Robust Seeded Image Segmentation Using Adaptive Label Propagation and Deep Learning-Based Contour Orientation.
ICCSA (2)(2023)
摘要
Deep Learning has become a popular tool for addressing complex tasks in many computer vision applications. Label diffusion methods have also been a very effective technique for getting accurate segmentations of real-world images, as they combine user autonomy, versatility and accurateness through a user-friendly interface. In this paper, we propose a seeded segmentation framework for partitioning real-world images by combining deep contour learning and graph-based label propagation models. More precisely, our approach takes a CNN-type contour detection network to learn graph edge weights, which are used as input to solve a coupled energy minimization problem that diffuses the user-selected annotations to the desired targets. To accurately extract deep features from image contours while generating diffusion maps, we train a deep learning architecture that integrates a hierarchical neural network, a graph-based label propagation model and a loss function, allowing the coupled training mechanism to refine the results until convergence. We attest to the effectiveness and accuracy of the proposed approach by conducting both quantitative and qualitative assessments with existing seeded image segmentation methods.
更多查看译文
关键词
robust seeded image segmentation,adaptive label propagation,learning-based
AI 理解论文
溯源树
样例
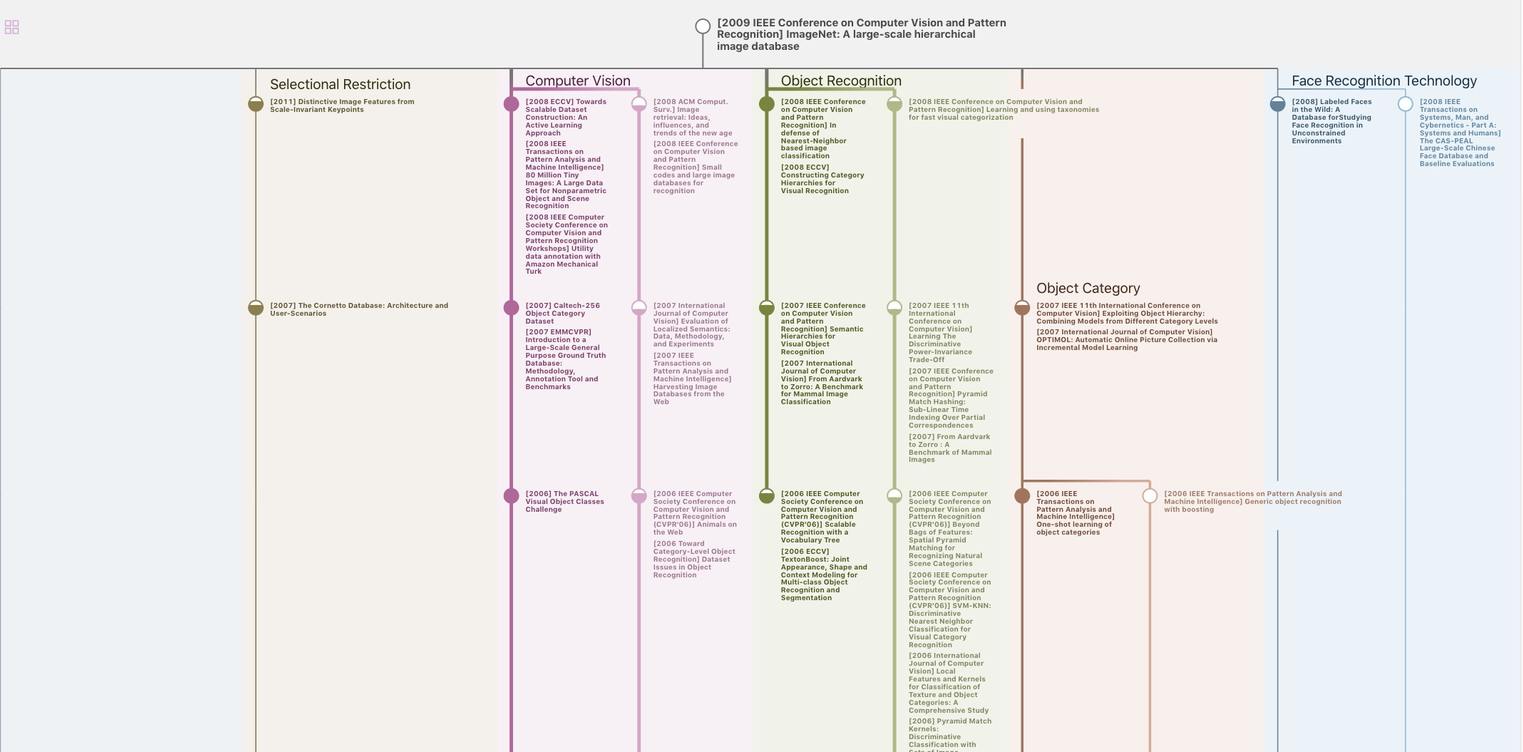
生成溯源树,研究论文发展脉络
Chat Paper
正在生成论文摘要