DRL-based Service Migration for MEC Cloud-Native 5G and beyond Networks.
NetSoft(2023)
摘要
Multi-access Edge Computing (MEC) has been considered one of the most prominent enablers for low-latency access to services provided over the telecommunications network. Nevertheless, client mobility, as well as external factors which impact the communication channel can severely deteriorate the eventual user-perceived latency times. Such processes can be averted by migrating the provided services to other edges, while the end-user changes their base station association as they move within the serviced region. In this work, we start from an entirely virtualized cloud-native 5G network based on the OpenAirInterface platform and develop our architecture for providing seamless live migration of edge services. On top of this infrastructure, we employ a Deep Reinforcement Learning (DRL) approach that is able to proactively relocate services to new edges, subject to the user's multi-cell latency measurements and the workload status of the servers. We evaluate our scheme in a testbed setup by emulating mobility using realistic mobility patterns and workloads from real-world clusters. Our results denote that our scheme is capable sustain low-latency values for the end users, based on their mobility within the serviced region.
更多查看译文
关键词
Multi-access Edge Computing,Beyond 5G,Cloud-Native network,AI/ML,OpenAirInterface,Kubernetes
AI 理解论文
溯源树
样例
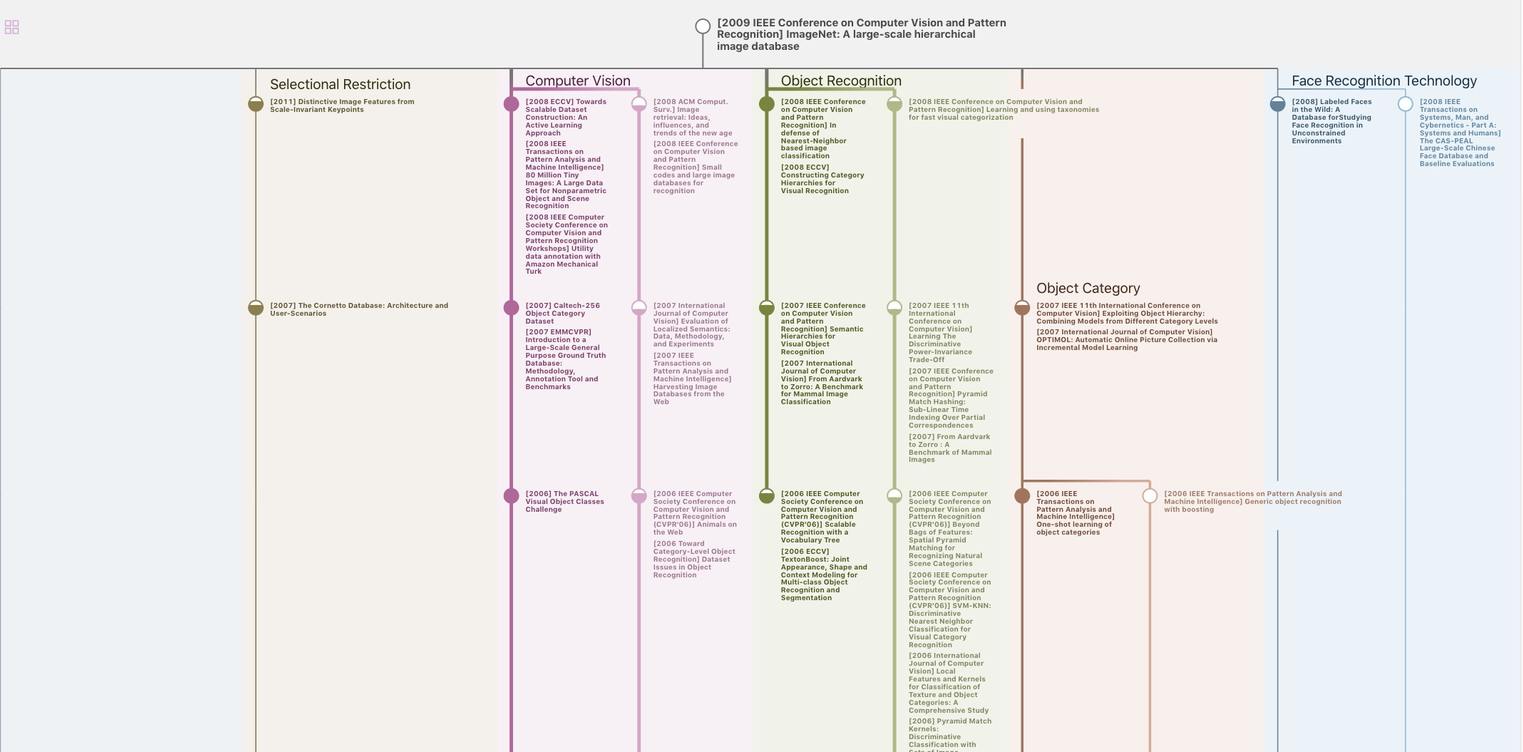
生成溯源树,研究论文发展脉络
Chat Paper
正在生成论文摘要