Implicit Neural Representation in Medical Imaging: A Comparative Survey
2023 IEEE/CVF INTERNATIONAL CONFERENCE ON COMPUTER VISION WORKSHOPS, ICCVW(2023)
摘要
Implicit neural representations (INRs) have gained prominence as a powerful paradigm in scene reconstruction and computer graphics, demonstrating remarkable results. By utilizing neural networks to parameterize data through implicit continuous functions, INRs offer several benefits. Recognizing the potential of INRs beyond these domains, this survey aims to provide a comprehensive overview of INR models in the field of medical imaging. In medical settings, numerous challenging and ill-posed problems exist, making INRs an attractive solution. The survey explores the application of INRs in various medical imaging tasks, such as image reconstruction, segmentation, registration, novel view synthesis, and compression. It discusses the advantages and limitations of INRs, highlighting their resolution-agnostic nature, memory efficiency, ability to avoid locality biases, and differentiability, enabling adaptation to different tasks. Furthermore, the survey addresses the challenges and considerations specific to medical imaging data, such as data availability, computational complexity, and dynamic clinical scene analysis. It also identifies future research directions and opportunities, including integration with multi-modal imaging, real-time and interactive systems, and domain adaptation for clinical decision support. To facilitate further exploration and implementation of INRs in medical image analysis, we have provided a compilation of cited studies along with their available open-source implementations on GitHub. Finally, we aim to consistently incorporate the most recent and relevant papers regularly.
更多查看译文
关键词
medical imaging,representation
AI 理解论文
溯源树
样例
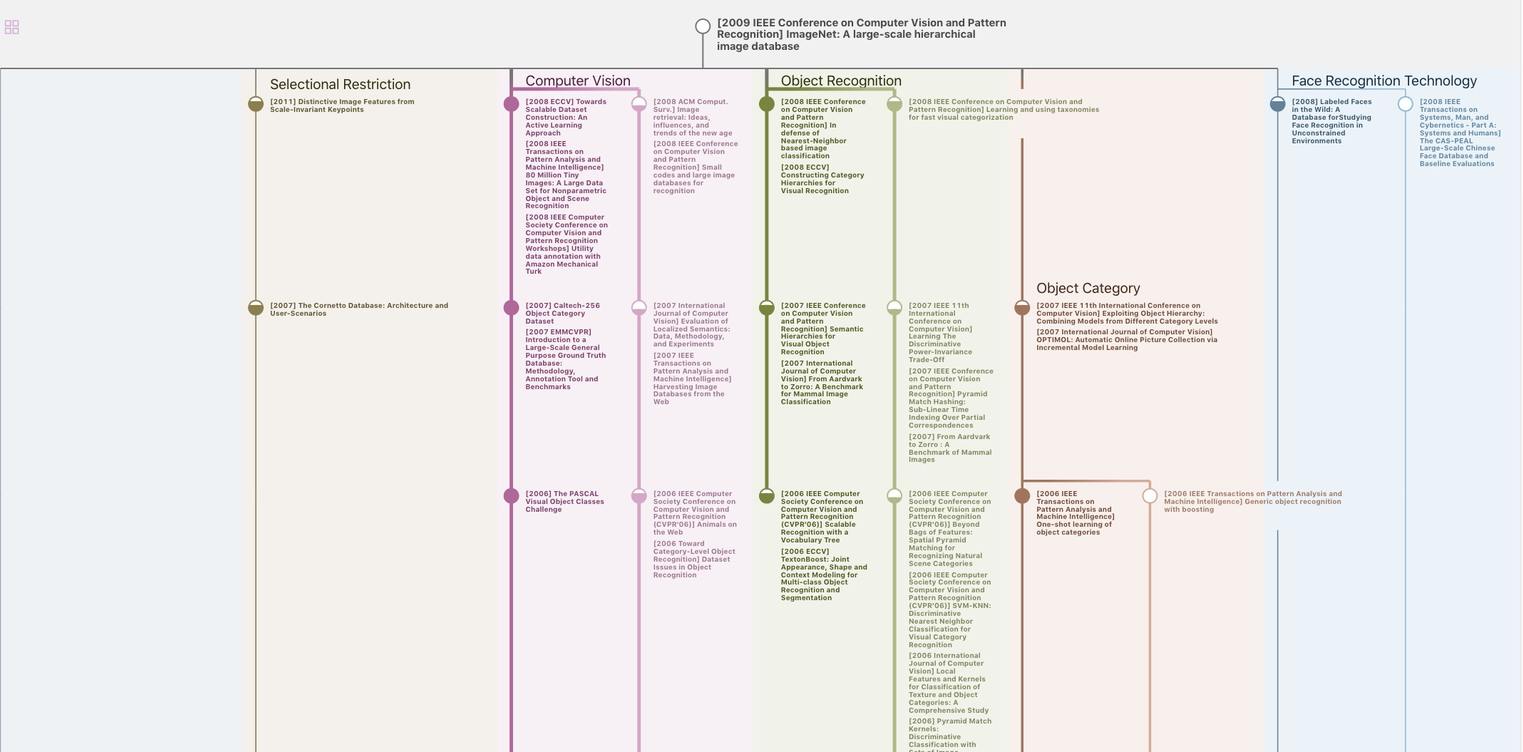
生成溯源树,研究论文发展脉络
Chat Paper
正在生成论文摘要