Spatially Varying Prior Learning for Blind Hyperspectral Image Fusion.
IEEE transactions on image processing : a publication of the IEEE Signal Processing Society(2023)
摘要
In recent years, researchers have become more interested in hyperspectral image fusion (HIF) as a potential alternative to expensive high-resolution hyperspectral imaging systems, which aims to recover a high-resolution hyperspectral image (HR-HSI) from two images obtained from low-resolution hyperspectral (LR-HSI) and high-spatial-resolution multispectral (HR-MSI). It is generally assumed that degeneration in both the spatial and spectral domains is known in traditional model-based methods or that there existed paired HR-LR training data in deep learning-based methods. However, such an assumption is often invalid in practice. Furthermore, most existing works, either introducing hand-crafted priors or treating HIF as a black-box problem, cannot take full advantage of the physical model. To address those issues, we propose a deep blind HIF method by unfolding model-based maximum a posterior (MAP) estimation into a network implementation in this paper. Our method works with a Laplace distribution (LD) prior that does not need paired training data. Moreover, we have developed an observation module to directly learn degeneration in the spatial domain from LR-HSI data, addressing the challenge of spatially-varying degradation. We also propose to learn the uncertainty (mean and variance) of LD models using a novel Swin-Transformer-based denoiser and to estimate the variance of degraded images from residual errors (rather than treating them as global scalars). All parameters of the MAP estimation algorithm and the observation module can be jointly optimized through end-to-end training. Extensive experiments on both synthetic and real datasets show that the proposed method outperforms existing competing methods in terms of both objective evaluation indexes and visual qualities.
更多查看译文
关键词
blind hyperspectral image fusion,prior learning
AI 理解论文
溯源树
样例
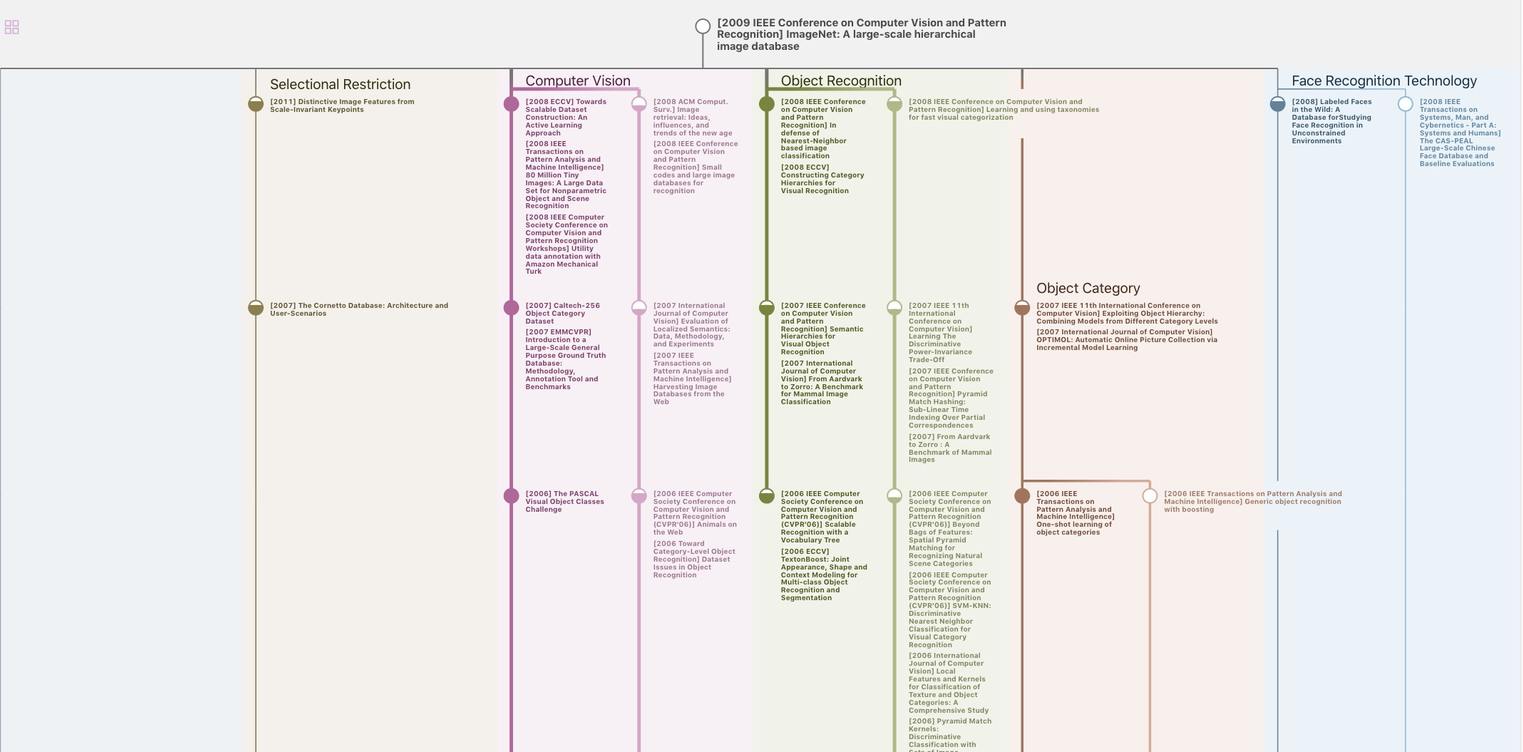
生成溯源树,研究论文发展脉络
Chat Paper
正在生成论文摘要