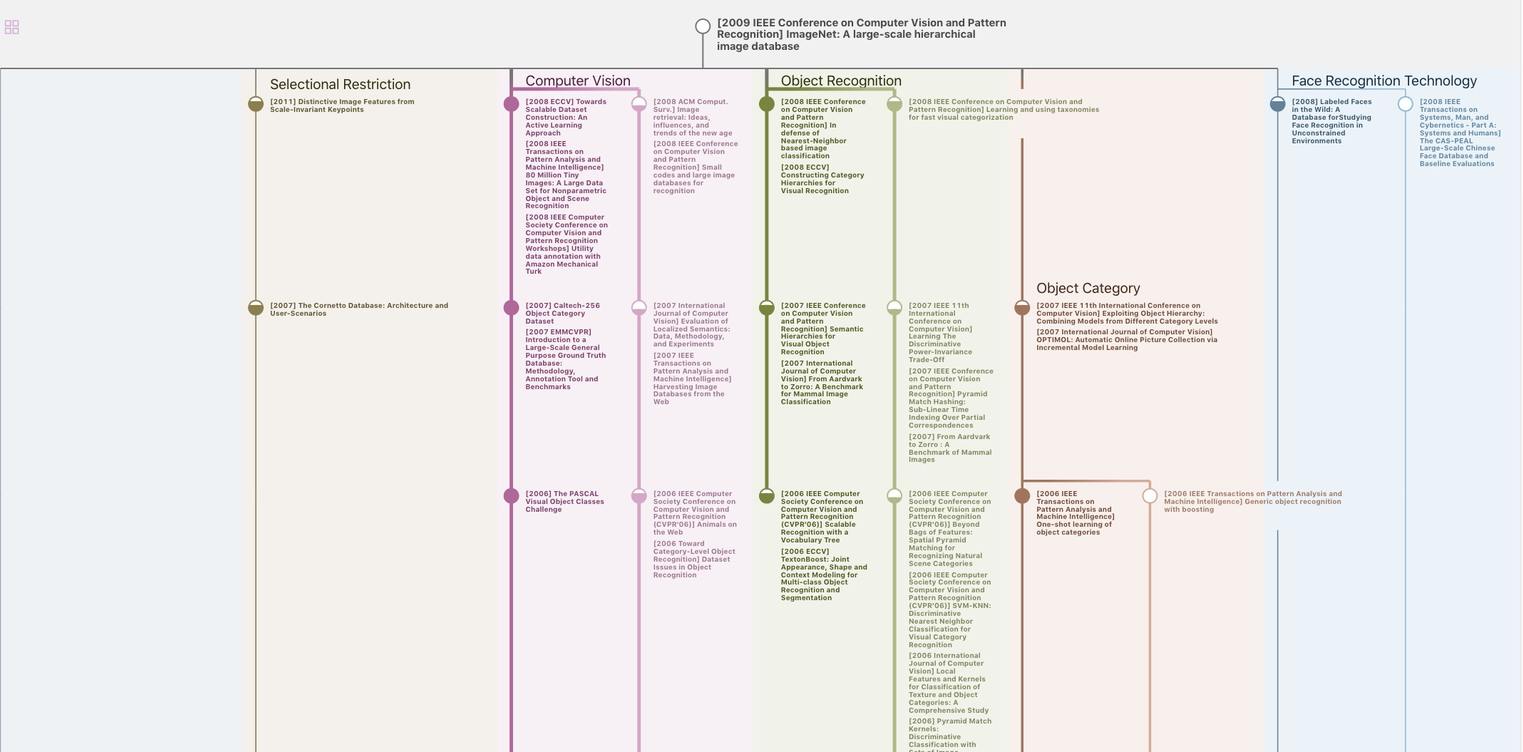
生成溯源树,研究论文发展脉络
Chat Paper
正在生成论文摘要