Personalized Category Frequency prediction for Buy It Again recommendations
PROCEEDINGS OF THE 17TH ACM CONFERENCE ON RECOMMENDER SYSTEMS, RECSYS 2023(2023)
摘要
Buy It Again (BIA) recommendations are crucial to retailers to help improve user experience and site engagement by suggesting items that customers are likely to buy again based on their own repeat purchasing patterns. Most existing BIA studies analyze guests' personalized behaviour at item granularity. This finer level of granularity might be appropriate for small businesses or small datasets for search purposes. However, this approach can be infeasible for big retailers which have hundreds of millions of guests and tens of millions of items. For such data sets, it is more practical to have a coarse-grained model that captures customer behaviour at the item category level. In addition, customers commonly explore variants of items within the same categories, e.g., trying different brands or flavors of yogurt. A category-based model may be more appropriate in such scenarios. We propose a recommendation system called a hierarchical PCIC model that consists of a personalized category model (PC model) and a personalized item model within categories (IC model). PC model generates a personalized list of categories that customers are likely to purchase again. IC model ranks items within categories that guests are likely to reconsume within a category. The hierarchical PCIC model captures the general consumption rate of products using survival models. Trends in consumption are captured using time series models. Features derived from these models are used in training a category-grained neural network. We compare PCIC to twelve existing baselines on four standard open datasets. PCIC improves NDCG up to 16% while improving recall by around 2%. We were able to scale and train (over 8 hours) PCIC on a large dataset of 100M guests and 3M items where repeat categories of a guest outnumber repeat items. PCIC was deployed and A/B tested on the site of a major retailer, leading to significant gains in guest engagement.
更多查看译文
关键词
Personalization,Recommender Systems,E-commerce,Repeat purchases,Buy it again,Survival Models,Time-Series Models,Neural Network
AI 理解论文
溯源树
样例
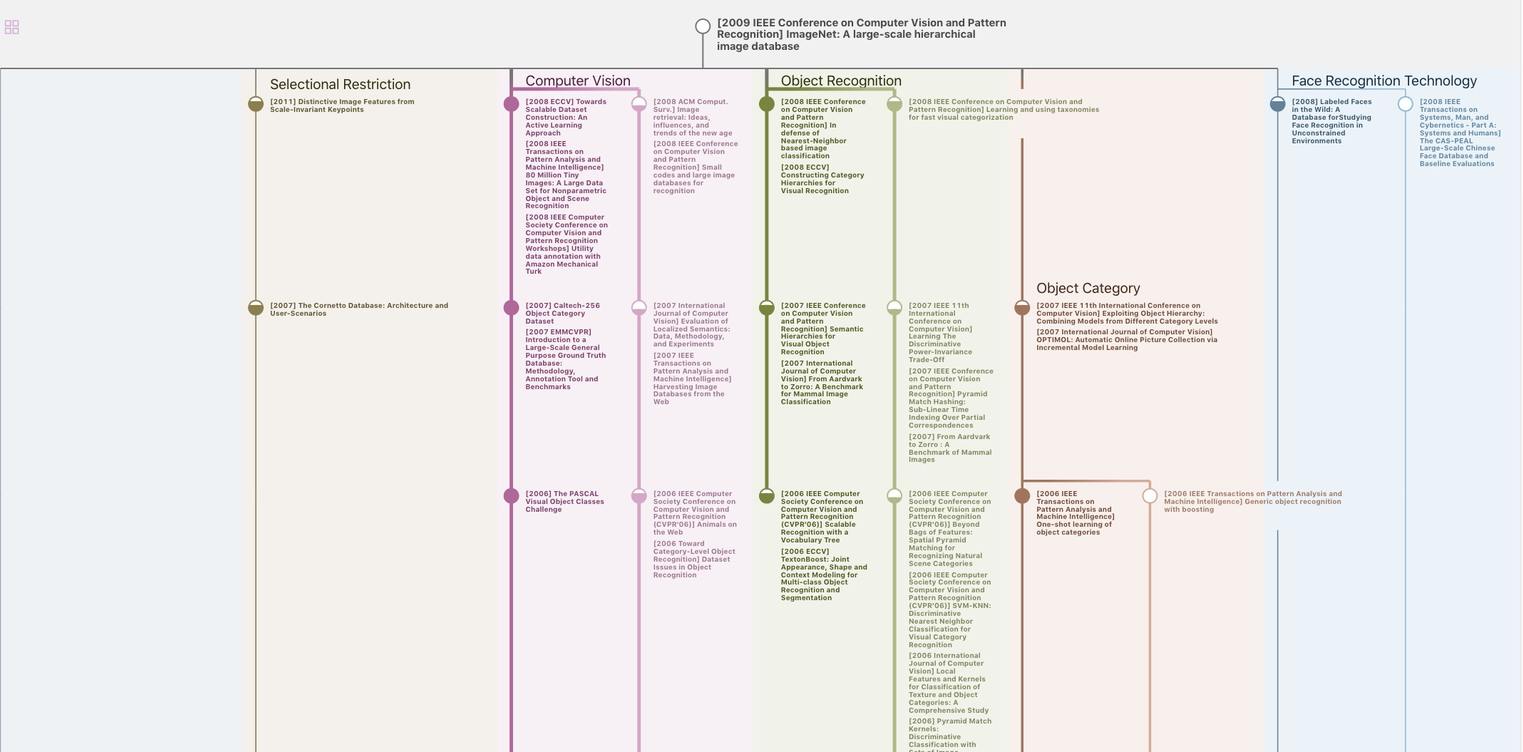
生成溯源树,研究论文发展脉络
Chat Paper
正在生成论文摘要