Primitive Skill-based Robot Learning from Human Evaluative Feedback
2023 IEEE/RSJ INTERNATIONAL CONFERENCE ON INTELLIGENT ROBOTS AND SYSTEMS (IROS)(2023)
Abstract
Reinforcement learning (RL) algorithms face significant challenges when dealing with long-horizon robot manipulation tasks in real-world environments due to sample inefficiency and safety issues. To overcome these challenges, we propose a novel framework, SEED, which leverages two approaches: reinforcement learning from human feedback (RLHF) and primitive skill-based reinforcement learning. Both approaches are particularly effective in addressing sparse reward issues and the complexities involved in long-horizon tasks. By combining them, SEED reduces the human effort required in RLHF and increases safety in training robot manipulation with RL in real-world settings. Additionally, parameterized skills provide a clear view of the agent's high-level intentions, allowing humans to evaluate skill choices before they are executed. This feature makes the training process even safer and more efficient. To evaluate the performance of SEED, we conducted extensive experiments on five manipulation tasks with varying levels of complexity. Our results show that SEED significantly outperforms state-of-the-art RL algorithms in sample efficiency and safety. In addition, SEED also exhibits a substantial reduction of human effort compared to other RLHF methods. Further details and video results can be found at https: //seediros23.github.io/.
MoreTranslated text
Key words
robot learning,skill-based
AI Read Science
Must-Reading Tree
Example
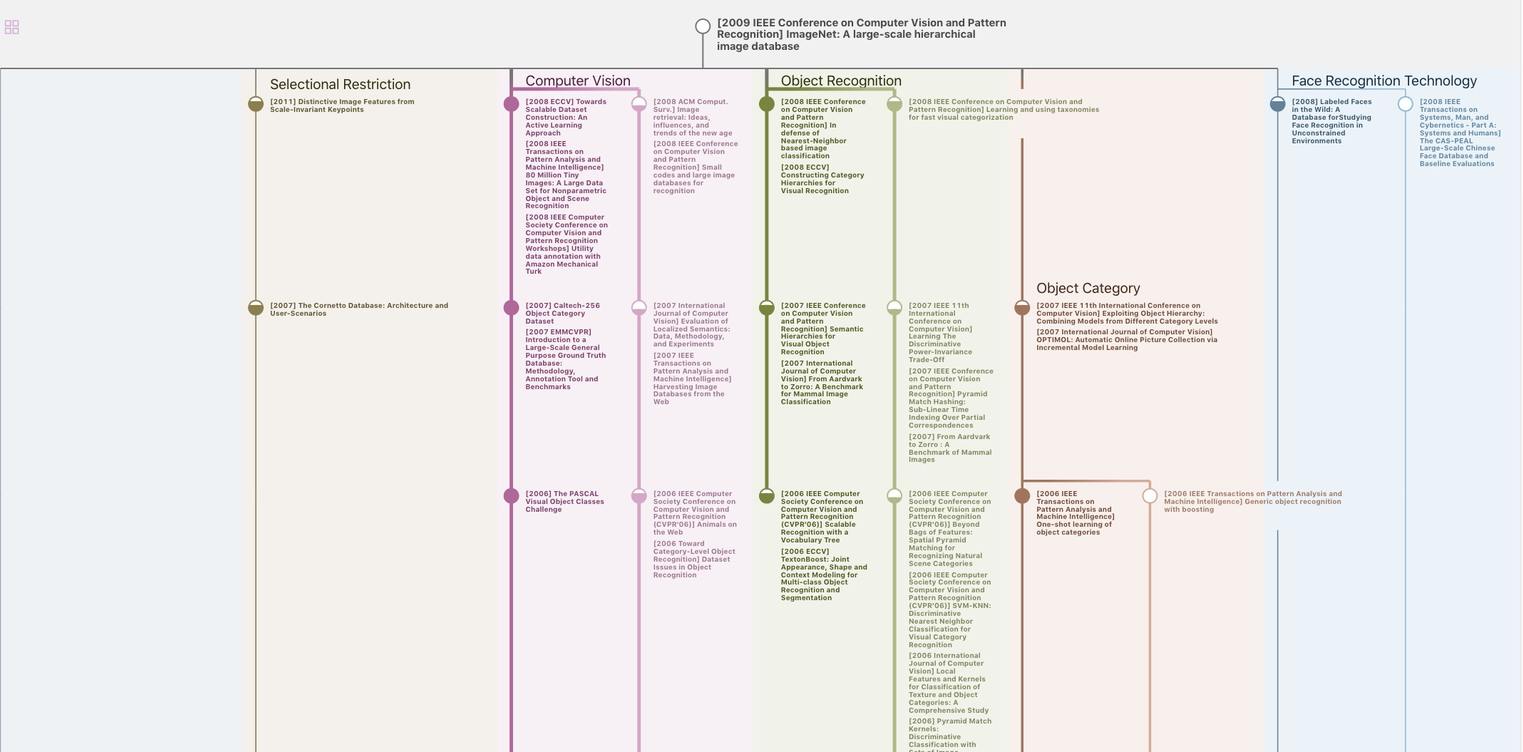
Generate MRT to find the research sequence of this paper
Chat Paper
Summary is being generated by the instructions you defined