Integrating Homomorphic Encryption and Trusted Execution Technology for Autonomous and Confidential Model Refining in Cloud
CoRR(2023)
摘要
With the popularity of cloud computing and machine learning, it has been a trend to outsource machine learning processes (including model training and model-based inference) to cloud. By the outsourcing, other than utilizing the extensive and scalable resource offered by the cloud service provider, it will also be attractive to users if the cloud servers can manage the machine learning processes autonomously on behalf of the users. Such a feature will be especially salient when the machine learning is expected to be a long-term continuous process and the users are not always available to participate. Due to security and privacy concerns, it is also desired that the autonomous learning preserves the confidentiality of users' data and models involved. Hence, in this paper, we aim to design a scheme that enables autonomous and confidential model refining in cloud. Homomorphic encryption and trusted execution environment technology can protect confidentiality for autonomous computation, but each of them has their limitations respectively and they are complementary to each other. Therefore, we further propose to integrate these two techniques in the design of the model refining scheme. Through implementation and experiments, we evaluate the feasibility of our proposed scheme. The results indicate that, with our proposed scheme the cloud server can autonomously refine an encrypted model with newly provided encrypted training data to continuously improve its accuracy. Though the efficiency is still significantly lower than the baseline scheme that refines plaintext-model with plaintext-data, we expect that it can be improved by fully utilizing the higher level of parallelism and the computational power of GPU at the cloud server.
更多查看译文
关键词
Autonomous Model Refining,Confidentiality,Homomorphic Encryption,Trusted Execution Environment
AI 理解论文
溯源树
样例
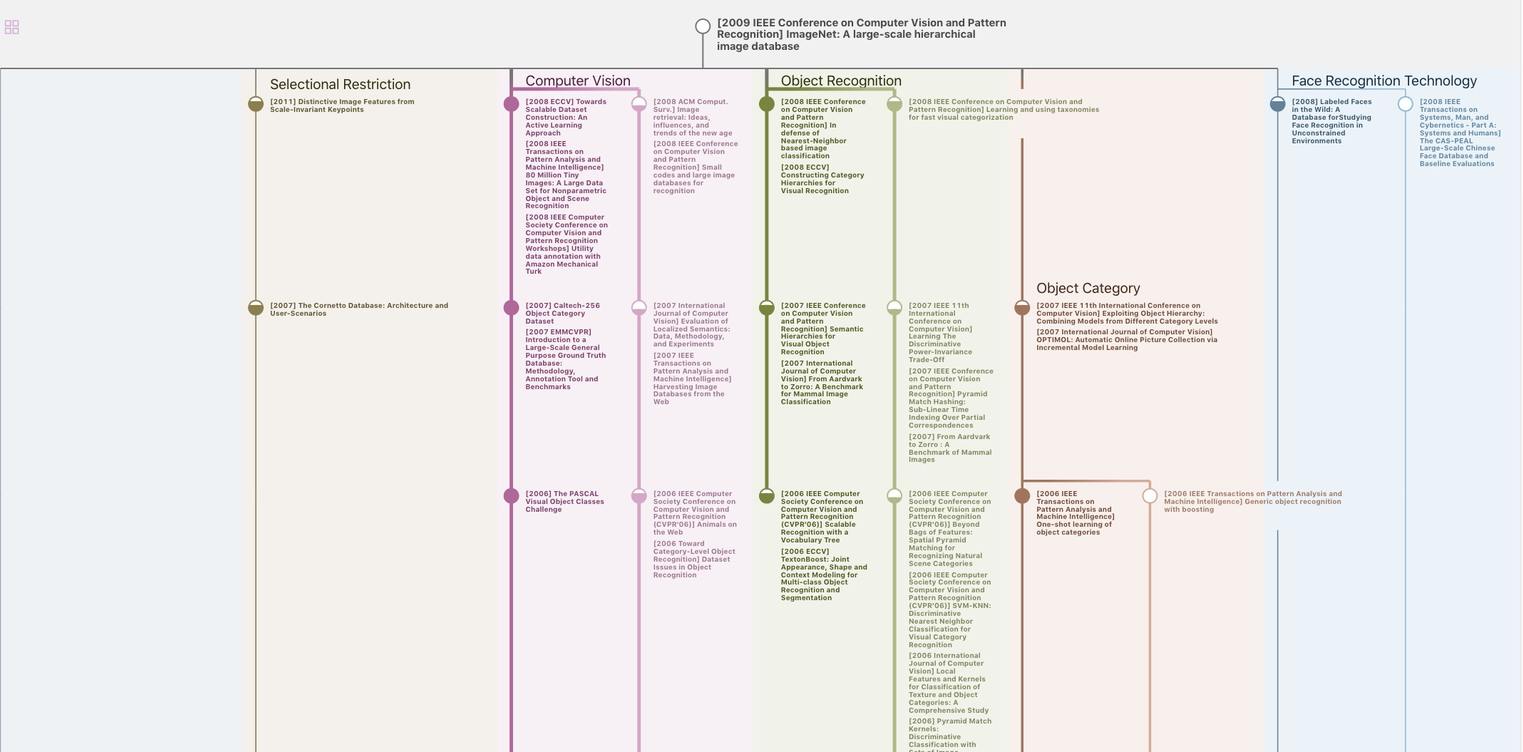
生成溯源树,研究论文发展脉络
Chat Paper
正在生成论文摘要