Time Series Forecasting Model Based on Domain Adaptation and Shared Attention.
IEA/AIE (2)(2023)
摘要
Time series forecasting is an essential problem involving many fields. Recently, with the development of big data technology, deep learning methods have been widely studied and achieved promising performance in time series forecasting tasks. But there is a limited number of time series or observations per time series. In this case, a time series forecasting model, which is based on domain adaptation and shared attention (DA-SA), is proposed in this study. First, we employ Transformer architecture as the basic framework of our model. Then, we specially design a selectively shared attention module to transfer valuable information from the data-rich domain to the data-poor domain by inducing domain-invariant latent features (queries and keys) and retraining domain-specific features (values). Besides, convolutional neural network is introduced to incorporate local context into the self-attention mechanism and captures the short-term dependencies of data. Finally, adversarial training is utilized to enhance the robustness of the model and improve prediction accuracy. The practicality and effectiveness of DA-SA for time series forecasting are verified on real-world datasets.
更多查看译文
关键词
domain adaptation,forecasting,time series,attention
AI 理解论文
溯源树
样例
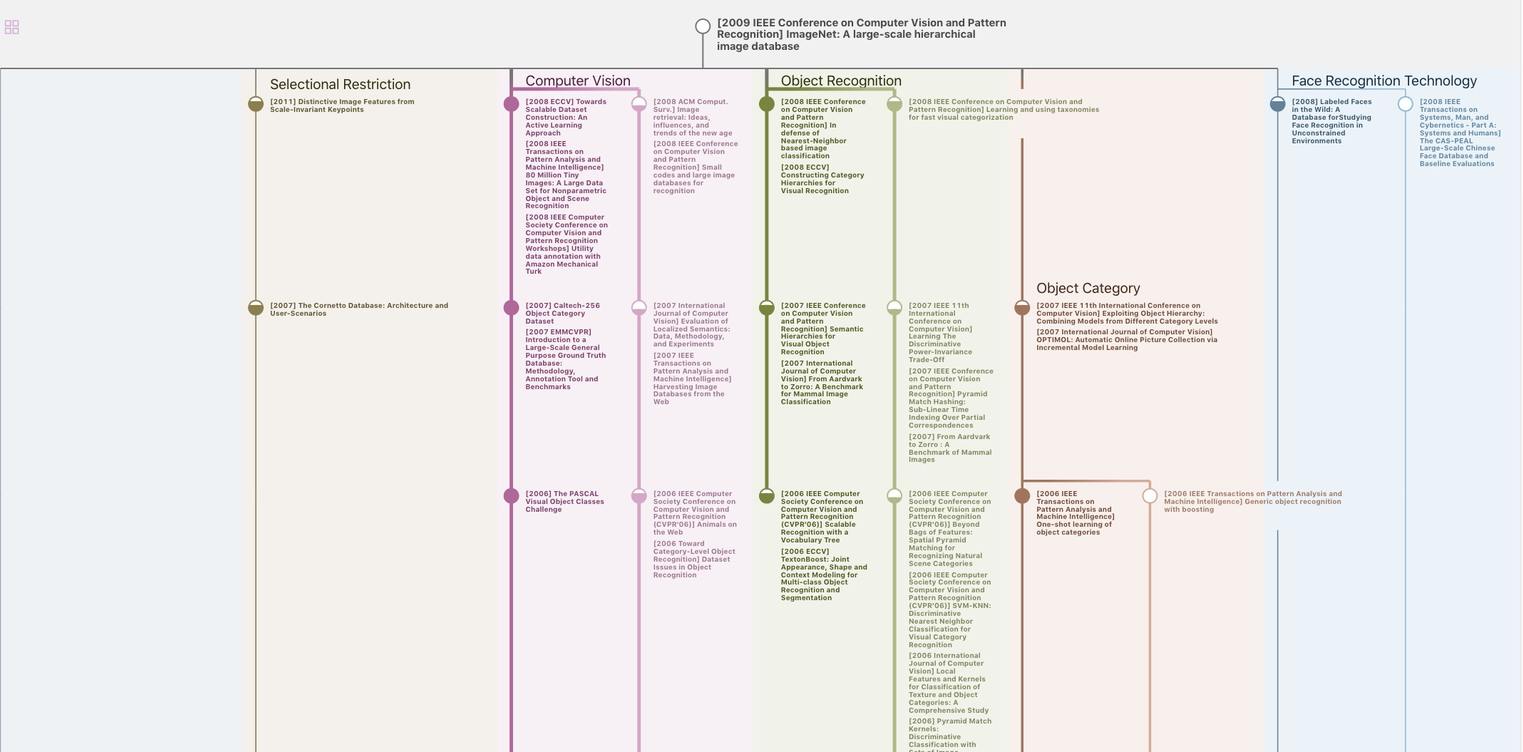
生成溯源树,研究论文发展脉络
Chat Paper
正在生成论文摘要