Distributed Traffic Engineering in Hybrid Software Defined Networks: A Multi-agent Reinforcement Learning Framework
CoRR(2023)
摘要
Traffic Engineering (TE) is an efficient technique to balance network flows and thus improves the performance of a hybrid Software Defined Network (SDN). Previous TE solutions mainly leverage heuristic algorithms to centrally optimize link weight setting or traffic splitting ratios under the static traffic demand. Note that as the network scale becomes larger and network management gains more complexity, it is notably that the centralized TE methods suffer from a high computation overhead and a long reaction time to optimize routing of flows when the network traffic demand dynamically fluctuates or network failures happen. To enable adaptive and efficient routing in TE, we propose a Multi-agent Reinforcement Learning method CMRL that divides the routing optimization of a large network into multiple small-scale routing decisionmaking problems. To coordinate the multiple agents for achieving a global optimization goal, we construct an interactive environment for training the routing agents that own partial link utilization observations. To optimize credit assignment of multi-agent, we introduce the difference reward assignment mechanism for encouraging agents to take better action. Extensive simulations conducted on the real traffic traces demonstrate the superiority of CMRL in improving TE performance, especially when traffic demands change or network failures happen.
更多查看译文
关键词
traffic engineering,reinforcement learning,hybrid software,networks,multi-agent
AI 理解论文
溯源树
样例
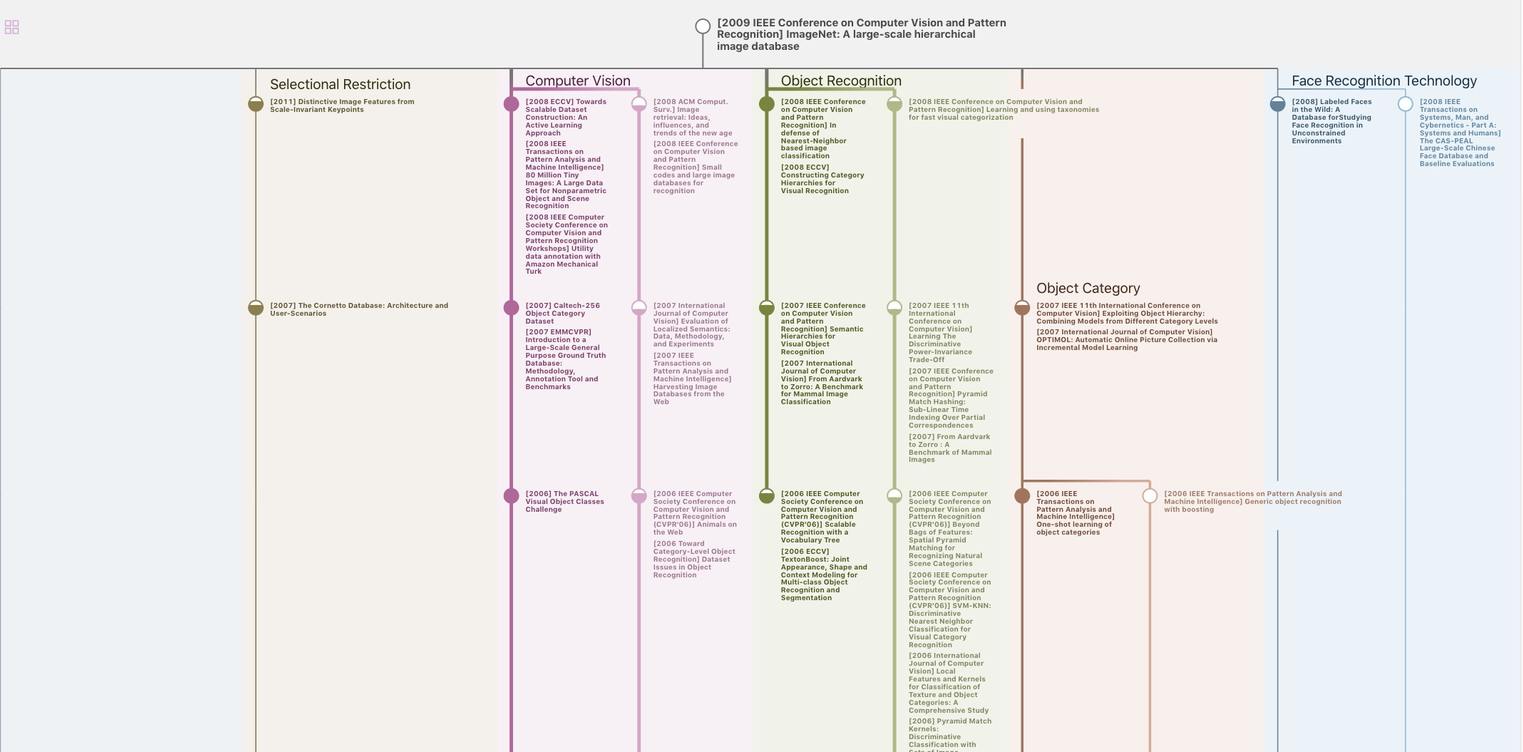
生成溯源树,研究论文发展脉络
Chat Paper
正在生成论文摘要