Differentially Private Linear Regression with Linked Data
CoRR(2023)
摘要
There has been increasing demand for establishing privacy-preserving methodologies for modern statistics and machine learning. Differential privacy, a mathematical notion from computer science, is a rising tool offering robust privacy guarantees. Recent work focuses primarily on developing differentially private versions of individual statistical and machine learning tasks, with nontrivial upstream pre-processing typically not incorporated. An important example is when record linkage is done prior to downstream modeling. Record linkage refers to the statistical task of linking two or more data sets of the same group of entities without a unique identifier. This probabilistic procedure brings additional uncertainty to the subsequent task. In this paper, we present two differentially private algorithms for linear regression with linked data. In particular, we propose a noisy gradient method and a sufficient statistics perturbation approach for the estimation of regression coefficients. We investigate the privacy-accuracy tradeoff by providing finite-sample error bounds for the estimators, which allows us to understand the relative contributions of linkage error, estimation error, and the cost of privacy. The variances of the estimators are also discussed. We demonstrate the performance of the proposed algorithms through simulations and an application to synthetic data.
更多查看译文
关键词
private linear regression
AI 理解论文
溯源树
样例
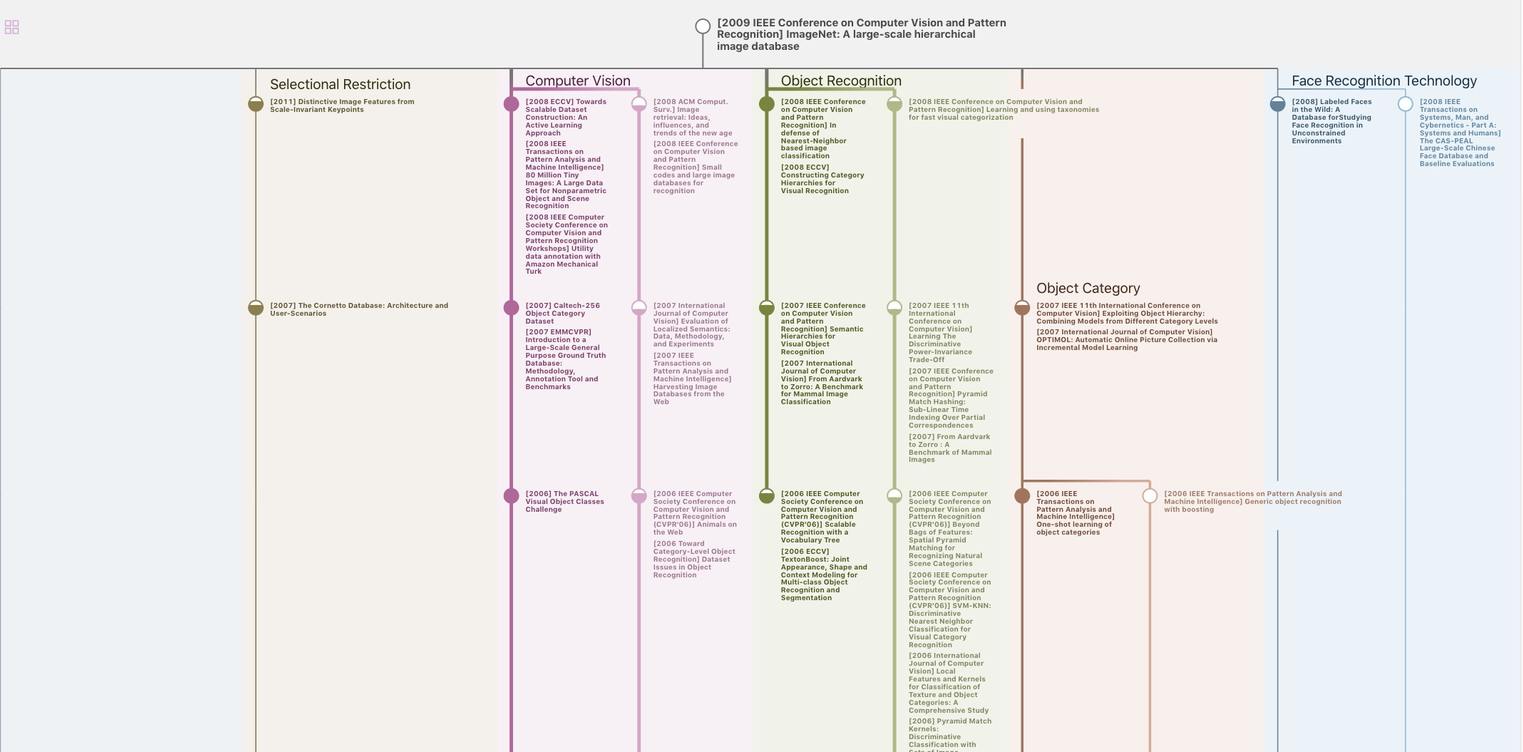
生成溯源树,研究论文发展脉络
Chat Paper
正在生成论文摘要