Principal Components Analysis Based Imputation for Logistic Regression.
IEA/AIE (1)(2023)
摘要
The field of AI and machine learning is constantly evolving, and as the size of data continues to grow, so does the need for accurate and efficient methods of data processing. However, the data is not always perfect, and missing data is becoming common and occurs more frequently. Therefore, imputation techniques, aside from precision, needed to be scalable. For that reason, we examine the performance of Principle Components Analysis Imputation (PCAI) [ 9 ], an imputation speeding up framework, for logistic regression. Note that the coefficients of a logistic regression model are usually used for interpretation. Therefore, in addition to examining the improvement in the speed of PCAI, we examine how the coefficients of fitted logistic regression models change when using this imputation speeding-up mechanism. To demonstrate the efficiency of the mentioned method, the model’s performance is compared against frequently used imputation methods on three popular datasets: Fashion MNIST, Gene, and Parkinson. And achieves lower time and better accuracy in most experiments.
更多查看译文
AI 理解论文
溯源树
样例
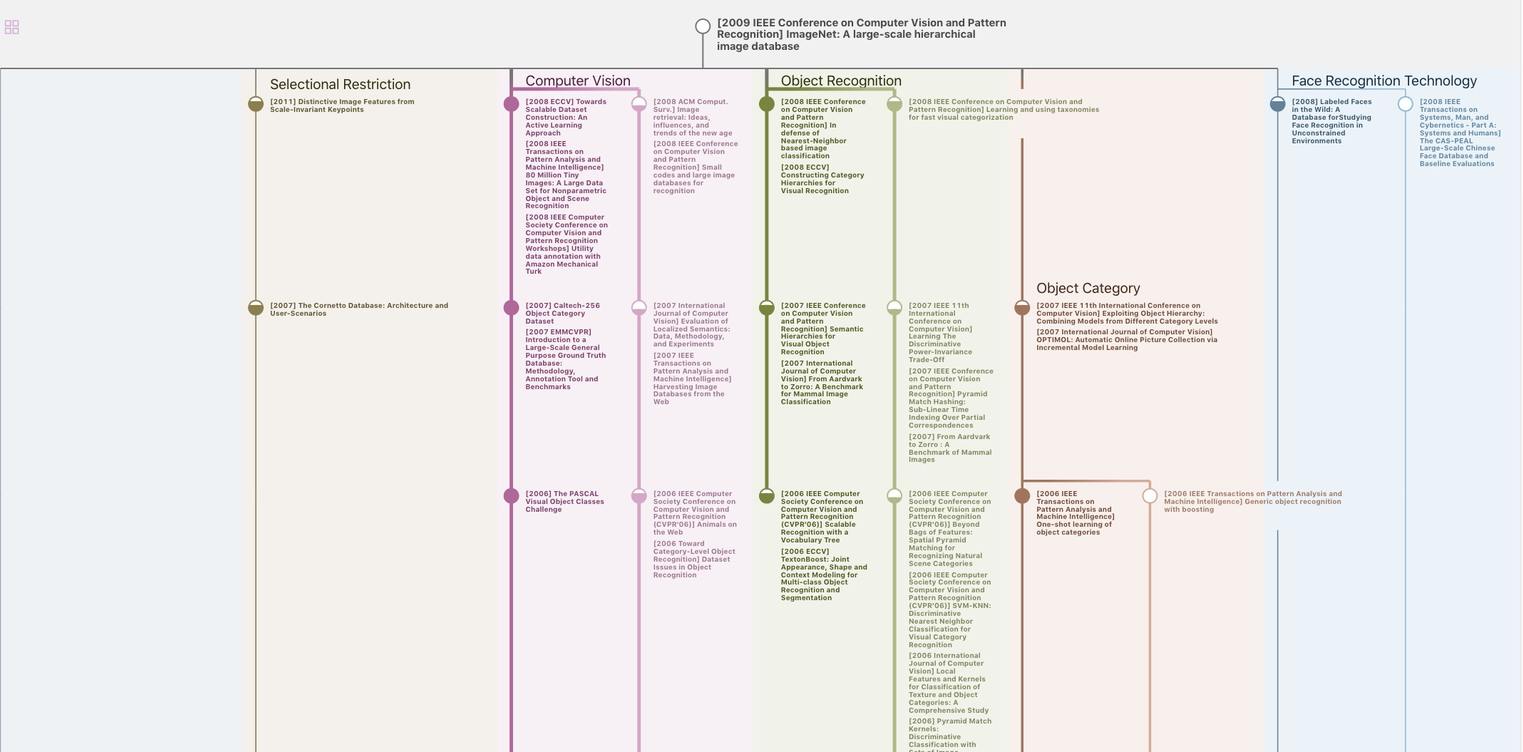
生成溯源树,研究论文发展脉络
Chat Paper
正在生成论文摘要