Interpolating Nonadiabatic Molecular Dynamics Hamiltonian with Bidirectional Long Short-Term Memory Networks.
The journal of physical chemistry letters(2023)
摘要
Essential for understanding far-from-equilibrium processes, nonadiabatic (NA) molecular dynamics (MD) requires expensive calculations of the excitation energies and NA couplings. Machine learning (ML) can simplify computation; however, the NA Hamiltonian requires complex ML models due to its intricate relationship to atomic geometry. Working directly in the time domain, we employ bidirectional long short-term memory networks (Bi-LSTM) to interpolate the Hamiltonian. Applying this multiscale approach to three metal-halide perovskite systems, we achieve two orders of magnitude computational savings compared to direct ab initio calculation. Reasonable charge trapping and recombination times are obtained with NA Hamiltonian sampling every half a picosecond. The Bi-LSTM-NAMD method outperforms earlier models and captures both slow and fast time scales. In combination with ML force fields, the methodology extends NAMD simulation times from picoseconds to nanoseconds, comparable to charge carrier lifetimes in many materials. Nanosecond sampling is particularly important in systems containing defects, boundaries, interfaces, etc. that can undergo slow rearrangements.
更多查看译文
关键词
nonadiabatic molecular dynamics hamiltonian,memory,short-term
AI 理解论文
溯源树
样例
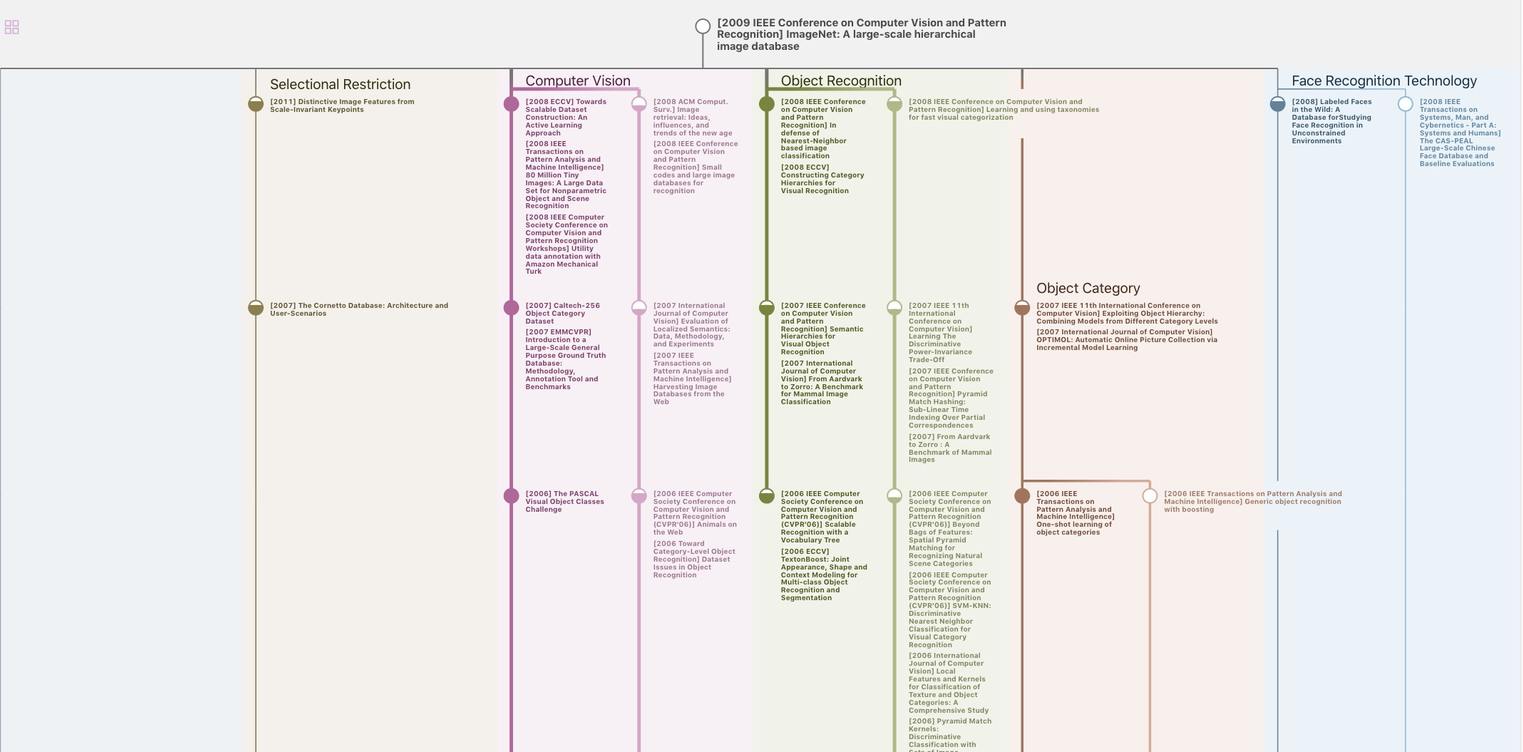
生成溯源树,研究论文发展脉络
Chat Paper
正在生成论文摘要