A Clustering Strategy-Based Evolutionary Algorithm for Feature Selection in Classification.
IEA/AIE (1)(2023)
摘要
Feature selection is a technique used in data pre-processing to select the most relevant subset of features from a larger set, with the goal of improving classification performance. Evolutionary algorithms have been commonly proposed to solve feature selection problems, but they can suffer from issues originated from diversity reduction and crowding distance decrease, which can lead to suboptimal results. In this study, we propose a new evolutionary algorithm called clustering strategy based evolutionary algorithm (CEA) for feature selection in classification. CEA combines the clustering mechanism to gather individuals into different clusters, and the crossover operation is dominated by the parents in different clusters, thus enhancing the exploration ability of the algorithm and avoiding the population falling into the local optimal solution space. The performance of CEA was evaluated on 13 classification datasets and compared to four mainstream evolutionary algorithms. The experimental results showed that CEA was able to achieve better classification performance using similar or fewer features than the other algorithms.
更多查看译文
关键词
feature selection,evolutionary algorithm,clustering,classification,strategy-based
AI 理解论文
溯源树
样例
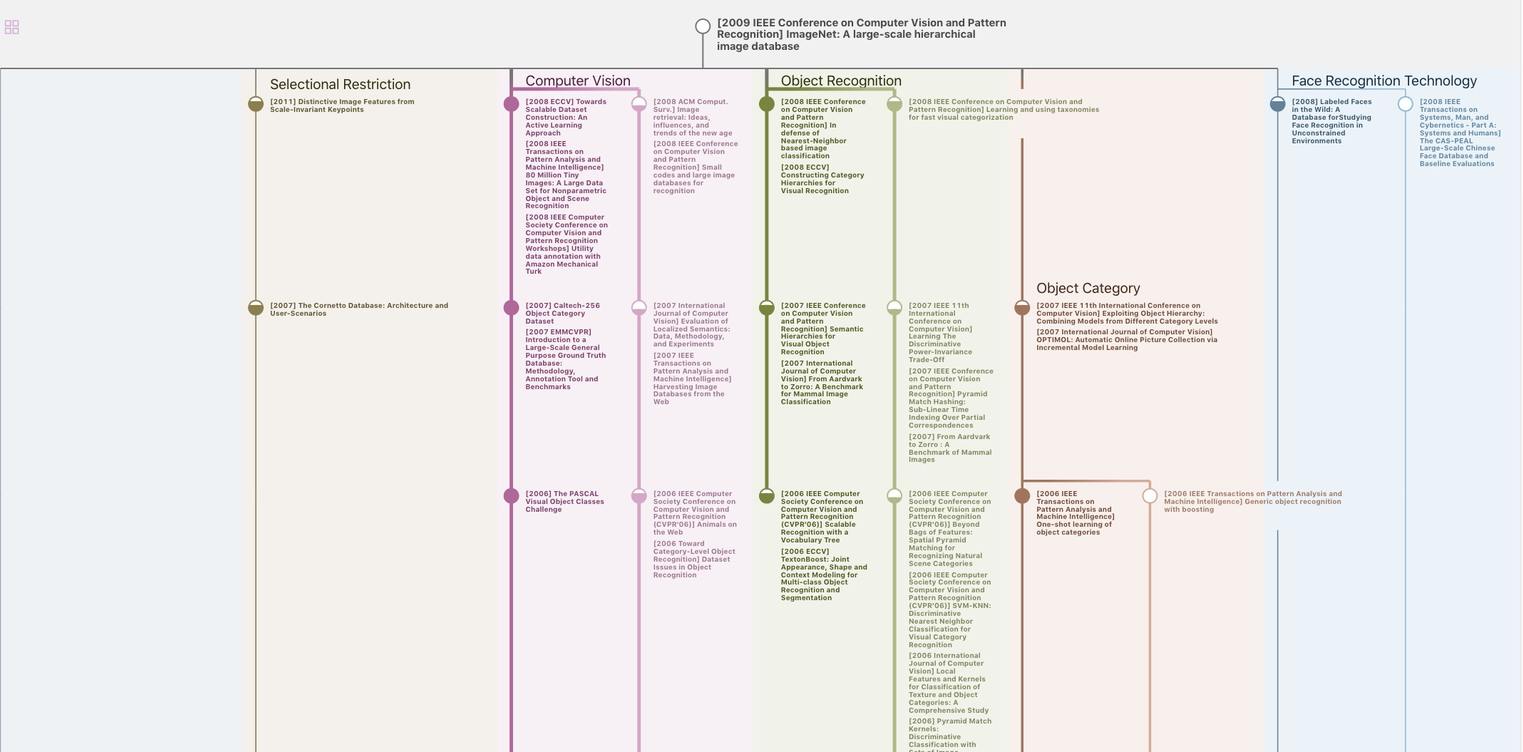
生成溯源树,研究论文发展脉络
Chat Paper
正在生成论文摘要