Multi-output Headed Ensembles for Product Item Classification
CoRR(2023)
摘要
In this paper, we revisit the problem of product item classification for large-scale e-commerce catalogs. The taxonomy of e-commerce catalogs consists of thousands of genres to which are assigned items that are uploaded by merchants on a continuous basis. The genre assignments by merchants are often wrong but treated as ground truth labels in automatically generated training sets, thus creating a feedback loop that leads to poorer model quality over time. This problem of taxonomy classification becomes highly pronounced due to the unavailability of sizable curated training sets. Under such a scenario it is common to combine multiple classifiers to combat poor generalization performance from a single classifier. We propose an extensible deep learning based classification model framework that benefits from the simplicity and robustness of averaging ensembles and fusion based classifiers. We are also able to use metadata features and low-level feature engineering to boost classification performance. We show these improvements against robust industry standard baseline models that employ hyperparameter optimization. Additionally, due to continuous insertion, deletion and updates to real-world high-volume e-commerce catalogs, assessing model performance for deployment using A/B testing and/or manual annotation becomes a bottleneck. To this end, we also propose a novel way to evaluate model performance using user sessions that provides better insights in addition to traditional measures of precision and recall.
更多查看译文
关键词
classification,multi-output
AI 理解论文
溯源树
样例
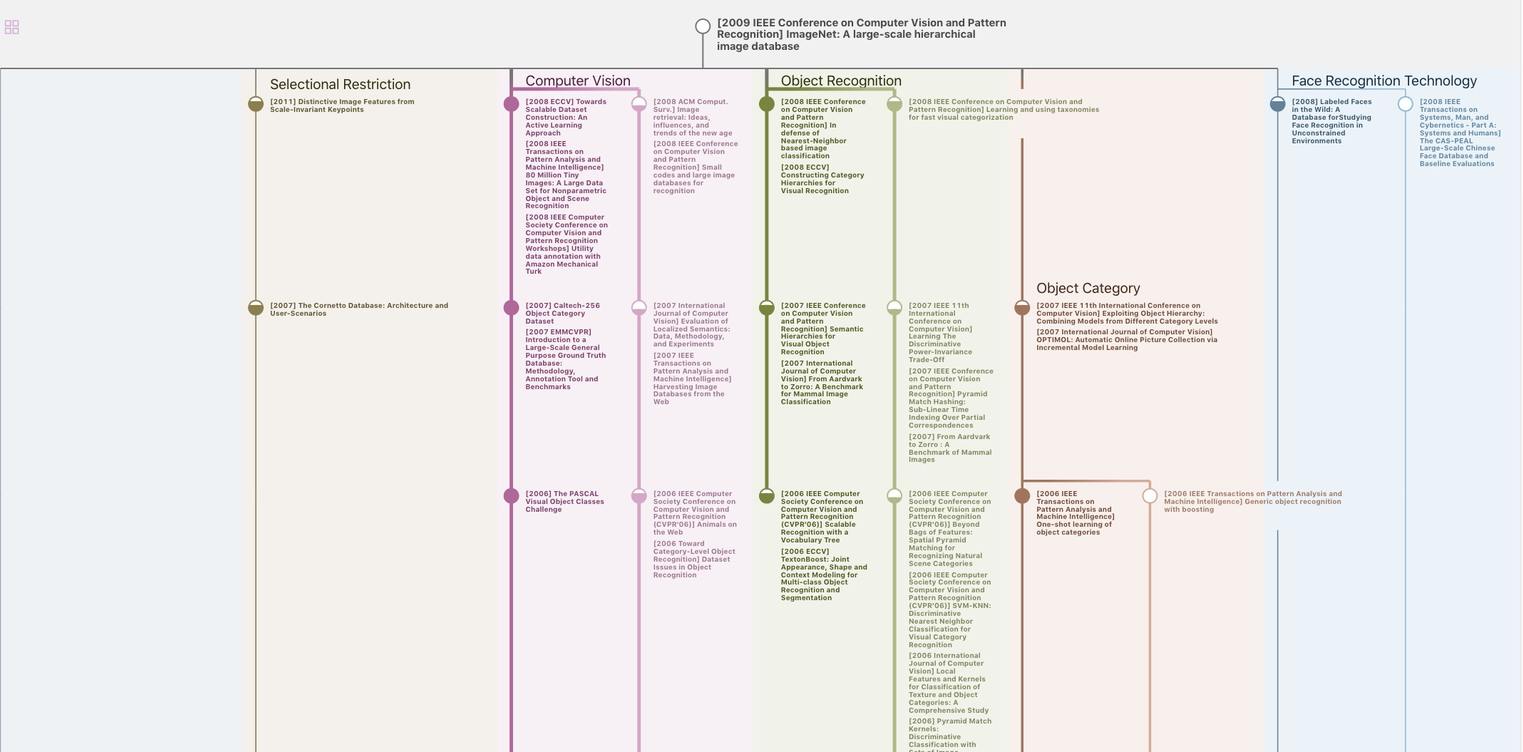
生成溯源树,研究论文发展脉络
Chat Paper
正在生成论文摘要