Evolutionary Multitasking for Optimization Based on Generative Strategies
IEEE Transactions on Evolutionary Computation(2023)
摘要
Evolutionary multitasking (EMT) is one of the emerging topics in evolutionary computation. EMT can solve multiple related optimization tasks simultaneously and enhance the optimization of each task via knowledge sharing among tasks. Many EMT algorithms have been proposed and achieved success in various problems, yet EMT for multiobjective optimization remains a big challenge. The existing multiobjective EMT algorithms tend to suffer from slow convergence and difficulty in generating high-quality knowledge. To alleviate these issues, this article proposes a new EMT algorithm, namely, EMT-GS for multiobjective optimization based on two generative strategies. Particularly, generative adversarial networks (GANs) and inertial differential evolution (IDE) are introduced to generate transferable knowledge and offspring, respectively. A GAN is trained periodically for each source-target task pair, based on which helpful knowledge is generated from the source task and transferred to boost the solving of the target task. To accelerate the population convergence, the IDE strategy is put forward to generate offspring in a promising direction according to the individuals from the previous generation and the transferred knowledge. The performance of EMT-GS is validated on three multitasking multiobjective benchmark problems. The experimental results highlight the excellent competitiveness of EMT-GS compared to other state-of-the-art multiobjective EMT algorithms.
更多查看译文
关键词
generative strategies,optimization
AI 理解论文
溯源树
样例
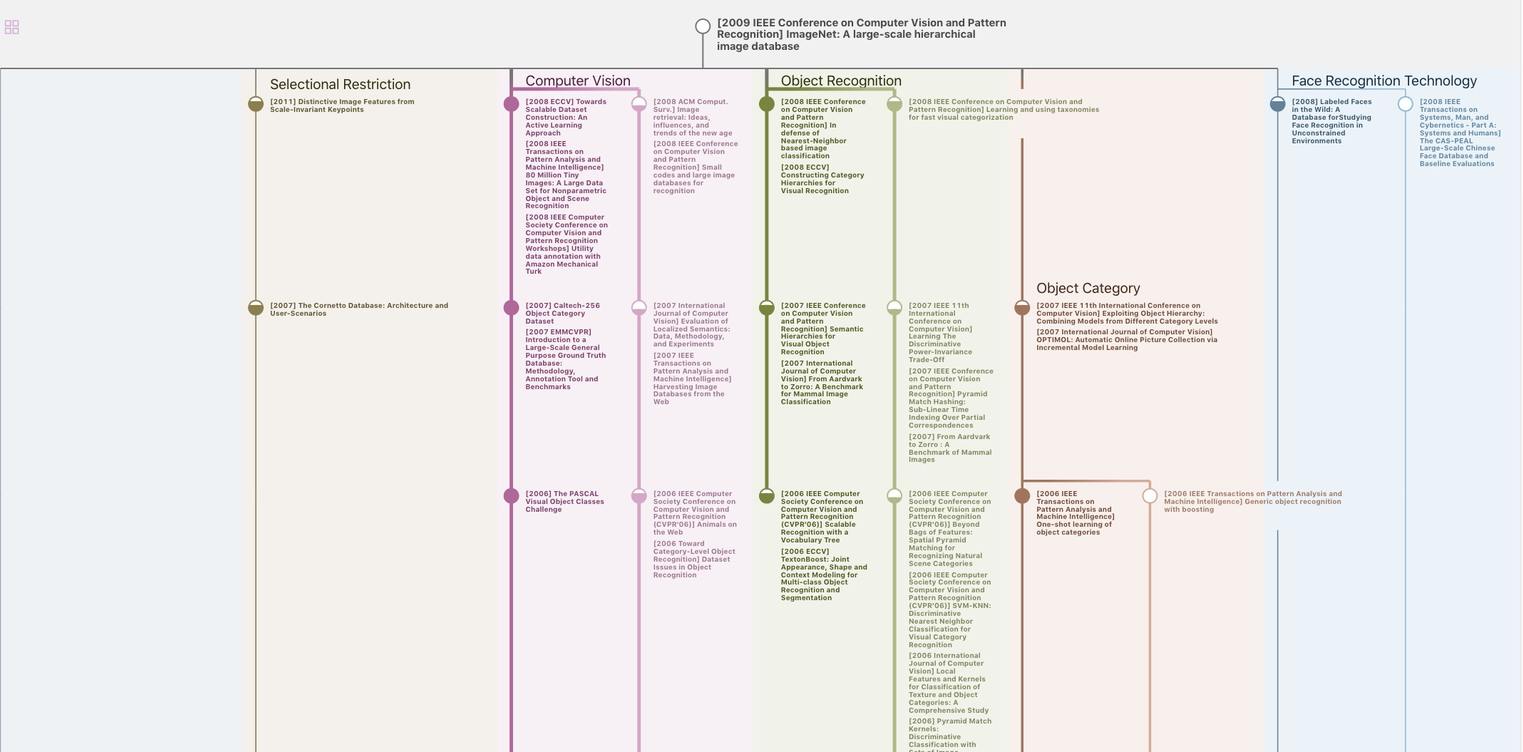
生成溯源树,研究论文发展脉络
Chat Paper
正在生成论文摘要