DeepThermo: Deep Learning Accelerated Parallel Monte Carlo Sampling for Thermodynamics Evaluation of High Entropy Alloys.
IPDPS(2023)
摘要
Since the introduction of Metropolis Monte Carlo (MC) sampling, it and its variants have become standard tools used for thermodynamics evaluations of physical systems. However, a long-standing problem that hinders the effectiveness and efficiency of MC sampling is the lack of a generic method (a.k.a. MC proposal) to update the system configurations. Consequently, current practices are not scalable. Here we propose a parallel MC sampling framework for thermodynamics evaluation-DeepThermo. By using deep learning-based MC proposals that can globally update the system configurations, we show that DeepThermo can effectively evaluate the phase transition behaviors of high entropy alloys, which have an astronomical configuration space. For the first time, we directly evaluate a density of states expanding over a range of similar to e(10,000) for a real material. We also demonstrate DeepThermo's performance and scalability up to 3,000 GPUs on both NVIDIA V100 and AMD MI250X-based supercomputers.
更多查看译文
关键词
Monte Carlo Method, Deep Learning, High Entropy Alloy
AI 理解论文
溯源树
样例
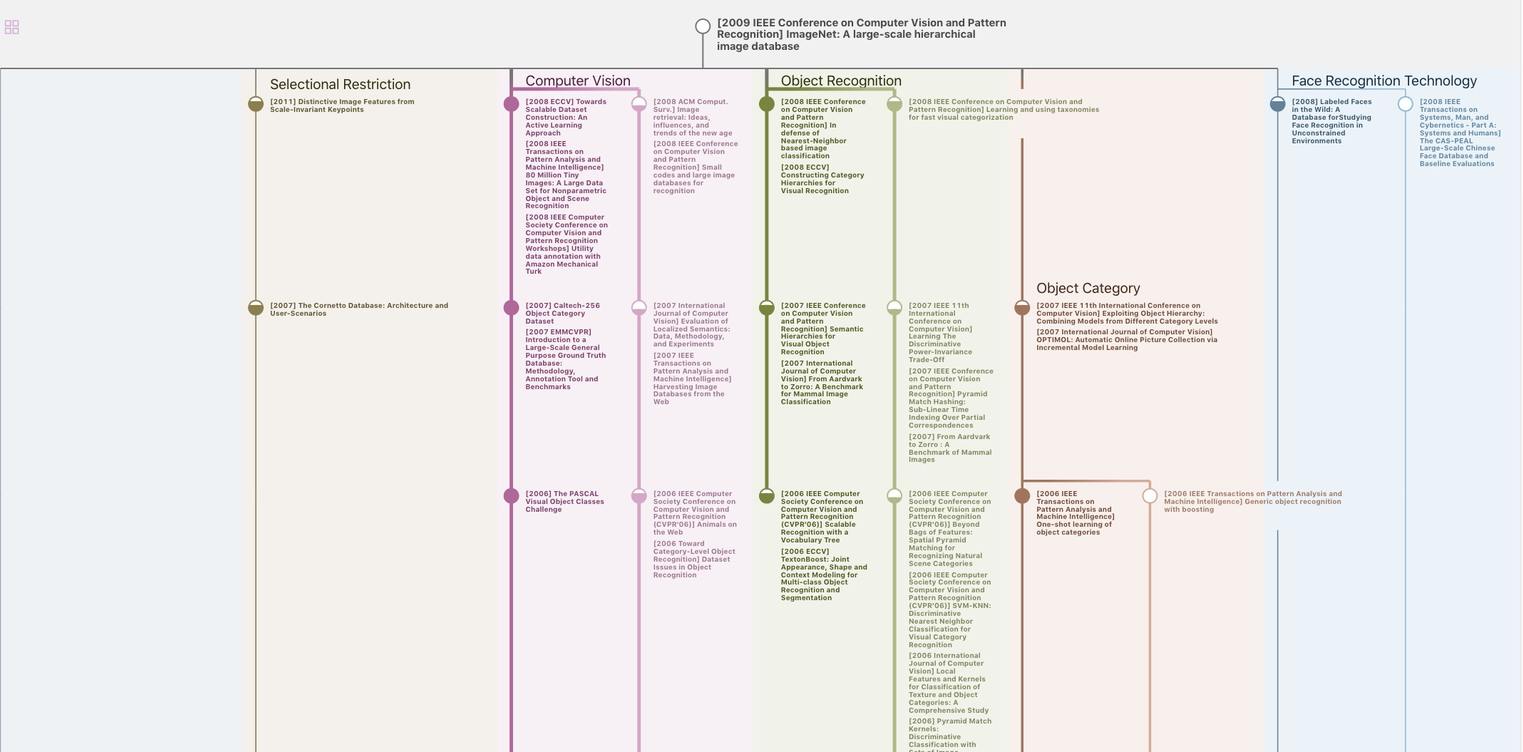
生成溯源树,研究论文发展脉络
Chat Paper
正在生成论文摘要