Generative Noisy-Label Learning by Implicit Dicriminative Approximation with Partial Label Prior
CoRR(2023)
摘要
The learning with noisy labels has been addressed with both discriminative and generative models. Although discriminative models have dominated the field due to their simpler modeling and more efficient computational training processes, generative models offer a more effective means of disentangling clean and noisy labels and improving the estimation of the label transition matrix. However, generative approaches maximize the joint likelihood of noisy labels and data using a complex formulation that only indirectly optimizes the model of interest associating data and clean labels. Additionally, these approaches rely on generative models that are challenging to train and tend to use uninformative clean label priors. In this paper, we propose a new generative noisy-label learning approach that addresses these three issues. First, we propose a new model optimisation that directly associates data and clean labels. Second, the generative model is implicitly estimated using a discriminative model, eliminating the inefficient training of a generative model. Third, we propose a new informative label prior inspired by partial label learning as supervision signal for noisy label learning. Extensive experiments on several noisy-label benchmarks demonstrate that our generative model provides state-of-the-art results while maintaining a similar computational complexity as discriminative models.
更多查看译文
关键词
partial noisy-label prior,implicit dicriminative approximation
AI 理解论文
溯源树
样例
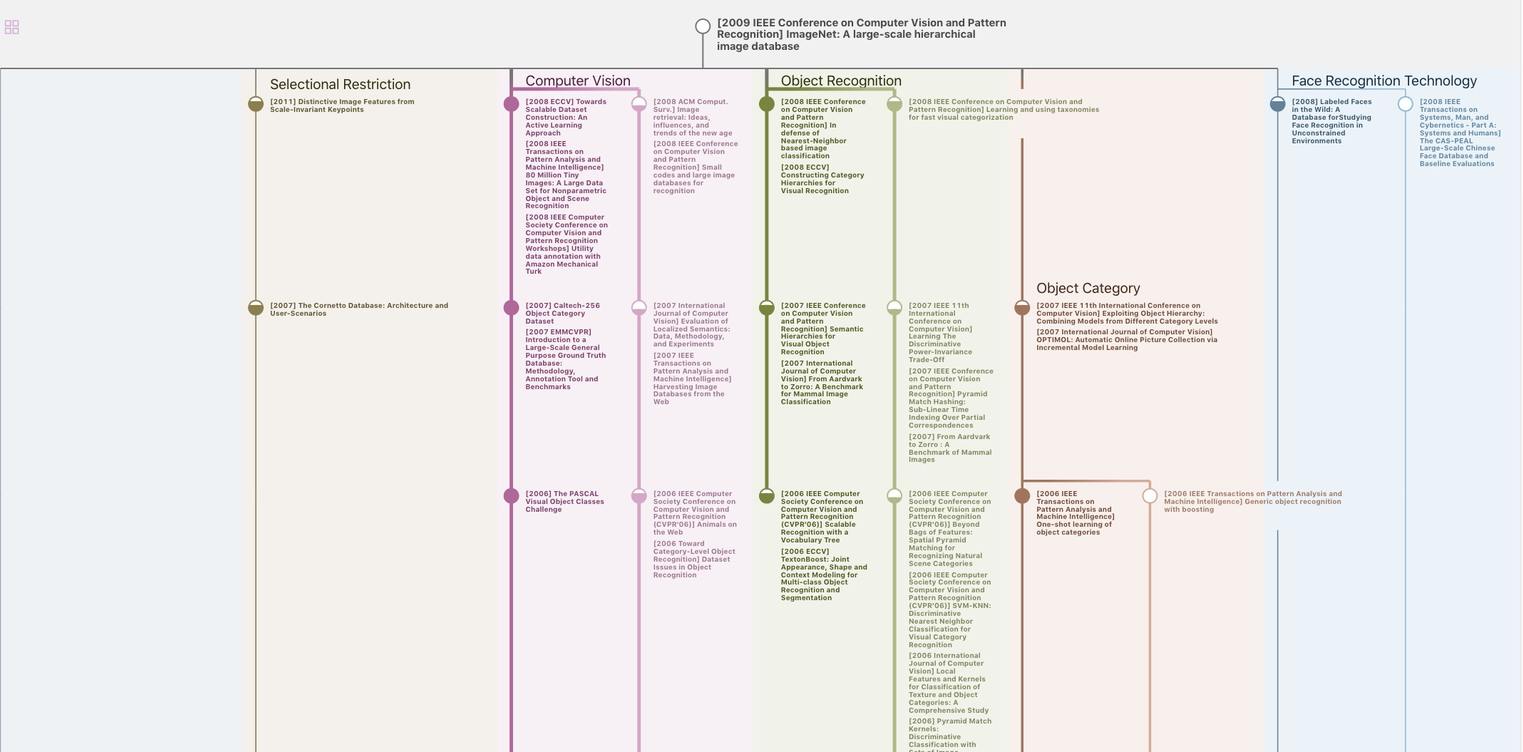
生成溯源树,研究论文发展脉络
Chat Paper
正在生成论文摘要