Evaluating Classifiers Trained on Differentially Private Synthetic Health Data
2023 IEEE 36TH INTERNATIONAL SYMPOSIUM ON COMPUTER-BASED MEDICAL SYSTEMS, CBMS(2023)
摘要
The release of differentially private (DP) synthetic data has been proposed as a solution to sharing sensitive individual-level medical data for statistical analysis and machine learning model development. The approach holds promise to generate realistic data that preserves many of the statistical properties of the original data while giving privacy guarantees that bound the risk of leaking any sensitive information about the individuals in the data. However, evaluating the generalization of machine learning models trained on DP-synthetic data remains an open question. A model selected based on its accuracy on synthetic data does not necessarily generalize well to real-world data, leading to poor results and incorrect insights. In this study, we experimentally compare two different protocols for model evaluation and hyperparameter selection for classifiers trained on DP-synthetic medical data. In the first protocol, we use only synthetic data for model selection and final evaluation of selected model, whereas in the second one, we assume limited DP access to a private real validation and test set held by the data curator. Our results provide novel insights into the practical feasibility and utility of different evaluation protocols for classifiers trained on DP synthetic data based on a comprehensive empirical study.
更多查看译文
关键词
Differential privacy,Model validation,Classification,Health data,Synthetic data
AI 理解论文
溯源树
样例
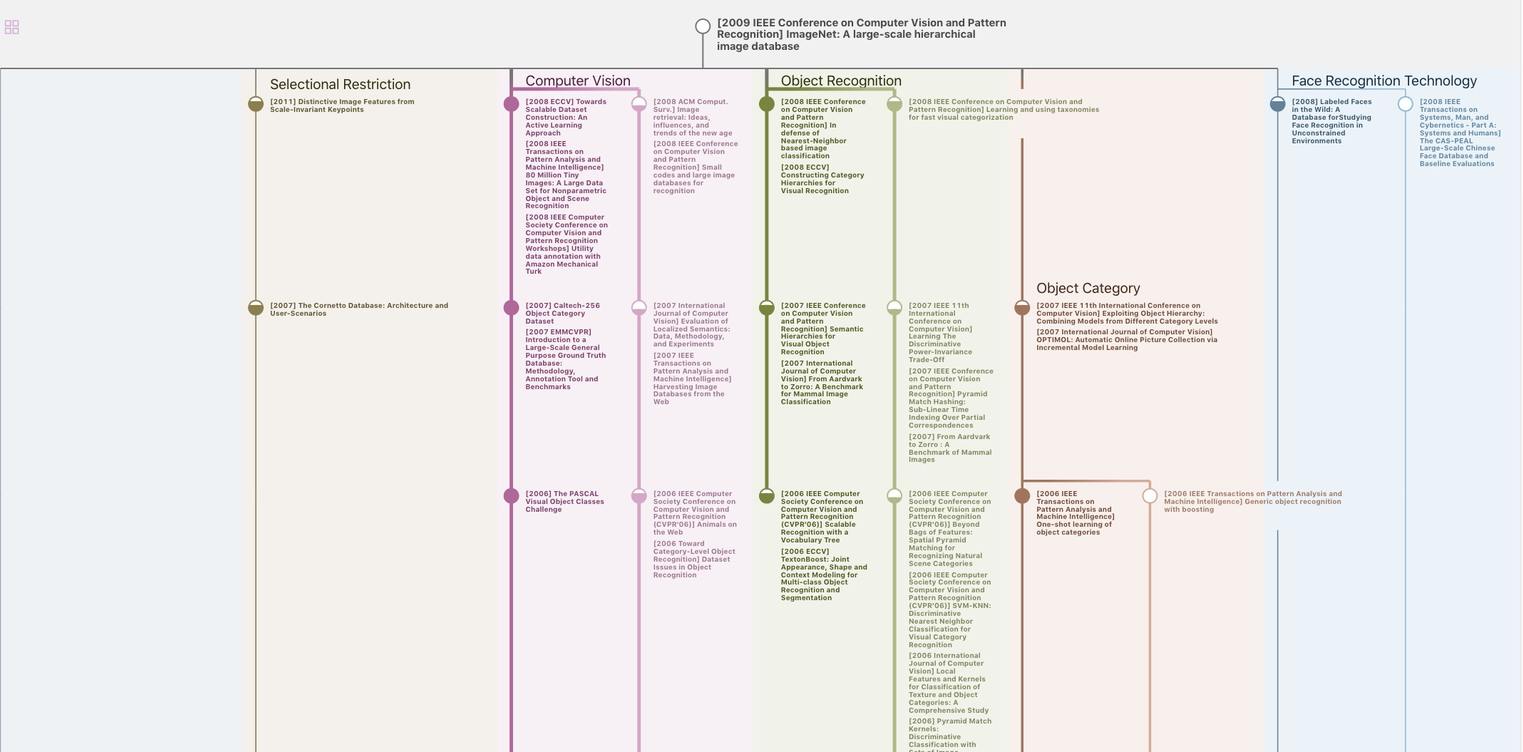
生成溯源树,研究论文发展脉络
Chat Paper
正在生成论文摘要