LK-IB: a hybrid framework with legal knowledge injection for compulsory measure prediction
Artificial Intelligence and Law(2023)
摘要
The interpretability of AI is just as important as its performance. In the LegalAI field, there have been efforts to enhance the interpretability of models, but a trade-off between interpretability and prediction accuracy remains inevitable. In this paper, we introduce a novel framework called LK-IB for compulsory measure prediction (CMP), one of the critical tasks in LegalAI. LK-IB leverages Legal Knowledge and combines an Interpretable model and a Black-box model to balance interpretability and prediction performance. Specifically, LK-IB involves three steps: (1) inputting cases into the first module, where first-order logic (FOL) rules are used to make predictions and output them directly if possible; (2) sending cases to the second module if FOL rules are not applicable, where a case distributor categorizes them as either “simple” or “complex“; and (3) sending simple cases to an interpretable model with strong interpretability and complex cases to a black-box model with outstanding performance. Experimental results demonstrate that the LK-IB framework provides more interpretable and accurate predictions than other state-of-the-art models. Given that the majority of cases in LegalAI are simple, the idea of model combination has significant potential for practical applications.
更多查看译文
关键词
Legal knowledge,Model combination,Compulsory measure prediction,Interpretability
AI 理解论文
溯源树
样例
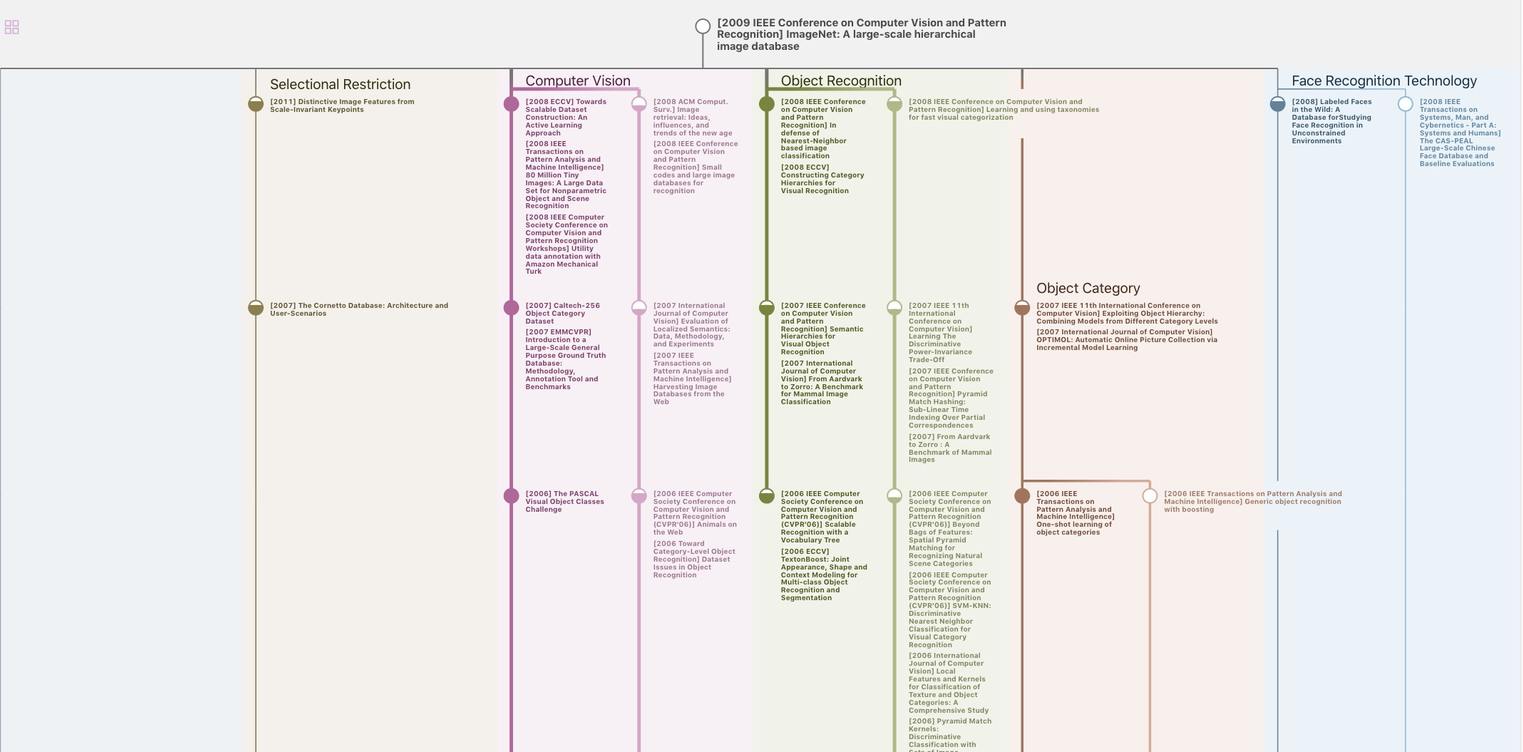
生成溯源树,研究论文发展脉络
Chat Paper
正在生成论文摘要