ST-Segment Anomalies Detection from Compressed Sensing Based ECG Data by Means of Machine Learning.
BIOSTEC (Selected Papers)(2022)
摘要
Telemedicine allows to constantly monitor patients without the need of hospitalization. Such a practice is enabled by IoMT (Internet of Medical Things) devices, which acquire signals, and by AI (Artificial Intelligence)-based algorithms, able to automatize the analysis carried out on many patients. The large quantity of data produced every minute by IoMT devices, however, makes the use of compression fundamental to reduce the bandwith used to transmit those data and the memory required to acquire them, and lossy compression (specifically, Compressed Sensing) has shown to be the most effective technique to use for the task. Previous work introduced AI-based approaches for automatically detecting hearth-related anomalies based on the electrocardiographic (ECG) signal. However, most of them assume the presence of the complete raw ECG signal. In this paper, we extend our previous work in which we introduced RAST, an approach for detecting ST segment-related anomalies. We present RAST $$^C$$ , an approach able at identifying the same abnormalities but on a highly compressed ECG signal. The results of our experiment, carried out on the Physionet European ST-T Database, shows that RAST $$^C$$ is capable of discriminating Normal ECG from ST-depression and ST-elevation with classification metrics around 90%, even with the highest compression ratio experimented, i.e., with an ECG signal compressed by a factor of 16.
更多查看译文
关键词
ecg data,compressed sensing,anomalies,st-segment
AI 理解论文
溯源树
样例
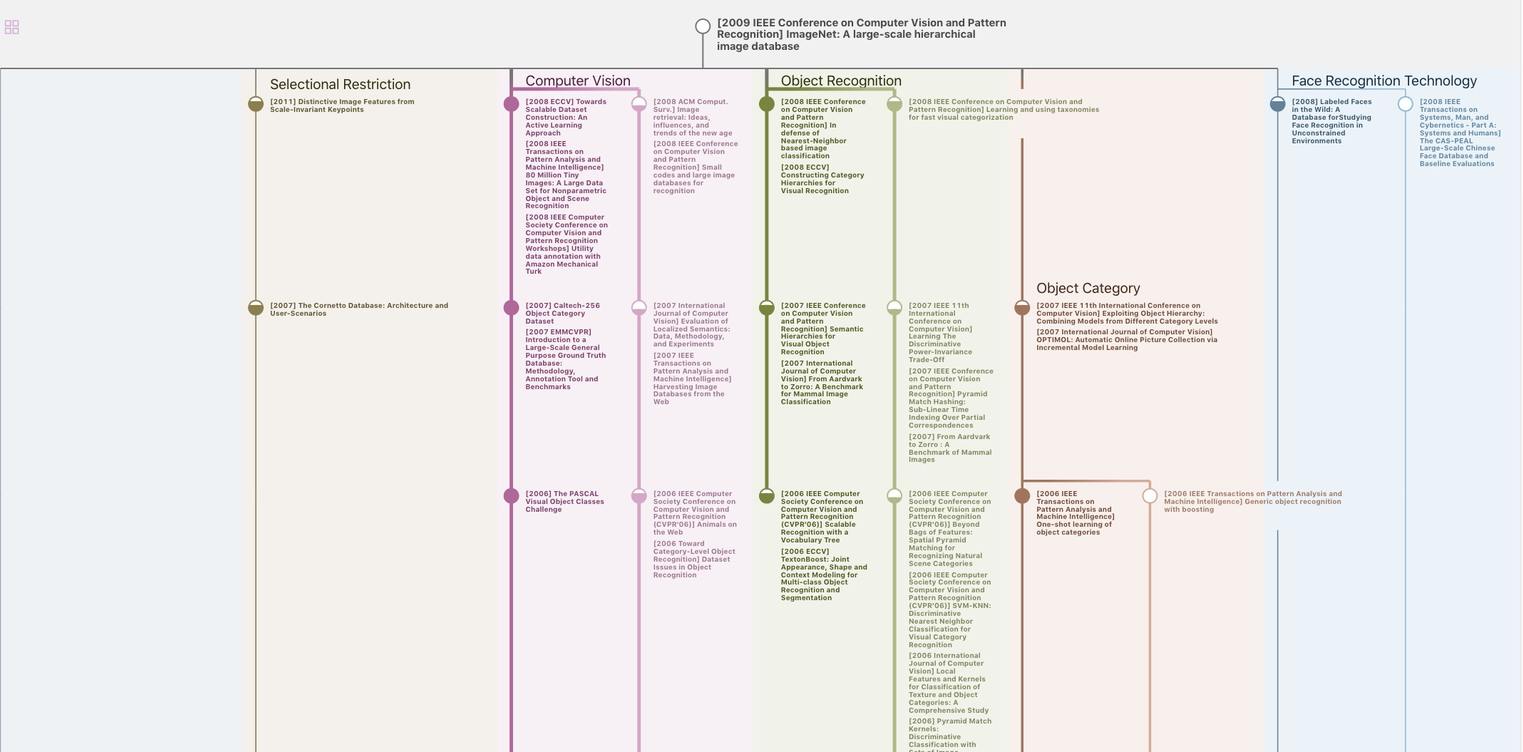
生成溯源树,研究论文发展脉络
Chat Paper
正在生成论文摘要