A Novel Temporal Multi-Gate Mixture-of-Experts Approach for Vehicle Trajectory and Driving Intention Prediction
IEEE Transactions on Intelligent Vehicles(2023)
摘要
Accurate Vehicle Trajectory Prediction is critical for automated vehicles and advanced driver assistance systems. Vehicle trajectory prediction consists of two essential tasks, i.e., longitudinal position prediction and lateral position prediction. There is a significant correlation between driving intentions and vehicle motion. In existing work, the three tasks are often conducted separately without considering the relationships between the longitudinal position, lateral position, and driving intention. In this paper, we propose a novel Temporal Multi-Gate Mixture-of-Experts (TMMOE) model for simultaneously predicting the vehicle trajectory and driving intention. The proposed model consists of three layers: a shared layer, an expert layer, and a fully connected layer. In the model, the shared layer utilizes Temporal Convolutional Networks (TCN) to extract temporal features. Then the expert layer is built to identify different information according to the three tasks. Moreover, the fully connected layer is used to integrate and export prediction results. To achieve better performance, uncertainty algorithm is used to construct the multi-task loss function. Finally, the publicly available CitySim dataset validates the TMMOE model, demonstrating superior performance compared to the LSTM model, achieving the highest classification and regression results. Keywords: Vehicle trajectory prediction, driving intentions Classification, Multi-task
更多查看译文
关键词
Multi-task learning,Vehicle trajectory prediction,Driving intentions classification,TMMOE model
AI 理解论文
溯源树
样例
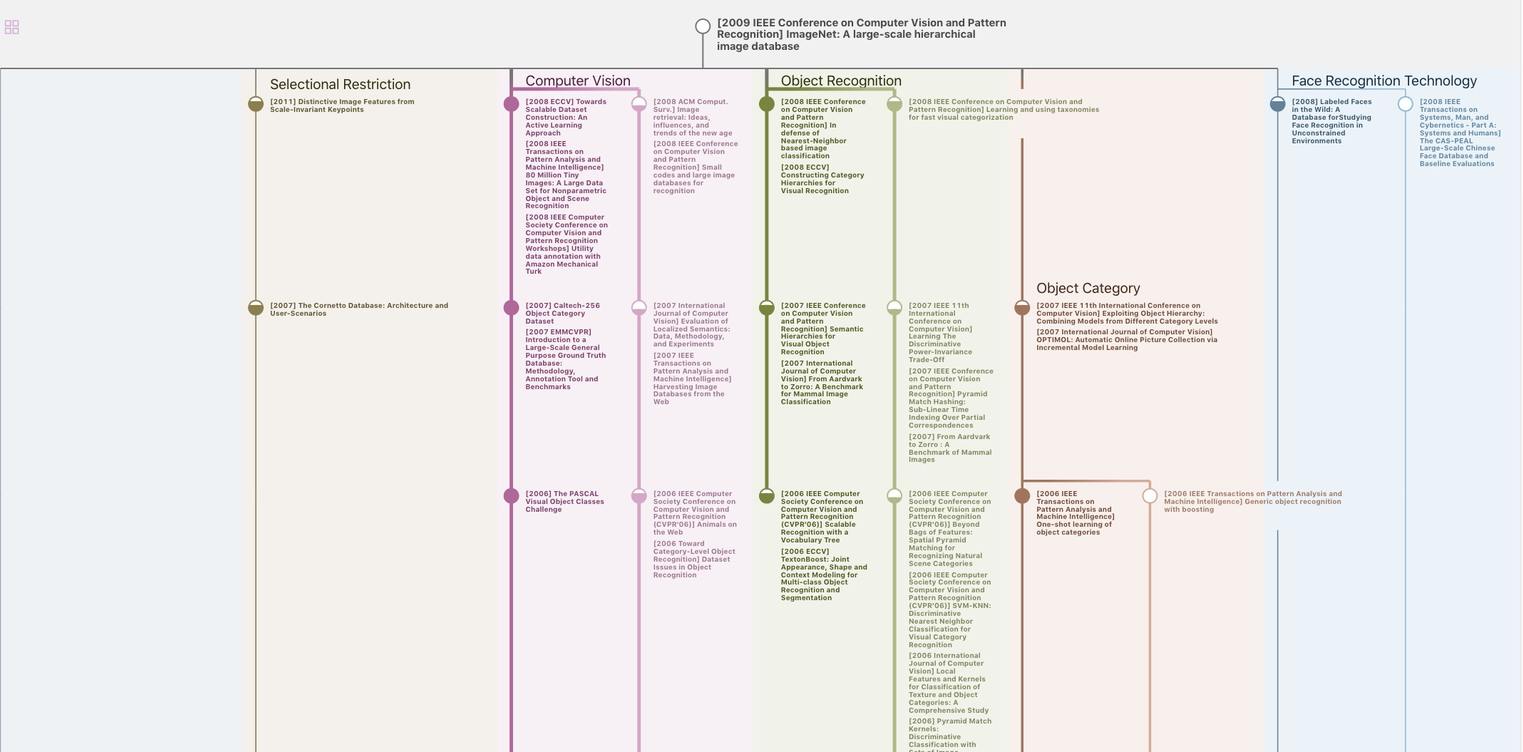
生成溯源树,研究论文发展脉络
Chat Paper
正在生成论文摘要