SF-IDS: An Imbalanced Semi-Supervised Learning Framework for Fine-grained Intrusion Detection
ICC 2023 - IEEE INTERNATIONAL CONFERENCE ON COMMUNICATIONS(2023)
摘要
Deep learning-based fine-grained network intrusion detection systems (NIDS) enable different attacks to be responded to in a fast and targeted manner with the help of large-scale labels. However, the cost of labeling causes insufficient labeled samples. Also, the real fine-grained traffic shows a long-tailed distribution with great class imbalance. These two problems often appear simultaneously, posing serious challenges to fine-grained NIDS. In this work, we propose a novel semi-supervised fine-grained intrusion detection framework, SF-IDS, to achieve attack classification in the label-limited and highly class imbalanced case. We design a self-training backbone model called RI-1DCNN to boost the feature extraction by reconstructing the input samples into a multichannel image format. The uncertainty of the generated pseudo-labels is evaluated and used as a reference for pseudo-label filtering in combination with the prediction probability. To mitigate the effects of fine-grained class imbalance, we propose a hybrid loss function combining supervised contrastive loss and multi-weighted classification loss to obtain more compact intra-class features and clearer interclass intervals. Experiments show that the proposed SF-IDS achieves 3.01% and 2.71% Marco-F1 improvement on two classical datasets with 1% labeled, respectively.
更多查看译文
关键词
Intrusion detection,semi-supervised learning,imbalanced classification
AI 理解论文
溯源树
样例
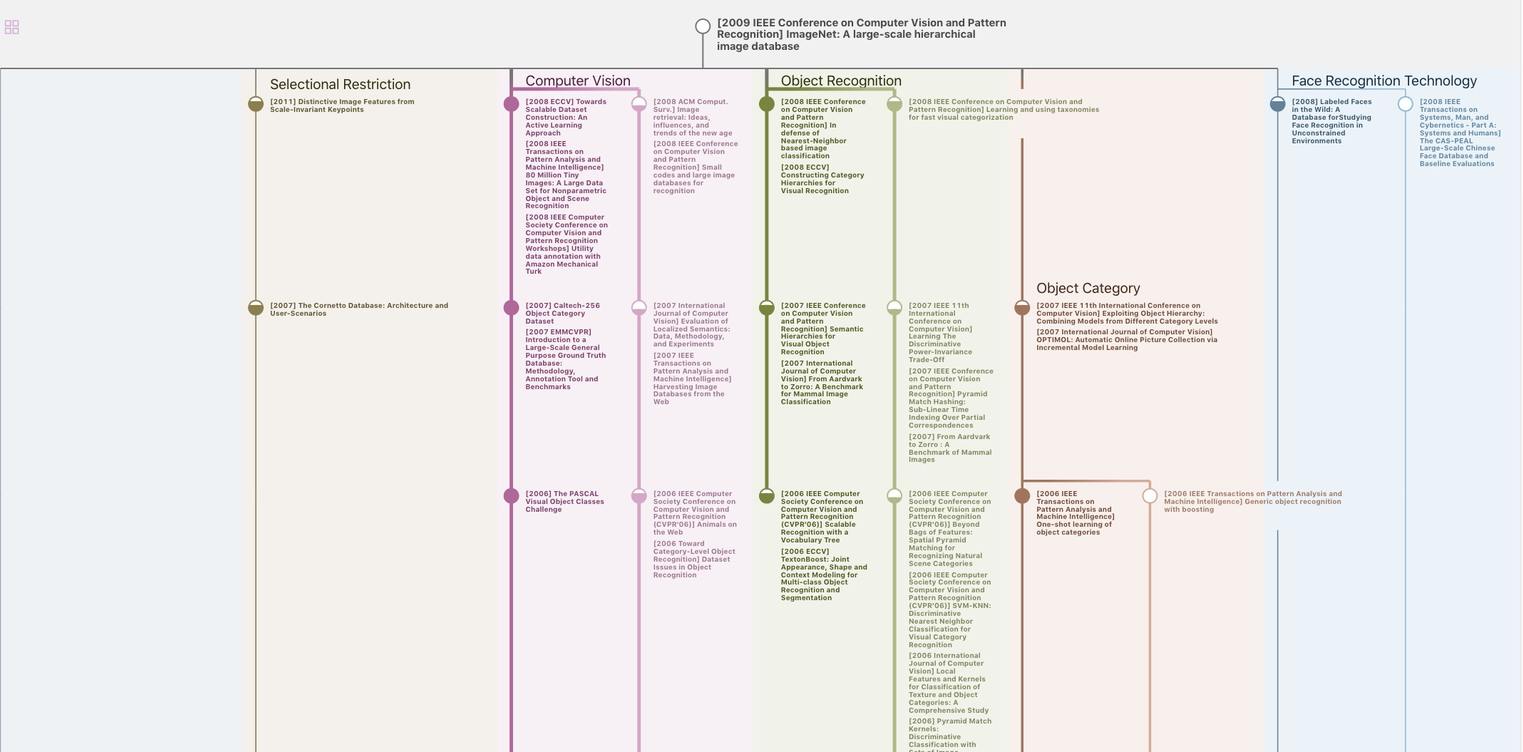
生成溯源树,研究论文发展脉络
Chat Paper
正在生成论文摘要