Structured Knowledge Distillation for Accurate and Efficient Object Detection
IEEE TRANSACTIONS ON PATTERN ANALYSIS AND MACHINE INTELLIGENCE(2023)
摘要
Knowledge distillation, which aims to transfer the knowledge learned by a cumbersome teacher model to a lightweight student model, has become one of the most popular and effective techniques in computer vision. However, many previous knowledge distillation methods are designed for image classification and fail in more challenging tasks such as object detection. In this paper, we first suggest that the failure of knowledge distillation on object detection is mainly caused by two reasons: (1) the imbalance between pixels of foreground and background and (2) lack of knowledge distillation on the relation among different pixels. Then, we propose a structured knowledge distillation scheme, including attention-guided distillation and non-local distillation to address the two issues, respectively. Attention-guided distillation is proposed to find the crucial pixels of foreground objects with an attention mechanism and then make the students take more effort to learn their features. Non-local distillation is proposed to enable students to learn not only the feature of an individual pixel but also the relation between different pixels captured by non-local modules. Experimental results have demonstrated the effectiveness of our method on thirteen kinds of object detection models with twelve comparison methods for both object detection and instance segmentation. For instance, Faster RCNN with our distillation achieves 43.9 mAP on MS COCO2017, which is 4.1 higher than the baseline. Additionally, we show that our method is also beneficial to the robustness and domain generalization ability of detectors. Codes and model weights have been released on GitHub(1).
更多查看译文
关键词
Attention,instance segmentation,knowledge distillation,model acceleration and compression,non-local module,object detection,student-teacher learning
AI 理解论文
溯源树
样例
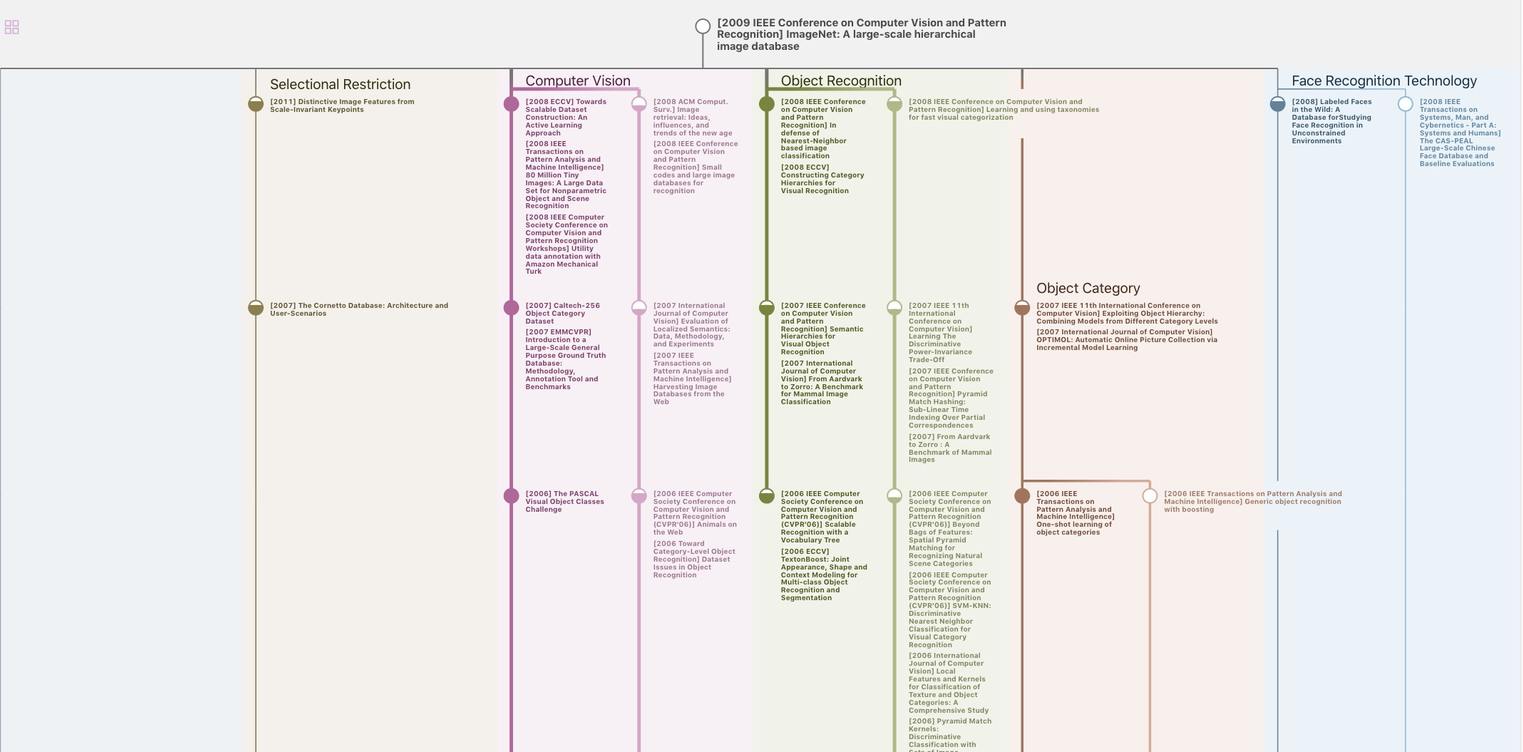
生成溯源树,研究论文发展脉络
Chat Paper
正在生成论文摘要