Geometric deep learning as a potential tool for antimicrobial peptide prediction.
Frontiers in bioinformatics(2023)
摘要
Antimicrobial peptides (AMPs) are components of natural immunity against invading pathogens. They are polymers that fold into a variety of three-dimensional structures, enabling their function, with an underlying sequence that is best represented in a non-flat space. The structural data of AMPs exhibits non-Euclidean characteristics, which means that certain properties, e.g., differential manifolds, common system of coordinates, vector space structure, or translation-equivariance, along with basic operations like convolution, in non-Euclidean space are not distinctly established. Geometric deep learning (GDL) refers to a category of machine learning methods that utilize deep neural models to process and analyze data in non-Euclidean settings, such as graphs and manifolds. This emerging field seeks to expand the use of structured models to these domains. This review provides a detailed summary of the latest developments in designing and predicting AMPs utilizing GDL techniques and also discusses both current research gaps and future directions in the field.
更多查看译文
关键词
geometric deep learning,deep learning
AI 理解论文
溯源树
样例
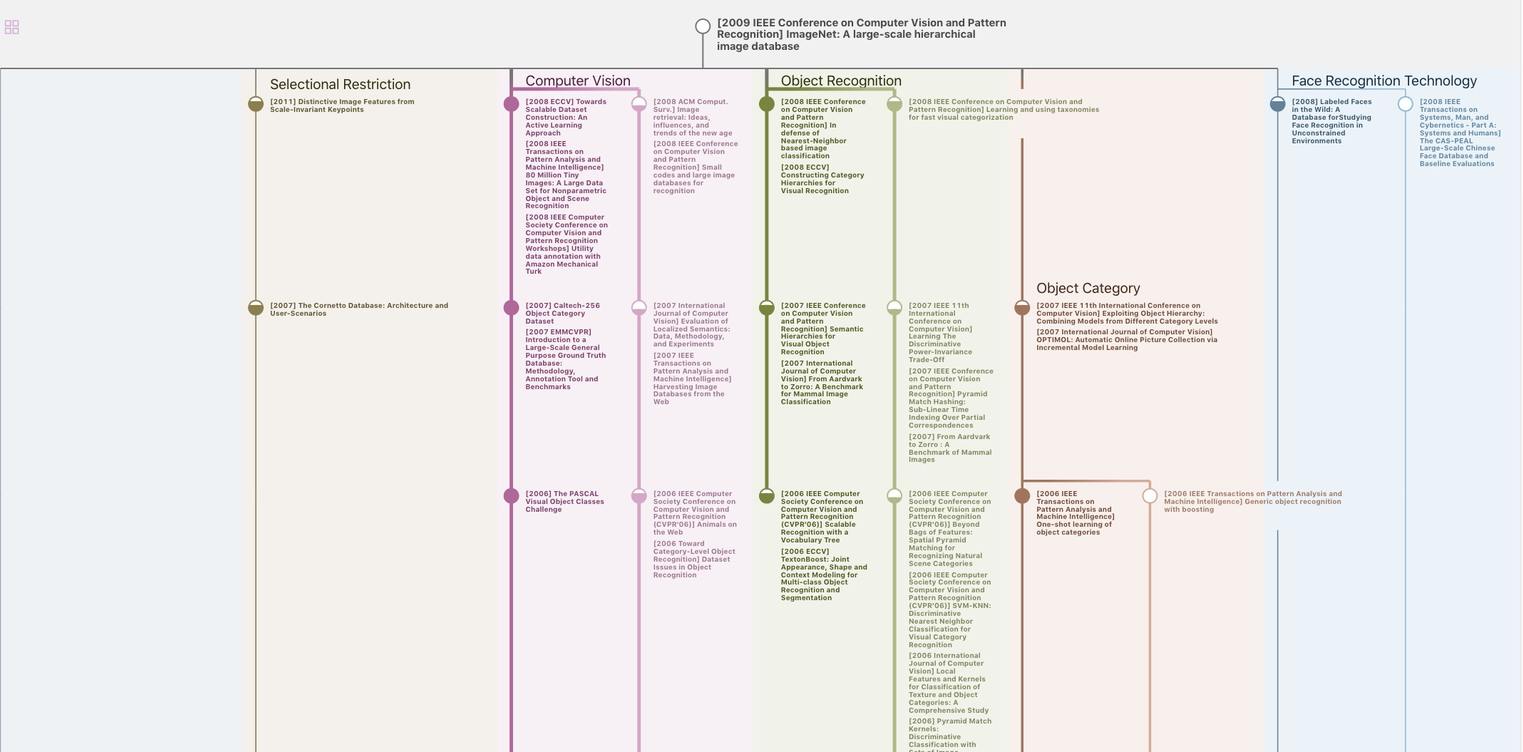
生成溯源树,研究论文发展脉络
Chat Paper
正在生成论文摘要