GPU-Accelerated Error-Bounded Compression Framework for Quantum Circuit Simulations.
IPDPS(2023)
摘要
Quantum circuit simulations enable researchers to develop quantum algorithms without the need for a physical quantum computer. Quantum computing simulators, however, all suffer from significant memory footprint requirements, which prevents large circuits from being simulated on classical supercomputers. In this paper, we explore different lossy compression strategies to substantially shrink quantum circuit tensors in the QTensor package (a state-of-the-art tensor network quantum circuit simulator) while ensuring the reconstructed data satisfy the user-needed fidelity. Our contribution is fourfold. (1) We propose a series of optimized pre- and post-processing steps to boost the compression ratio of tensors with a very limited performance overhead. (2) We characterize the impact of lossy decompressed data on quantum circuit simulation results, and leverage the analysis to ensure the fidelity of reconstructed data. (3) We propose a configurable compression framework for GPU based on cuSZ and cuSZx, two state-of-the-art GPU-accelerated lossy compressors, to address different use-cases: either prioritizing compression ratios or prioritizing compression speed. (4) We perform a comprehensive evaluation by running 9 state-of-the-art compressors on an NVIDIA A100 GPU based on QTensor-generated tensors of varying sizes. When prioritizing compression ratio, our results show that our strategies can increase the compression ratio nearly 10 times compared to using only cuSZ. When prioritizing throughput, we can perform compression at the comparable speed as cuSZx while achieving 3-4x higher compression ratios. Decompressed tensors can be used in QTensor circuit simulation to yield a final energy result within 1-5% of the true energy value.
更多查看译文
关键词
compression, quantum computing, GPU
AI 理解论文
溯源树
样例
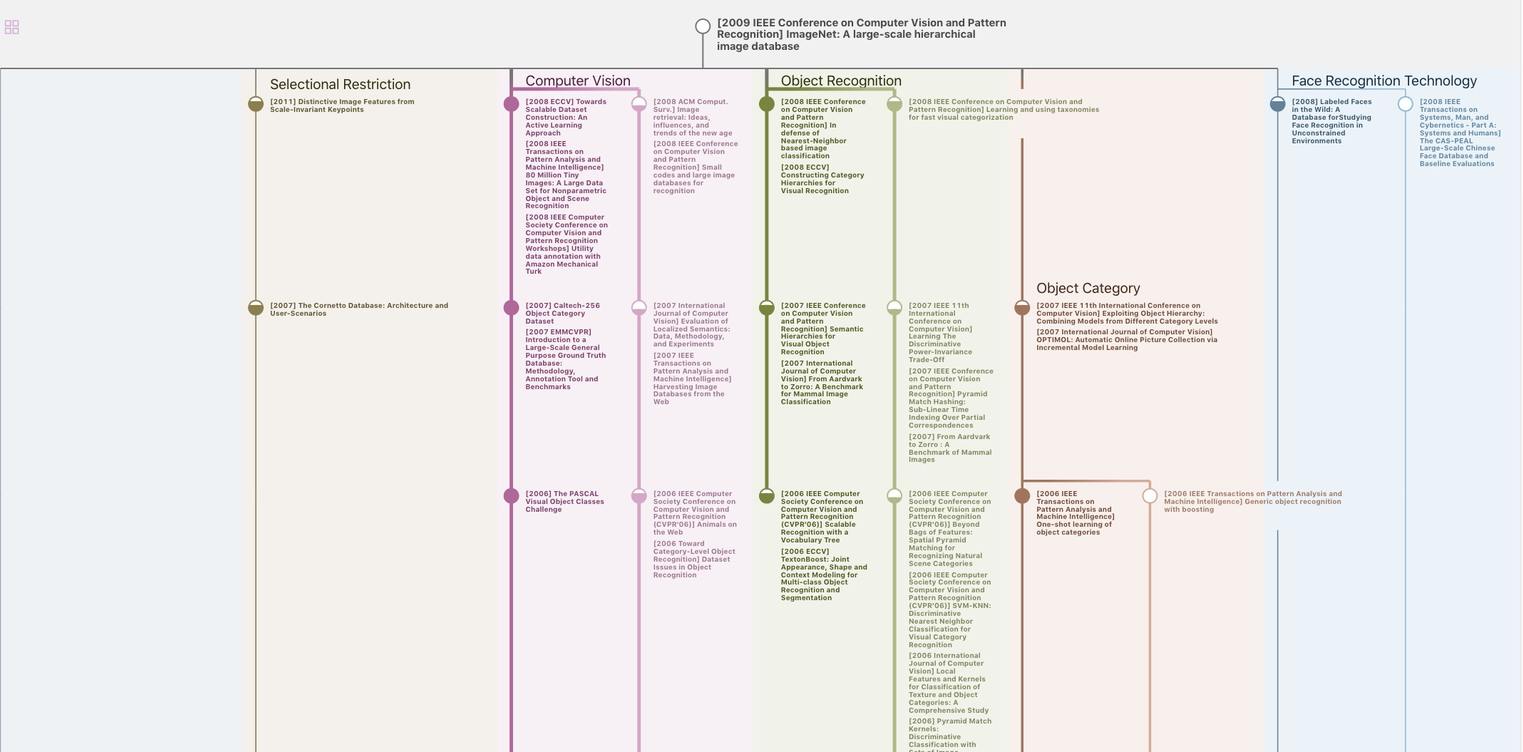
生成溯源树,研究论文发展脉络
Chat Paper
正在生成论文摘要